Weakly-Supervised Aspect-Based Sentiment Analysis via Joint Aspect-Sentiment Topic Embedding
Jiaxin Huang, Yu Meng, Fang Guo, Heng Ji, Jiawei Han
Sentiment Analysis, Stylistic Analysis, and Argument Mining Long Paper
You can open the pre-recorded video in a separate window.
Abstract:
Aspect-based sentiment analysis of review texts is of great value for understanding user feedback in a fine-grained manner. It has in general two sub-tasks: (i) extracting aspects from each review, and (ii) classifying aspect-based reviews by sentiment polarity. In this paper, we propose a weakly-supervised approach for aspect-based sentiment analysis, which uses only a few keywords describing each aspect/sentiment without using any labeled examples. Existing methods are either designed only for one of the sub-tasks, or are based on topic models that may contain overlapping concepts. We propose to first learn <sentiment, aspect> joint topic embeddings in the word embedding space by imposing regularizations to encourage topic distinctiveness, and then use neural models to generalize the word-level discriminative information by pre-training the classifiers with embedding-based predictions and self-training them on unlabeled data. Our comprehensive performance analysis shows that our method generates quality joint topics and outperforms the baselines significantly (7.4% and 5.1% F1-score gain on average for aspect and sentiment classification respectively) on benchmark datasets.
NOTE: Video may display a random order of authors.
Correct author list is at the top of this page.
Connected Papers in EMNLP2020
Similar Papers
Multi-Instance Multi-Label Learning Networks for Aspect-Category Sentiment Analysis
Yuncong Li, Cunxiang Yin, Sheng-hua Zhong, Xu Pan,

Diversified Multiple Instance Learning for Document-Level Multi-Aspect Sentiment Classification
Yunjie Ji, Hao Liu, Bolei He, Xinyan Xiao, Hua Wu, Yanhua Yu,

Inducing Target-Specific Latent Structures for Aspect Sentiment Classification
Chenhua Chen, Zhiyang Teng, Yue Zhang,
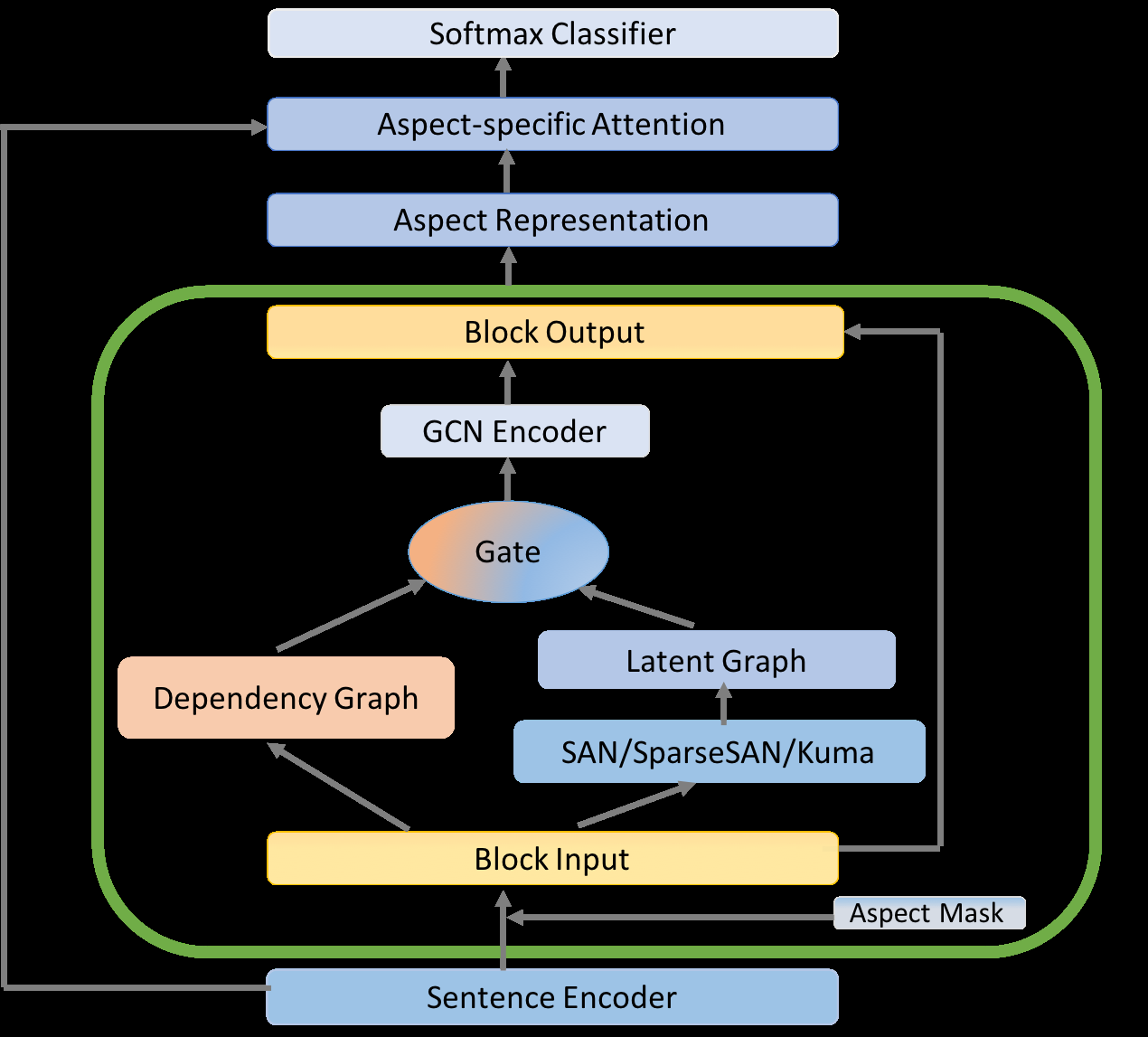
Introducing Syntactic Structures into Target Opinion Word Extraction with Deep Learning
Amir Pouran Ben Veyseh, Nasim Nouri, Franck Dernoncourt, Dejing Dou, Thien Huu Nguyen,
