STORIUM: A Dataset and Evaluation Platform for Machine-in-the-Loop Story Generation
Nader Akoury, Shufan Wang, Josh Whiting, Stephen Hood, Nanyun Peng, Mohit Iyyer
Language Generation Long Paper
You can open the pre-recorded video in a separate window.
Abstract:
Systems for story generation are asked to produce plausible and enjoyable stories given an input context. This task is underspecified, as a vast number of diverse stories can originate from a single input. The large output space makes it difficult to build and evaluate story generation models, as (1) existing datasets lack rich enough contexts to meaningfully guide models, and (2) existing evaluations (both crowdsourced and automatic) are unreliable for assessing long-form creative text. To address these issues, we introduce a dataset and evaluation platform built from STORIUM, an online collaborative storytelling community. Our author-generated dataset contains 6K lengthy stories (125M tokens) with fine-grained natural language annotations (e.g., character goals and attributes) interspersed throughout each narrative, forming a robust source for guiding models. We evaluate language models fine-tuned on our dataset by integrating them onto STORIUM, where real authors can query a model for suggested story continuations and then edit them. Automatic metrics computed over these edits correlate well with both user ratings of generated stories and qualitative feedback from semi-structured user interviews. We release both the STORIUM dataset and evaluation platform to spur more principled research into story generation.
NOTE: Video may display a random order of authors.
