Connecting the Dots: Event Graph Schema Induction with Path Language Modeling
Manling Li, Qi Zeng, Ying Lin, Kyunghyun Cho, Heng Ji, Jonathan May, Nathanael Chambers, Clare Voss
Information Extraction Long Paper
You can open the pre-recorded video in a separate window.
Abstract:
Event schemas can guide our understanding and ability to make predictions with respect to what might happen next. We propose a new Event Graph Schema, where two event types are connected through multiple paths involving entities that fill important roles in a coherent story. We then introduce Path Language Model, an auto-regressive language model trained on event-event paths, and select salient and coherent paths to probabilistically construct these graph schemas. We design two evaluation metrics, instance coverage and instance coherence, to evaluate the quality of graph schema induction, by checking when coherent event instances are covered by the schema graph. Intrinsic evaluations show that our approach is highly effective at inducing salient and coherent schemas. Extrinsic evaluations show the induced schema repository provides significant improvement to downstream end-to-end Information Extraction over a state-of-the-art joint neural extraction model, when used as additional global features to unfold instance graphs.
NOTE: Video may display a random order of authors.
Correct author list is at the top of this page.
Connected Papers in EMNLP2020
Similar Papers
Hierarchical Graph Network for Multi-hop Question Answering
Yuwei Fang, Siqi Sun, Zhe Gan, Rohit Pillai, Shuohang Wang, Jingjing Liu,
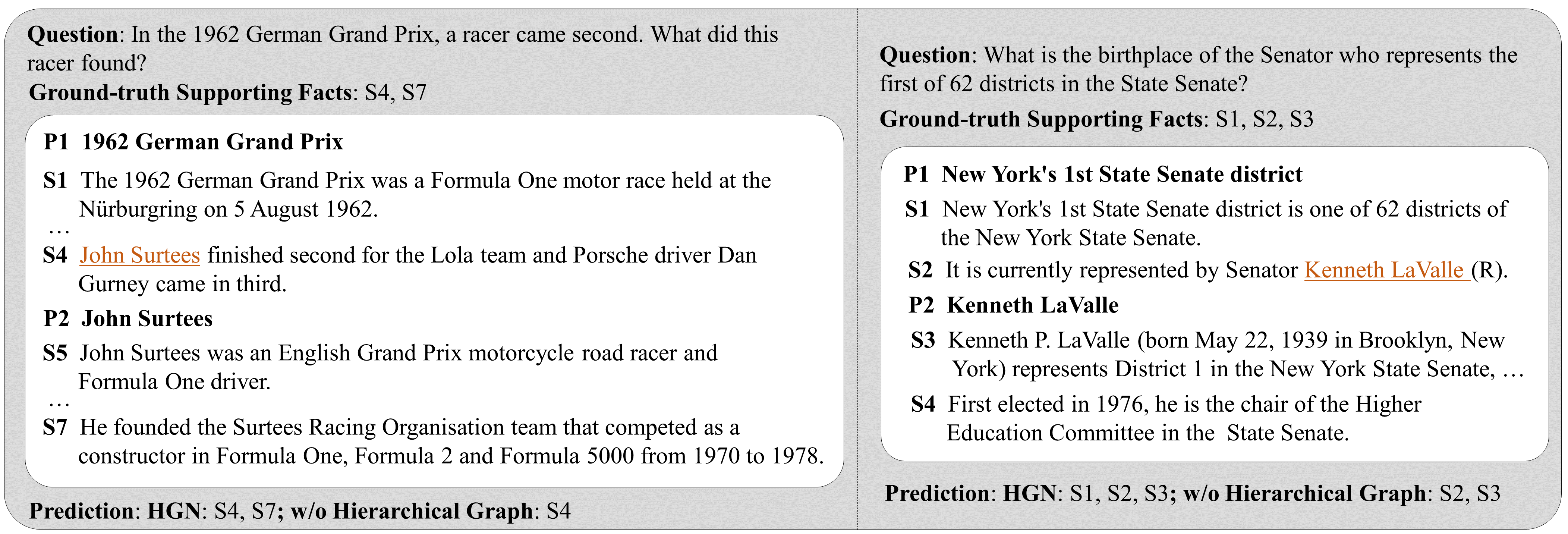
Double Graph Based Reasoning for Document-level Relation Extraction
Shuang Zeng, Runxin Xu, Baobao Chang, Lei Li,

Exploiting Structured Knowledge in Text via Graph-Guided Representation Learning
Tao Shen, Yi Mao, Pengcheng He, Guodong Long, Adam Trischler, Weizhu Chen,
