A Knowledge-driven Generative Model for Multi-implication Chinese Medical Procedure Entity Normalization
Jinghui Yan, Yining Wang, Lu Xiang, Yu Zhou, Chengqing Zong
NLP Applications Long Paper
You can open the pre-recorded video in a separate window.
Abstract:
Medical entity normalization, which links medical mentions in the text to entities in knowledge bases, is an important research topic in medical natural language processing. In this paper, we focus on Chinese medical procedure entity normalization. However, nonstandard Chinese expressions and combined procedures present challenges in our problem. The existing strategies relying on the discriminative model are poorly to cope with normalizing combined procedure mentions. We propose a sequence generative framework to directly generate all the corresponding medical procedure entities. we adopt two strategies: category-based constraint decoding and category-based model refining to avoid unrealistic results. The method is capable of linking entities when a mention contains multiple procedure concepts and our comprehensive experiments demonstrate that the proposed model can achieve remarkable improvements over existing baselines, particularly significant in the case of multi-implication Chinese medical procedures.
NOTE: Video may display a random order of authors.
Correct author list is at the top of this page.
Connected Papers in EMNLP2020
Similar Papers
Named Entity Recognition for Social Media Texts with Semantic Augmentation
Yuyang Nie, Yuanhe Tian, Xiang Wan, Yan Song, Bo Dai,
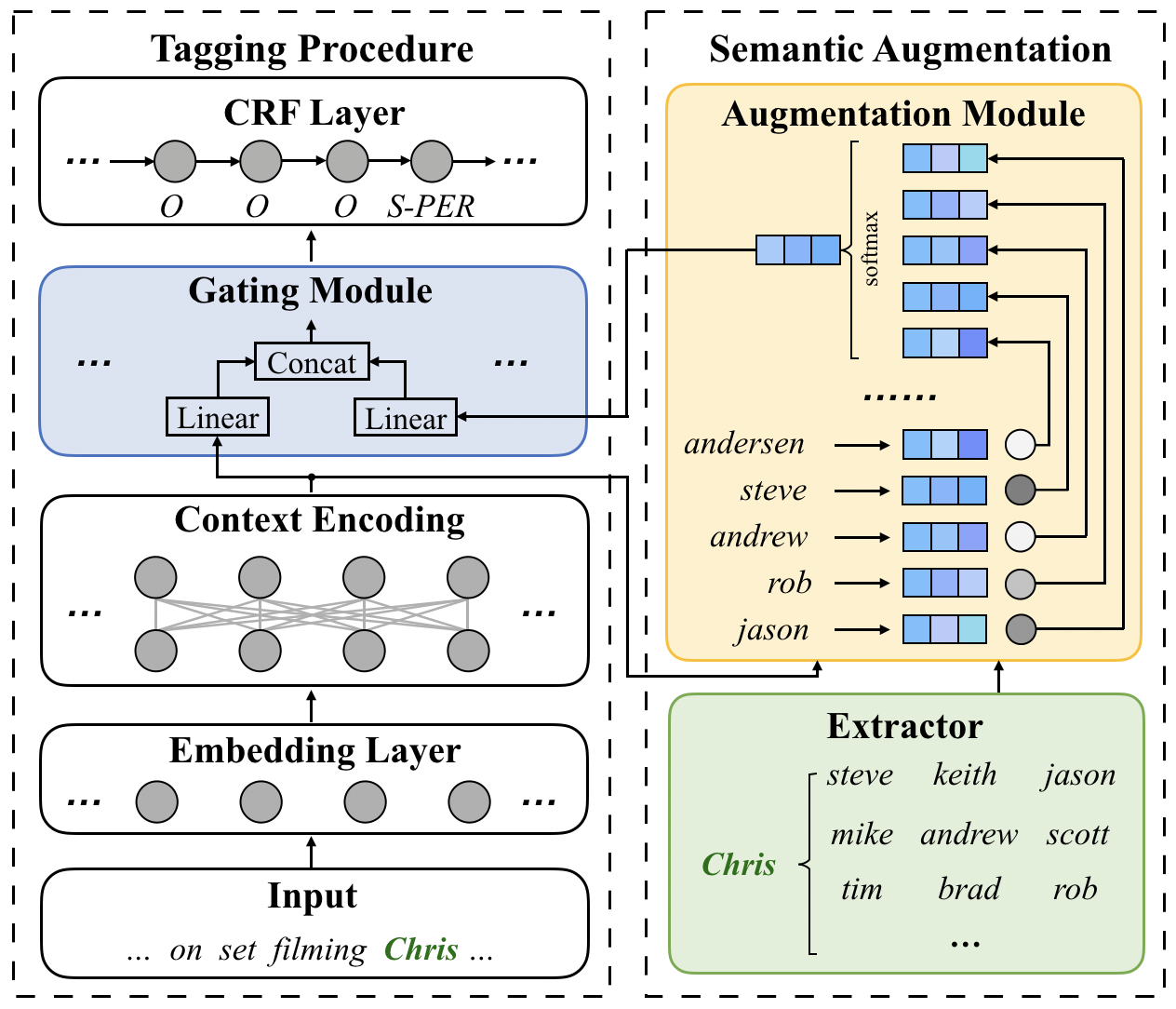
Modeling Content Importance for Summarization with Pre-trained Language Models
Liqiang Xiao, Lu Wang, Hao He, Yaohui Jin,
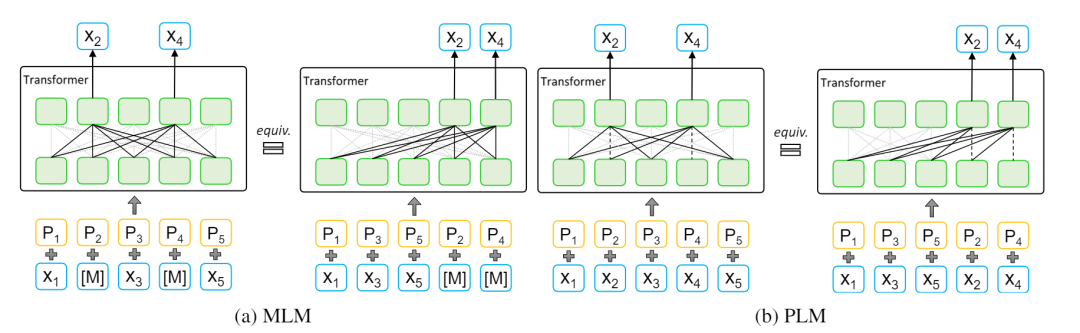