Scene Restoring for Narrative Machine Reading Comprehension
Zhixing Tian, Yuanzhe Zhang, Kang Liu, Jun Zhao, Yantao Jia, Zhicheng Sheng
Question Answering Long Paper
Abstract:
This paper focuses on machine reading comprehension for narrative passages. Narrative passages usually describe a chain of events. When reading this kind of passage, humans tend to restore a scene according to the text with their prior knowledge, which helps them understand the passage comprehensively. Inspired by this behavior of humans, we propose a method to let the machine imagine a scene during reading narrative for better comprehension. Specifically, we build a scene graph by utilizing Atomic as the external knowledge and propose a novel Graph Dimensional-Iteration Network (GDIN) to encode the graph. We conduct experiments on the ROCStories, a dataset of Story Cloze Test (SCT), and CosmosQA, a dataset of multiple choice. Our method achieves state-of-the-art.
Connected Papers in EMNLP2020
Similar Papers
Content Planning for Neural Story Generation with Aristotelian Rescoring
Seraphina Goldfarb-Tarrant, Tuhin Chakrabarty, Ralph Weischedel, Nanyun Peng,
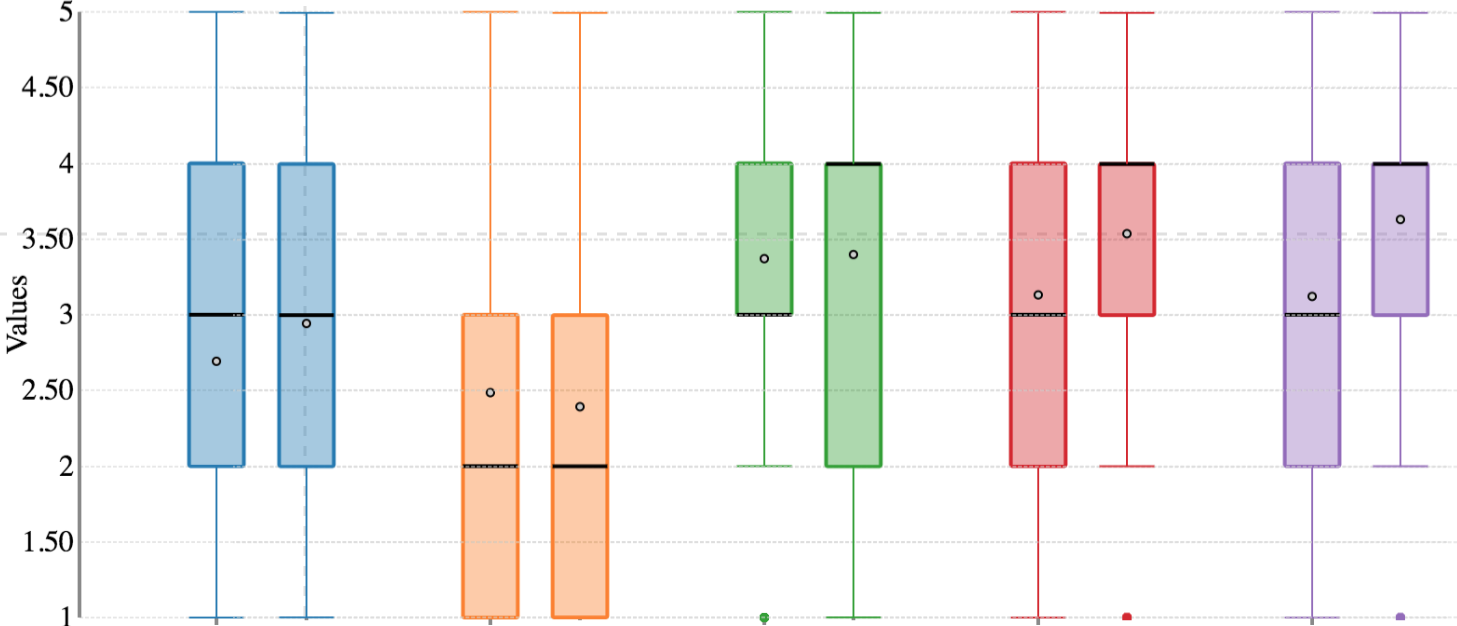
TORQUE: A Reading Comprehension Dataset of Temporal Ordering Questions
Qiang Ning, Hao Wu, Rujun Han, Nanyun Peng, Matt Gardner, Dan Roth,
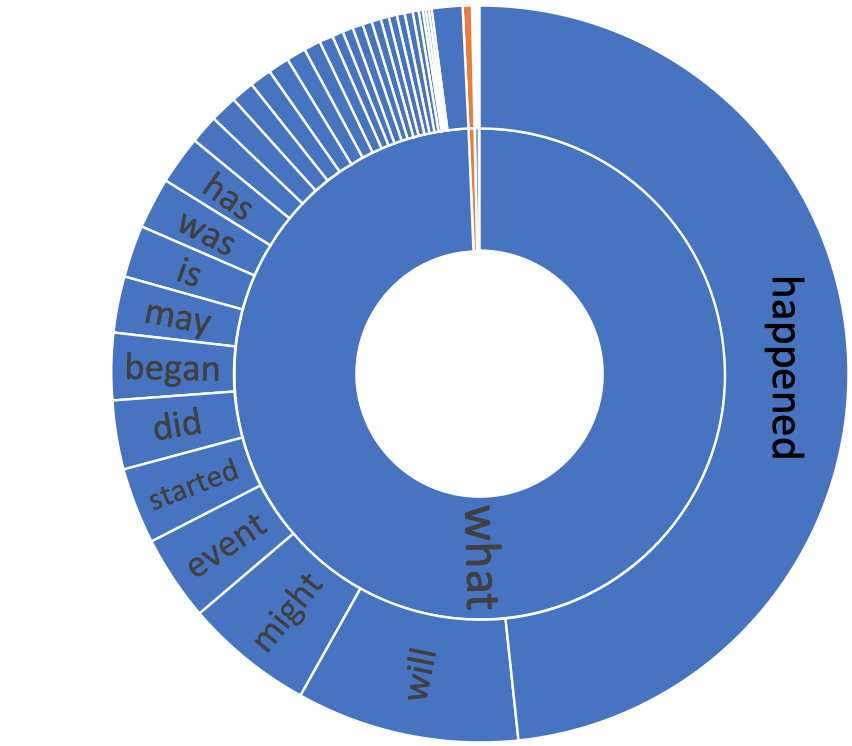
Understanding Procedural Text using Interactive Entity Networks
Jizhi Tang, Yansong Feng, Dongyan Zhao,
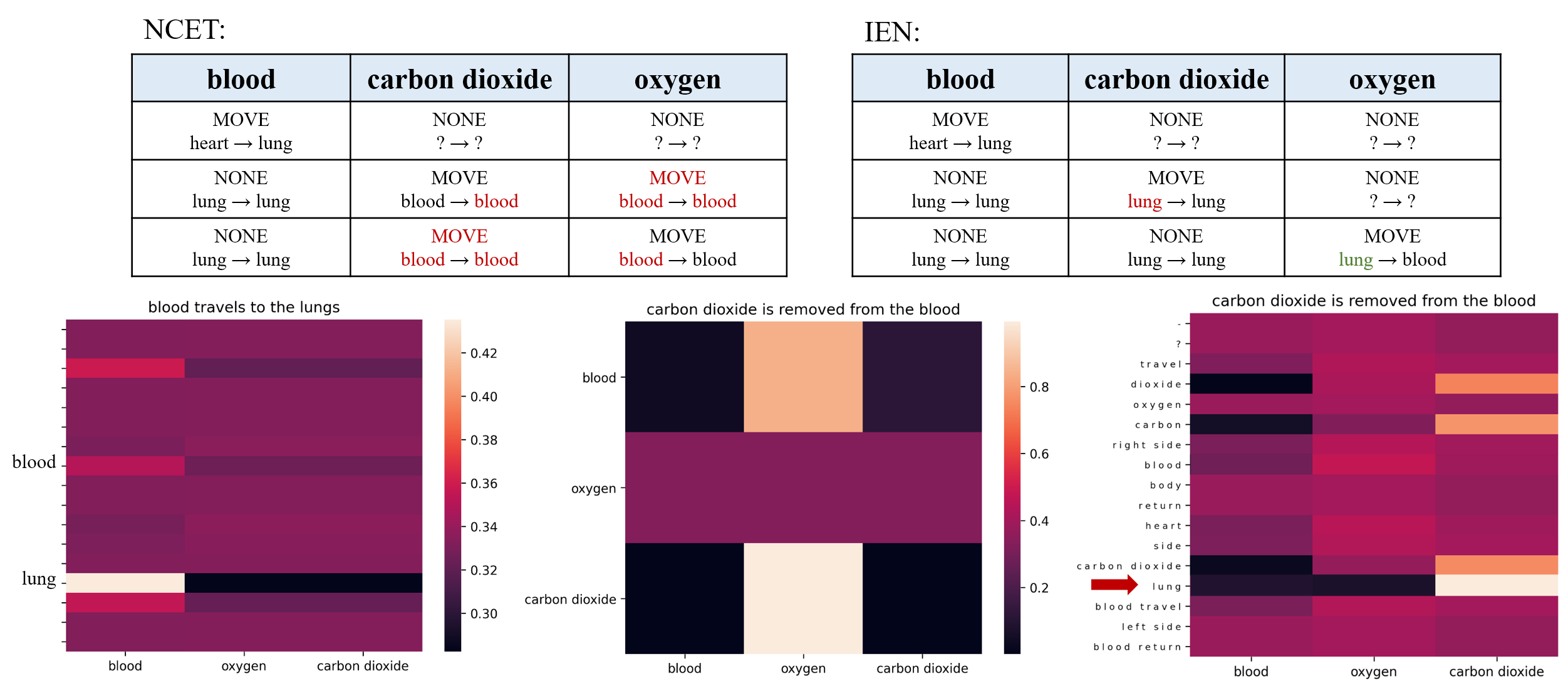