AutoQA: From Databases To QA Semantic Parsers With Only Synthetic Training Data
Silei Xu, Sina Semnani, Giovanni Campagna, Monica Lam
Semantics: Sentence-level Semantics, Textual Inference and Other areas Long Paper
You can open the pre-recorded video in a separate window.
Abstract:
We propose AutoQA, a methodology and toolkit to generate semantic parsers that answer questions on databases, with no manual effort. Given a database schema and its data, AutoQA automatically generates a large set of high-quality questions for training that covers different database operations. It uses automatic paraphrasing combined with template-based parsing to find alternative expressions of an attribute in different parts of speech. It also uses a novel filtered auto-paraphraser to generate correct paraphrases of entire sentences. We apply AutoQA to the Schema2QA dataset and obtain an average logical form accuracy of 62.9% when tested on natural questions, which is only 6.4% lower than a model trained with expert natural language annotations and paraphrase data collected from crowdworkers. To demonstrate the generality of AutoQA, we also apply it to the Overnight dataset. AutoQA achieves 69.8% answer accuracy, 16.4% higher than the state-of-the-art zero-shot models and only 5.2% lower than the same model trained with human data.
NOTE: Video may display a random order of authors.
Correct author list is at the top of this page.
Connected Papers in EMNLP2020
Similar Papers
AutoPrompt: Eliciting Knowledge from Language Models with Automatically Generated Prompts
Taylor Shin, Yasaman Razeghi, Robert L. Logan IV, Eric Wallace, Sameer Singh,

Automatic Machine Translation Evaluation in Many Languages via Zero-Shot Paraphrasing
Brian Thompson, Matt Post,

Training Question Answering Models From Synthetic Data
Raul Puri, Ryan Spring, Mohammad Shoeybi, Mostofa Patwary, Bryan Catanzaro,
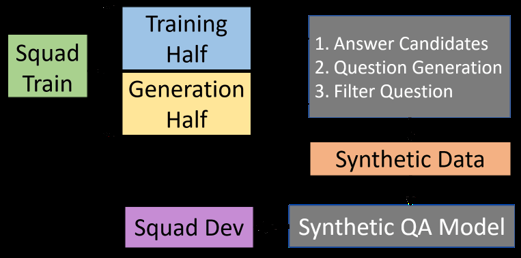