MedFilter: Improving Extraction of Task-relevant Utterances through Integration of Discourse Structure and Ontological Knowledge
Sopan Khosla, Shikhar Vashishth, Jill Fain Lehman, Carolyn Rose
Information Extraction Long Paper
You can open the pre-recorded video in a separate window.
Abstract:
Information extraction from conversational data is particularly challenging because the task-centric nature of conversation allows for effective communication of implicit information by humans, but is challenging for machines. The challenges may differ between utterances depending on the role of the speaker within the conversation, especially when relevant expertise is distributed asymmetrically across roles. Further, the challenges may also increase over the conversation as more shared context is built up through information communicated implicitly earlier in the dialogue. In this paper, we propose the novel modeling approach MedFilter, which addresses these insights in order to increase performance at identifying and categorizing task-relevant utterances, and in so doing, positively impacts performance at a downstream information extraction task. We evaluate this approach on a corpus of nearly 7,000 doctor-patient conversations where MedFilter is used to identify medically relevant contributions to the discussion (achieving a 10% improvement over SOTA baselines in terms of area under the PR curve). Identifying task-relevant utterances benefits downstream medical processing, achieving improvements of 15%, 105%, and 23% respectively for the extraction of symptoms, medications, and complaints.
NOTE: Video may display a random order of authors.
Correct author list is at the top of this page.
Connected Papers in EMNLP2020
Similar Papers
Conversational Semantic Parsing
Armen Aghajanyan, Jean Maillard, Akshat Shrivastava, Keith Diedrick, Michael Haeger, Haoran Li, Yashar Mehdad, Veselin Stoyanov, Anuj Kumar, Mike Lewis, Sonal Gupta,

Structured Attention for Unsupervised Dialogue Structure Induction
Liang Qiu, Yizhou Zhao, Weiyan Shi, Yuan Liang, Feng Shi, Tao Yuan, Zhou Yu, Song-Chun Zhu,
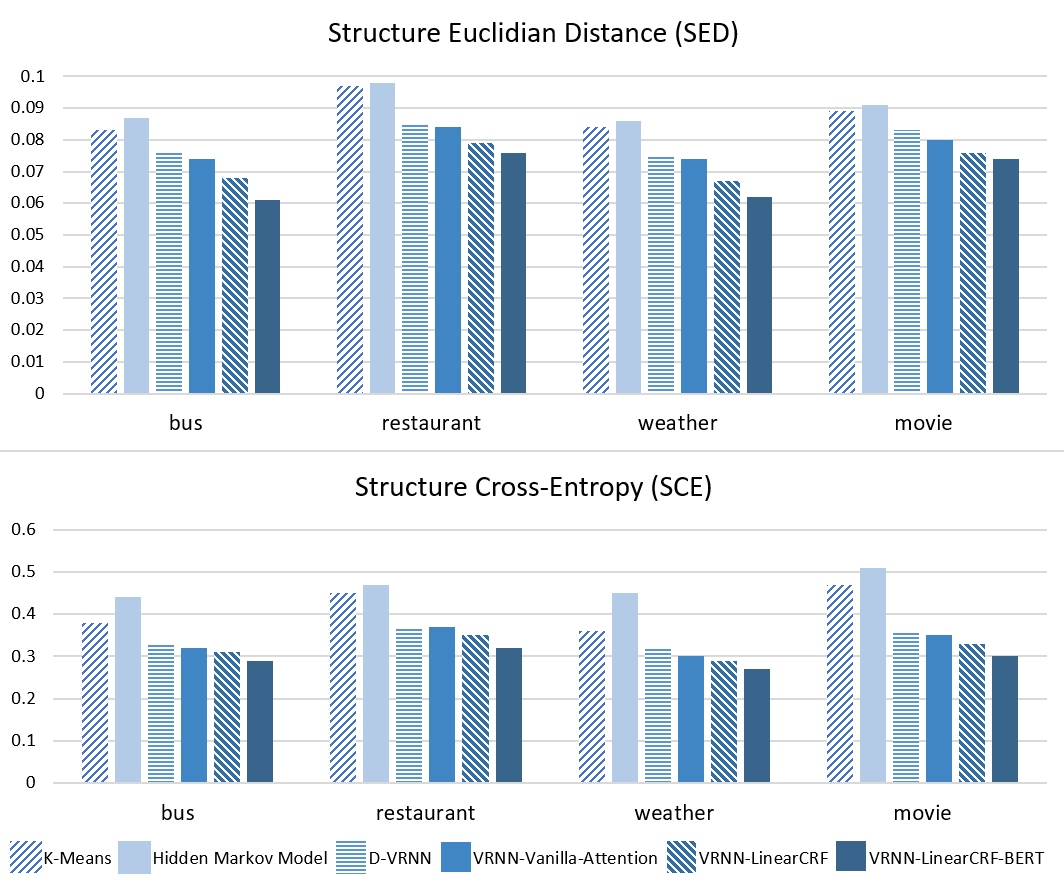
Semantic Role Labeling Guided Multi-turn Dialogue ReWriter
Kun Xu, Haochen Tan, Linfeng Song, Han Wu, Haisong Zhang, Linqi Song, Dong Yu,
