Chapter Captor: Text Segmentation in Novels
Charuta Pethe, Allen Kim, Steve Skiena
NLP Applications Long Paper
You can open the pre-recorded video in a separate window.
Abstract:
Books are typically segmented into chapters and sections, representing coherent sub-narratives and topics. We investigate the task of predicting chapter boundaries, as a proxy for the general task of segmenting long texts. We build a Project Gutenberg chapter segmentation data set of 9,126 English novels, using a hybrid approach combining neural inference and rule matching to recognize chapter title headers in books, achieving an F1-score of 0.77 on this task. Using this annotated data as ground truth after removing structural cues, we present cut-based and neural methods for chapter segmentation, achieving a F1-score of 0.453 on the challenging task of exact break prediction over book-length documents. Finally, we reveal interesting historical trends in the chapter structure of novels.
NOTE: Video may display a random order of authors.
Correct author list is at the top of this page.
Connected Papers in EMNLP2020
Similar Papers
Better Highlighting: Creating Sub-Sentence Summary Highlights
Sangwoo Cho, Kaiqiang Song, Chen Li, Dong Yu, Hassan Foroosh, Fei Liu,
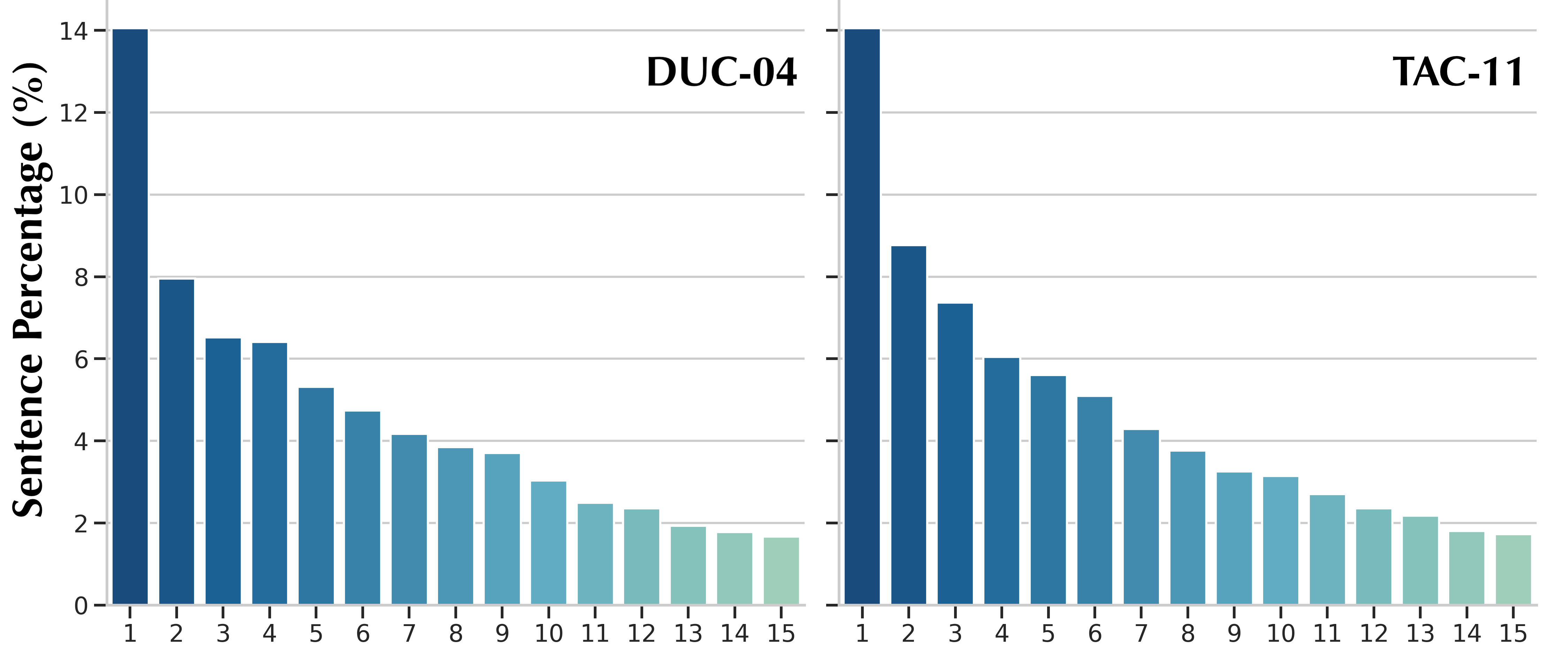
ToTTo: A Controlled Table-To-Text Generation Dataset
Ankur Parikh, Xuezhi Wang, Sebastian Gehrmann, Manaal Faruqui, Bhuwan Dhingra, Diyi Yang, Dipanjan Das,
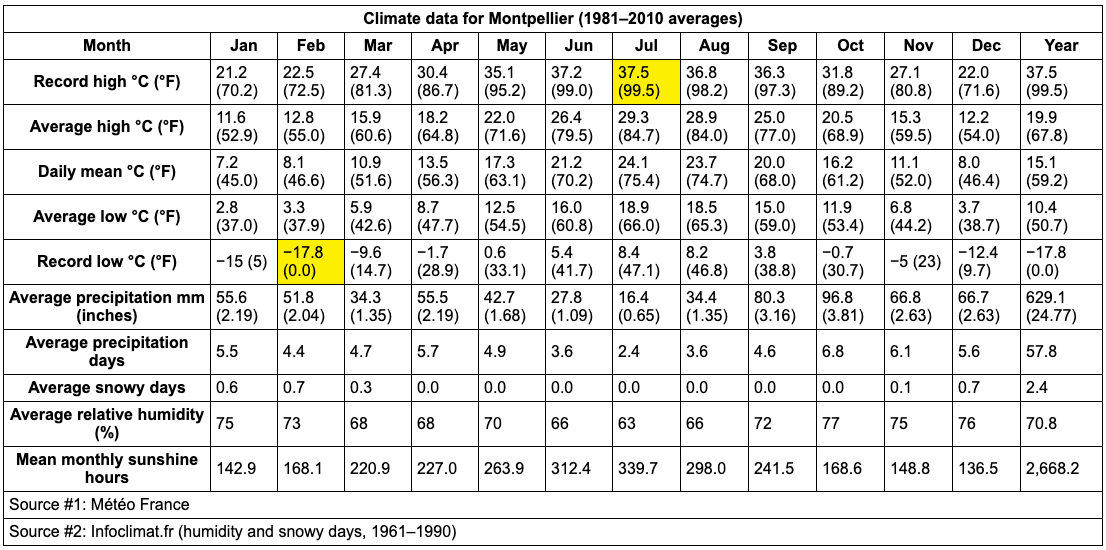
Understanding Neural Abstractive Summarization Models via Uncertainty
Jiacheng Xu, Shrey Desai, Greg Durrett,
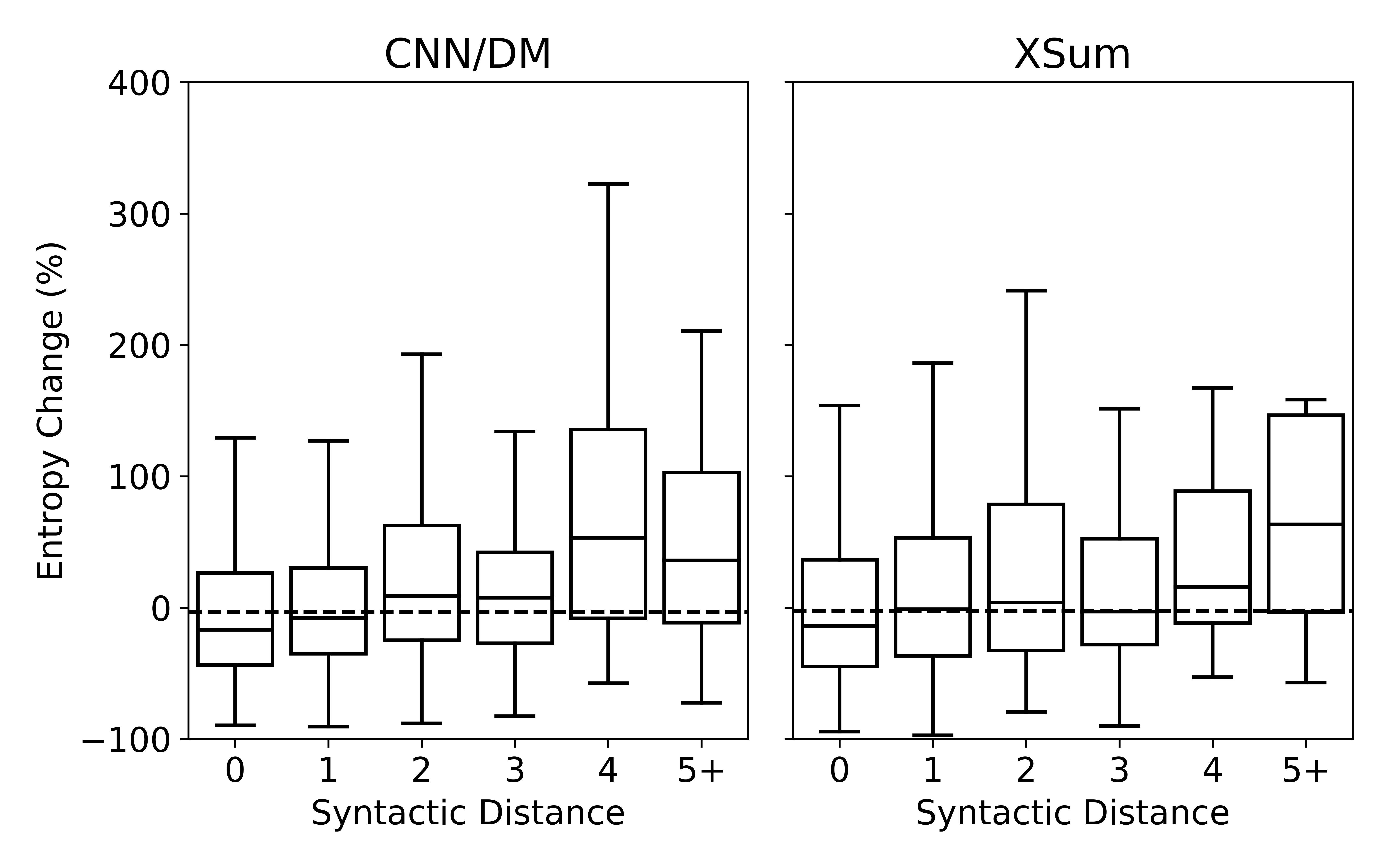