Hierarchical Graph Network for Multi-hop Question Answering
Yuwei Fang, Siqi Sun, Zhe Gan, Rohit Pillai, Shuohang Wang, Jingjing Liu
Question Answering Long Paper
You can open the pre-recorded video in a separate window.
Abstract:
In this paper, we present Hierarchical Graph Network (HGN) for multi-hop question answering. To aggregate clues from scattered texts across multiple paragraphs, a hierarchical graph is created by constructing nodes on different levels of granularity (questions, paragraphs, sentences, entities), the representations of which are initialized with pre-trained contextual encoders. Given this hierarchical graph, the initial node representations are updated through graph propagation, and multi-hop reasoning is performed via traversing through the graph edges for each subsequent sub-task (e.g., paragraph selection, supporting facts extraction, answer prediction). By weaving heterogeneous nodes into an integral unified graph, this hierarchical differentiation of node granularity enables HGN to support different question answering sub-tasks simultaneously. Experiments on the HotpotQA benchmark demonstrate that the proposed model achieves new state of the art, outperforming existing multi-hop QA approaches.
NOTE: Video may display a random order of authors.
Correct author list is at the top of this page.
Connected Papers in EMNLP2020
Similar Papers
Scalable Multi-Hop Relational Reasoning for Knowledge-Aware Question Answering
Yanlin Feng, Xinyue Chen, Bill Yuchen Lin, Peifeng Wang, Jun Yan, Xiang Ren,
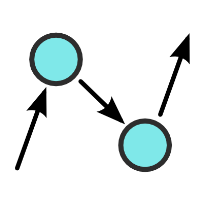
Modeling Global and Local Node Contexts for Text Generation from Knowledge Graphs
Leonardo F. R. Ribeiro, Yue Zhang, Claire Gardent, Iryna Gurevych,

Double Graph Based Reasoning for Document-level Relation Extraction
Shuang Zeng, Runxin Xu, Baobao Chang, Lei Li,
