SubjQA: A Dataset for Subjectivity and Review Comprehension
Johannes Bjerva, Nikita Bhutani, Behzad Golshan, Wang-Chiew Tan, Isabelle Augenstein
Question Answering Long Paper
You can open the pre-recorded video in a separate window.
Abstract:
Subjectivity is the expression of internal opinions or beliefs which cannot be objectively observed or verified, and has been shown to be important for sentiment analysis and word-sense disambiguation. Furthermore, subjectivity is an important aspect of user-generated data. In spite of this, subjectivity has not been investigated in contexts where such data is widespread, such as in question answering (QA). We develop a new dataset which allows us to investigate this relationship. We find that subjectivity is an important feature in the case of QA, albeit with more intricate interactions between subjectivity and QA performance than found in previous work on sentiment analysis. For instance, a subjective question may or may not be associated with a subjective answer. We release an English QA dataset (SubjQA) based on customer reviews, containing subjectivity annotations for questions and answer spans across 6 domains.
NOTE: Video may display a random order of authors.
Correct author list is at the top of this page.
Connected Papers in EMNLP2020
Similar Papers
Inquisitive Question Generation for High Level Text Comprehension
Wei-Jen Ko, Te-yuan Chen, Yiyan Huang, Greg Durrett, Junyi Jessy Li,
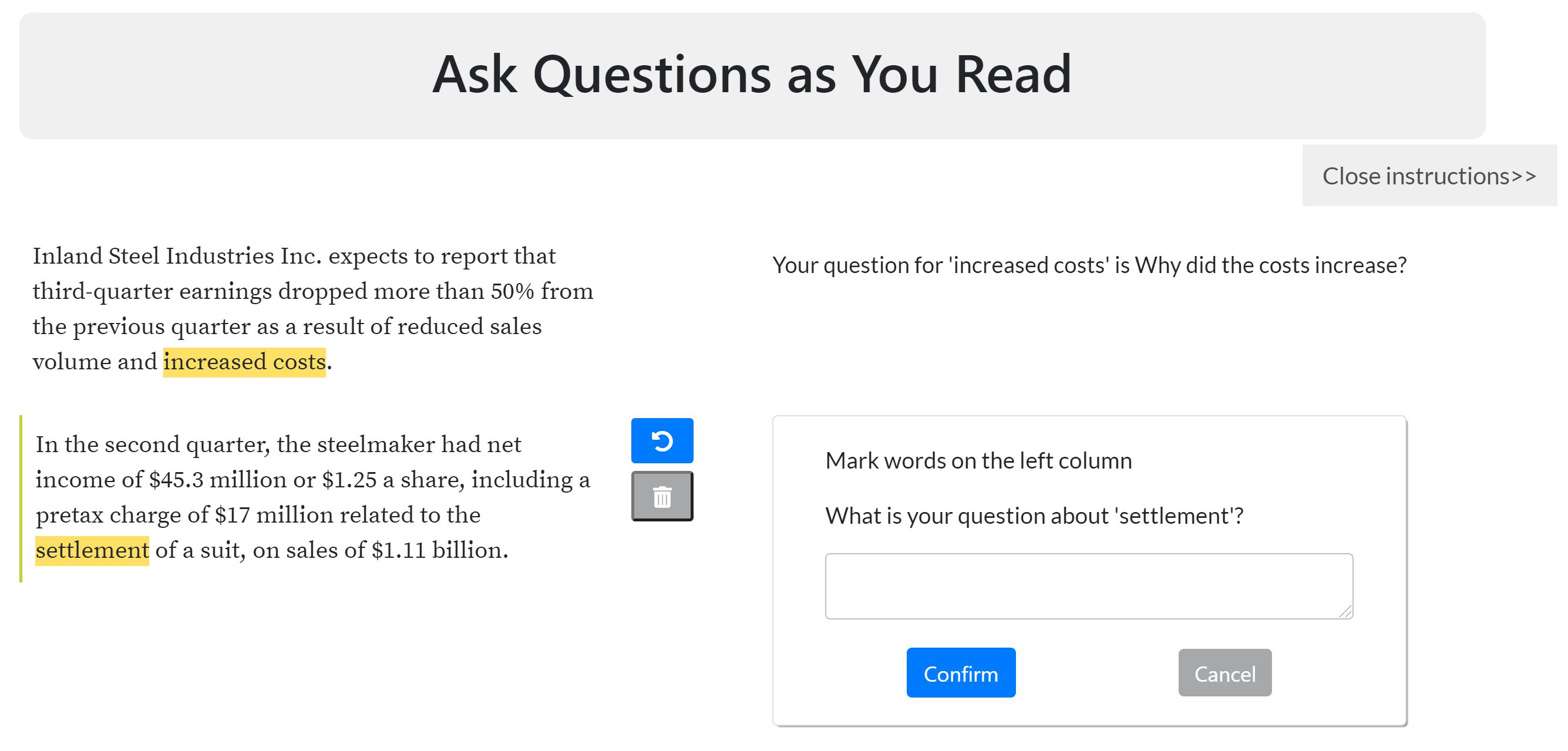
Weakly-Supervised Aspect-Based Sentiment Analysis via Joint Aspect-Sentiment Topic Embedding
Jiaxin Huang, Yu Meng, Fang Guo, Heng Ji, Jiawei Han,

IIRC: A Dataset of Incomplete Information Reading Comprehension Questions
James Ferguson, Matt Gardner, Hannaneh Hajishirzi, Tushar Khot, Pradeep Dasigi,
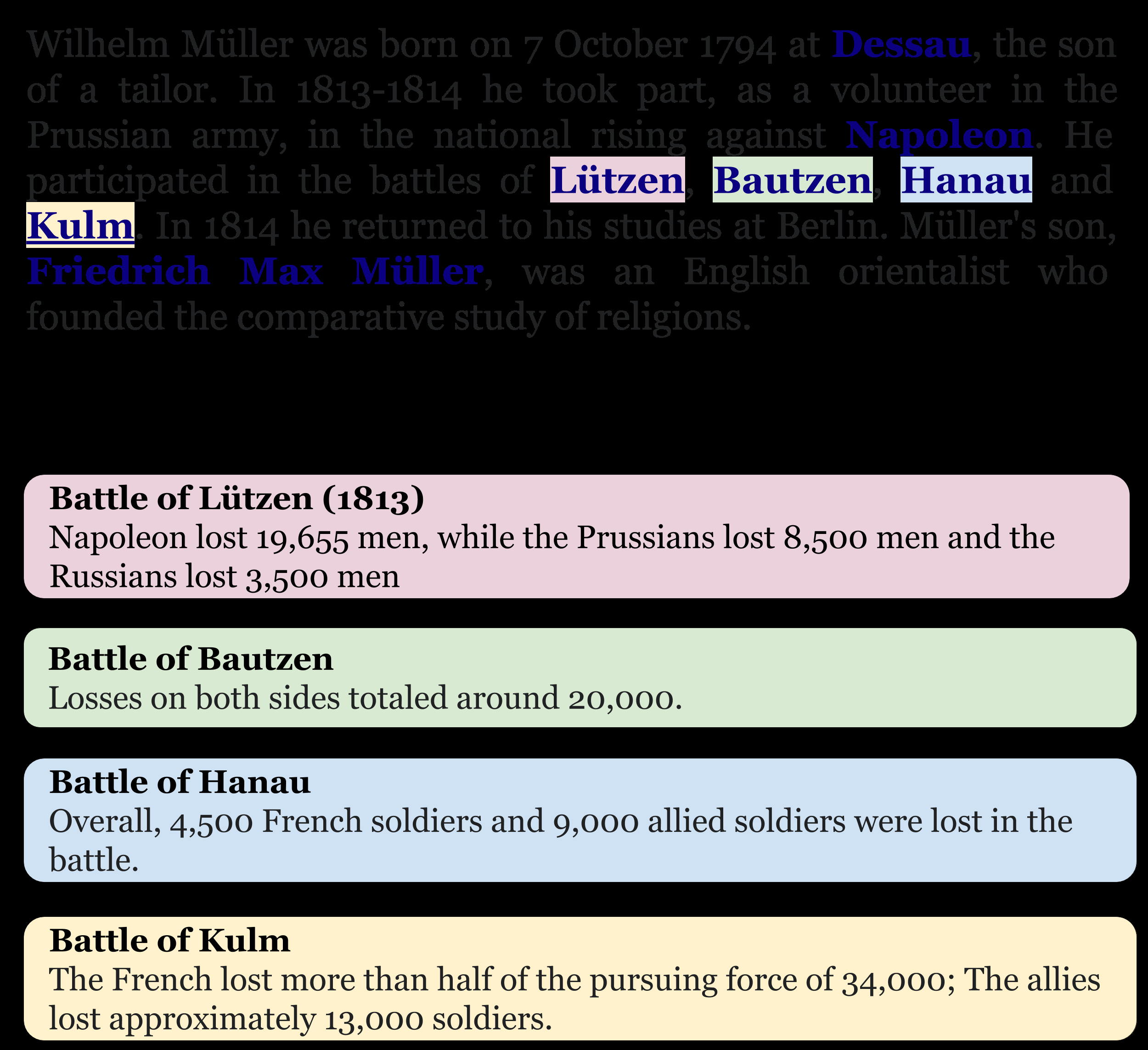
What Can We Learn from Collective Human Opinions on Natural Language Inference Data?
