SSMBA: Self-Supervised Manifold Based Data Augmentation for Improving Out-of-Domain Robustness
Nathan Ng, Kyunghyun Cho, Marzyeh Ghassemi
Machine Learning for NLP Long Paper
You can open the pre-recorded video in a separate window.
Abstract:
Models that perform well on a training domain often fail to generalize to out-of-domain (OOD) examples. Data augmentation is a common method used to prevent overfitting and improve OOD generalization. However, in natural language, it is difficult to generate new examples that stay on the underlying data manifold. We introduce SSMBA, a data augmentation method for generating synthetic training examples by using a pair of corruption and reconstruction functions to move randomly on a data manifold. We investigate the use of SSMBA in the natural language domain, leveraging the manifold assumption to reconstruct corrupted text with masked language models. In experiments on robustness benchmarks across 3 tasks and 9 datasets, SSMBA consistently outperforms existing data augmentation methods and baseline models on both in-domain and OOD data, achieving gains of 0.8% on OOD Amazon reviews, 1.8% accuracy on OOD MNLI, and 1.4 BLEU on in-domain IWSLT14 German-English.
NOTE: Video may display a random order of authors.
Correct author list is at the top of this page.
Connected Papers in EMNLP2020
Similar Papers
Local Additivity Based Data Augmentation for Semi-supervised NER
Jiaao Chen, Zhenghui Wang, Ran Tian, Zichao Yang, Diyi Yang,
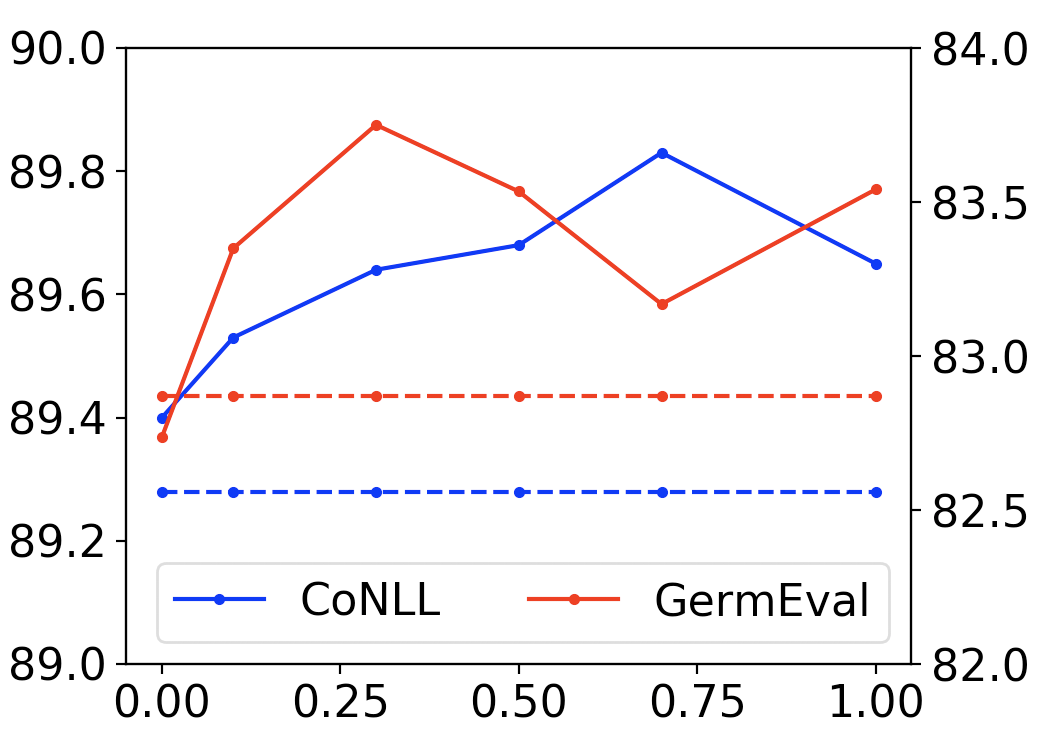
Effective Unsupervised Domain Adaptation with Adversarially Trained Language Models
Thuy-Trang Vu, Dinh Phung, Gholamreza Haffari,
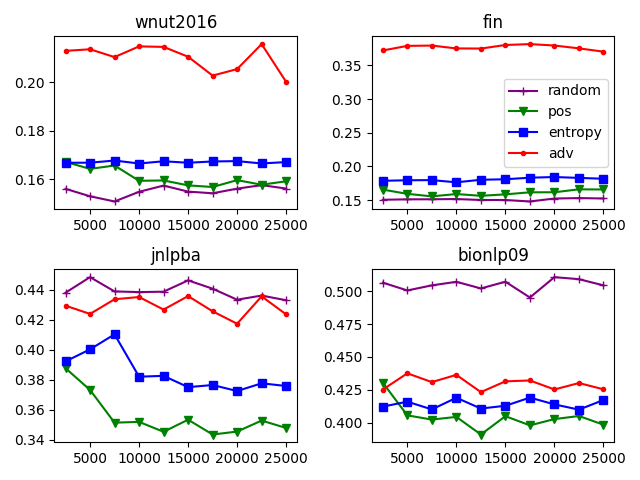
Adversarial Self-Supervised Data-Free Distillation for Text Classification
Xinyin Ma, Yongliang Shen, Gongfan Fang, Chen Chen, Chenghao Jia, Weiming Lu,
