Towards Understanding Sample Variance in Visually Grounded Language Generation: Evaluations and Observations
Wanrong Zhu, Xin Wang, Pradyumna Narayana, Kazoo Sone, Sugato Basu, William Yang Wang
Language Grounding to Vision, Robotics and Beyond Short Paper
You can open the pre-recorded video in a separate window.
Abstract:
A major challenge in visually grounded language generation is to build robust benchmark datasets and models that can generalize well in real-world settings. To do this, it is critical to ensure that our evaluation protocols are correct, and benchmarks are reliable. In this work, we set forth to design a set of experiments to understand an important but often ignored problem in visually grounded language generation: given that humans have different utilities and visual attention, how will the sample variance in multi-reference datasets affect the models' performance? Empirically, we study several multi-reference datasets and corresponding vision-and-language tasks. We show that it is of paramount importance to report variance in experiments; that human-generated references could vary drastically in different datasets/tasks, revealing the nature of each task; that metric-wise, CIDEr has shown systematically larger variances than others. Our evaluations on reference-per-instance shed light on the design of reliable datasets in the future.
NOTE: Video may display a random order of authors.
Correct author list is at the top of this page.
Connected Papers in EMNLP2020
Similar Papers
STORIUM: A Dataset and Evaluation Platform for Machine-in-the-Loop Story Generation
Nader Akoury, Shufan Wang, Josh Whiting, Stephen Hood, Nanyun Peng, Mohit Iyyer,

MOCHA: A Dataset for Training and Evaluating Generative Reading Comprehension Metrics
Anthony Chen, Gabriel Stanovsky, Sameer Singh, Matt Gardner,
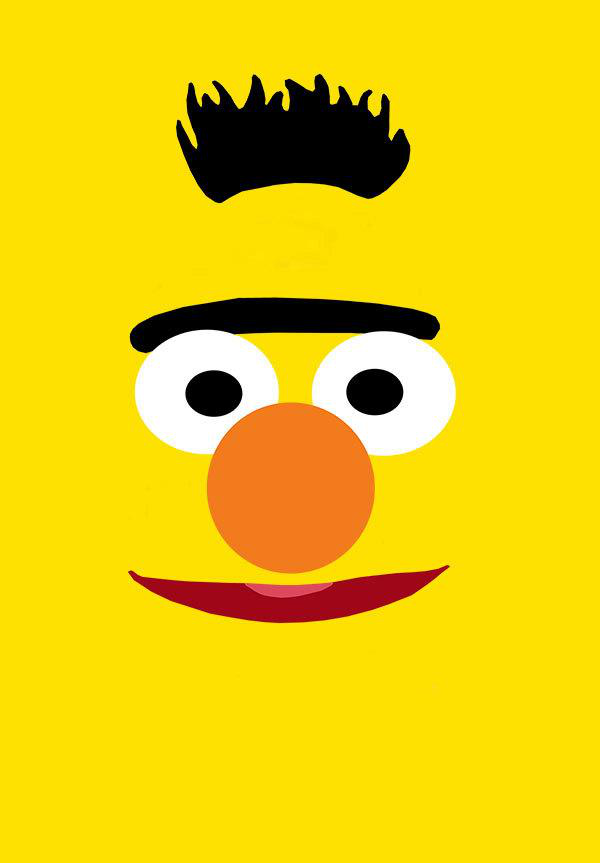
New Protocols and Negative Results for Textual Entailment Data Collection
Samuel R. Bowman, Jennimaria Palomaki, Livio Baldini Soares, Emily Pitler,