Yixin Nie, Xiang Zhou, Mohit Bansal,
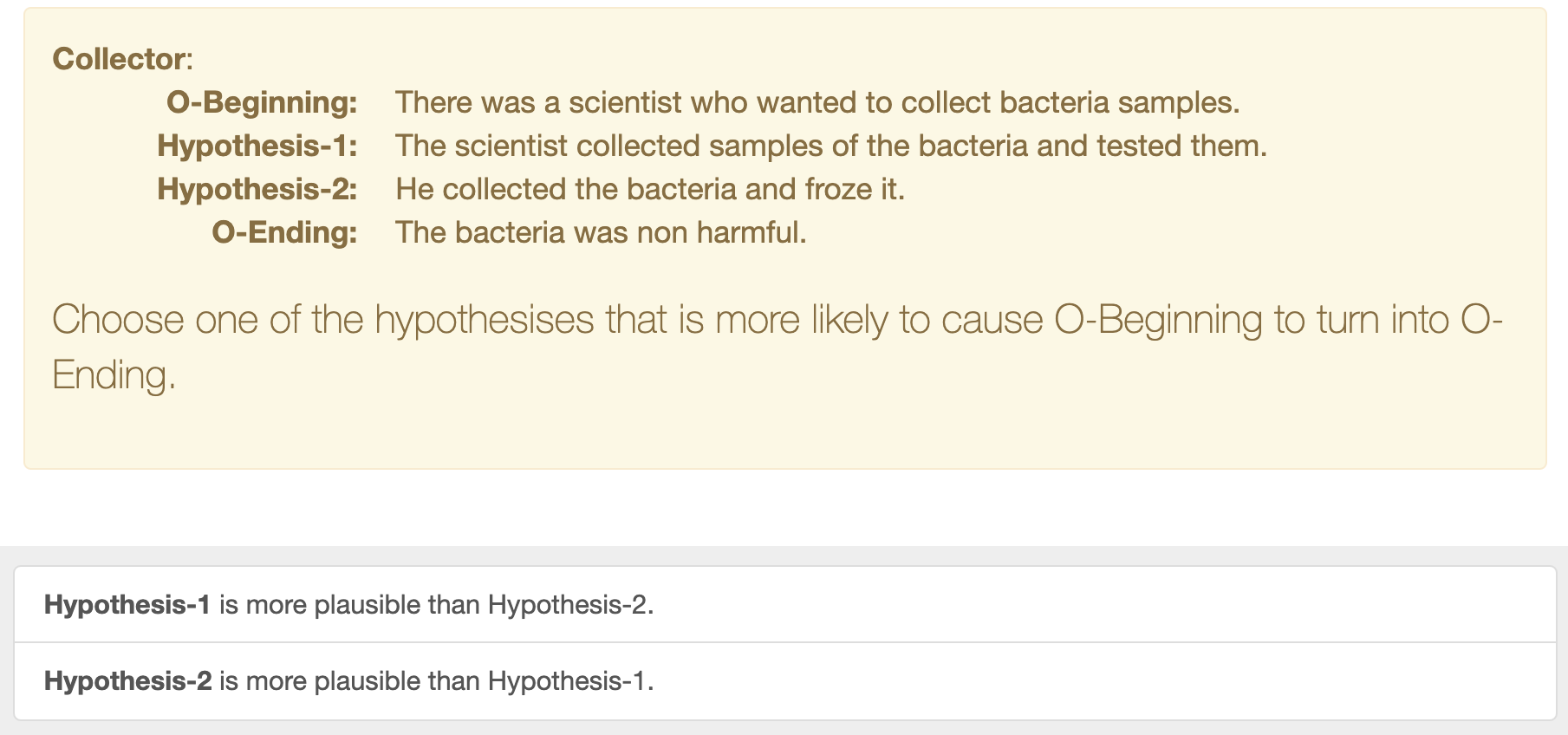