Correct author list is at the top of this page.
Connected Papers in EMNLP2020
Similar Papers
MEGATRON-CNTRL: Controllable Story Generation with External Knowledge Using Large-Scale Language Models
Peng Xu, Mostofa Patwary, Mohammad Shoeybi, Raul Puri, Pascale Fung, Anima Anandkumar, Bryan Catanzaro,
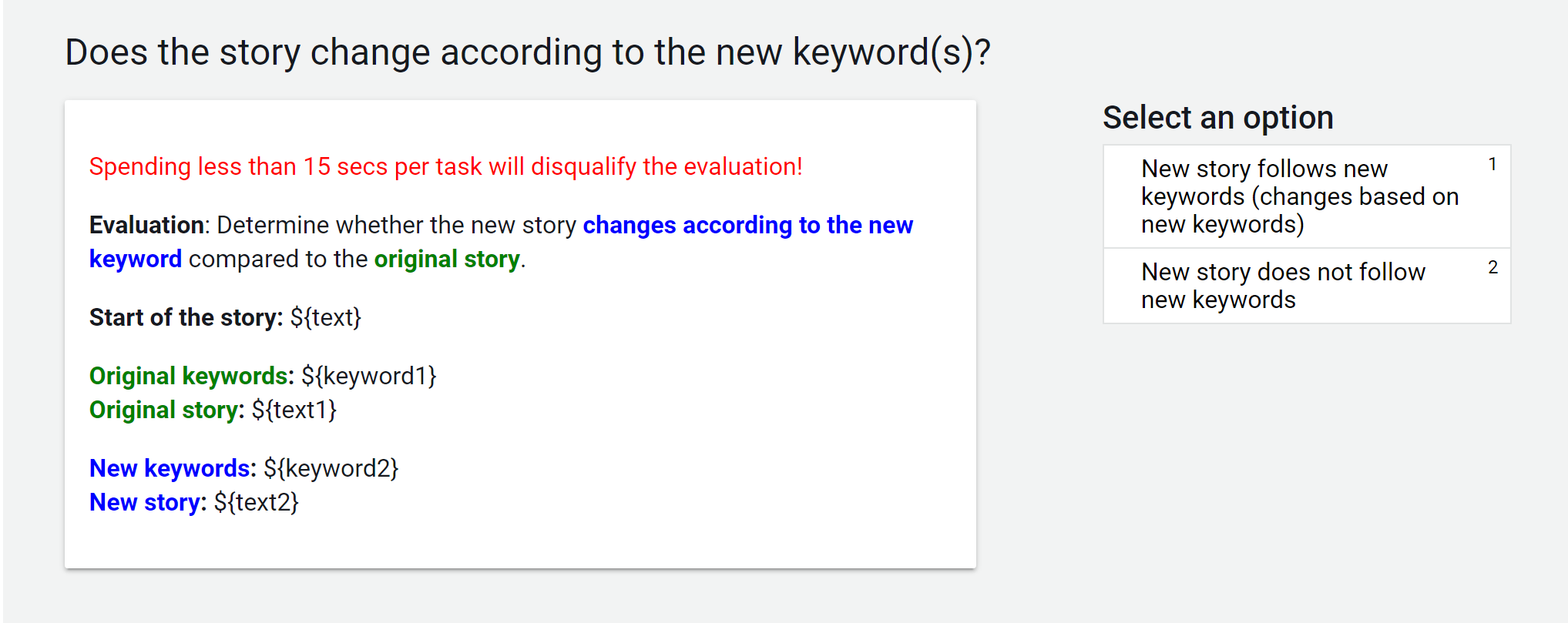
Reading Between the Lines: Exploring Infilling in Visual Narratives
Khyathi Raghavi Chandu, Ruo-Ping Dong, Alan W Black,
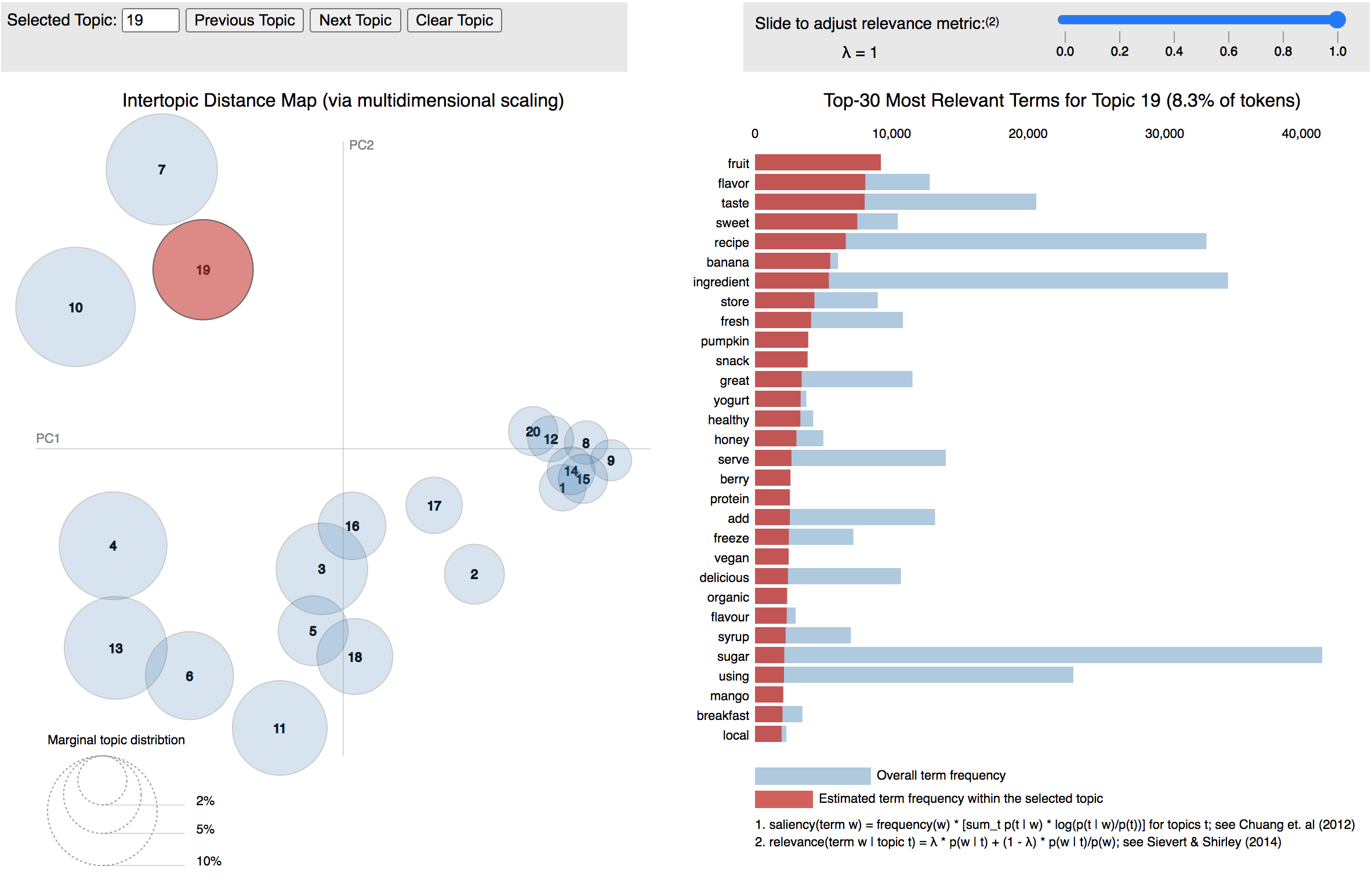
PathQG: Neural Question Generation from Facts
Siyuan Wang, Zhongyu Wei, Zhihao Fan, Zengfeng Huang, Weijian Sun, Qi Zhang, Xuanjing Huang,
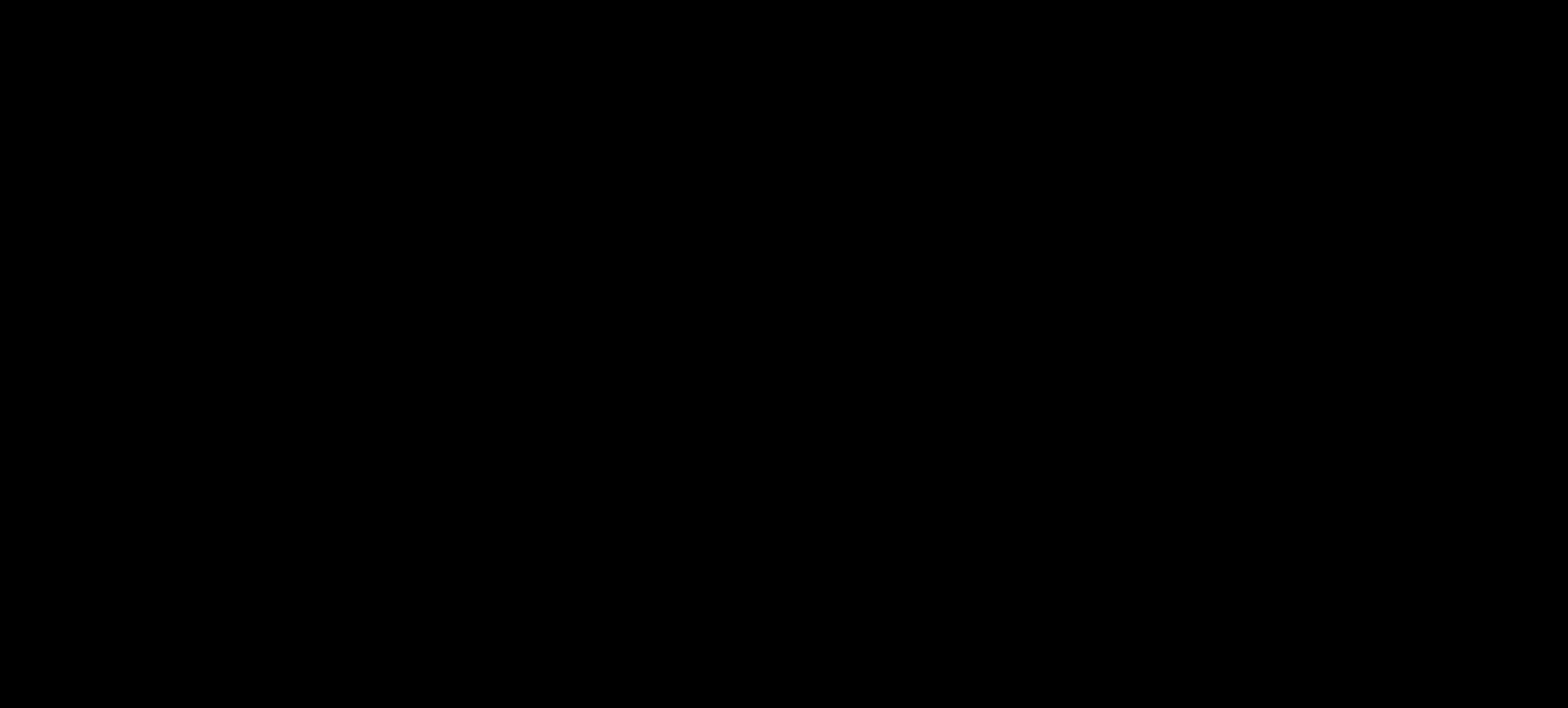
Grounded Compositional Outputs for Adaptive Language Modeling
Nikolaos Pappas, Phoebe Mulcaire, Noah A. Smith,
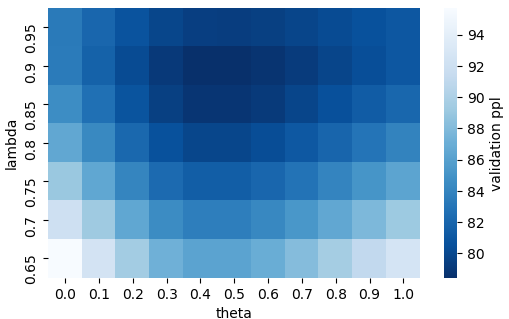