Learning from Task Descriptions
Orion Weller, Nicholas Lourie, Matt Gardner, Matthew Peters
Machine Learning for NLP Long Paper
You can open the pre-recorded video in a separate window.
Abstract:
Typically, machine learning systems solve new tasks by training on thousands of examples. In contrast, humans can solve new tasks by reading some instructions, with perhaps an example or two. To take a step toward closing this gap, we introduce a framework for developing NLP systems that solve new tasks after reading their descriptions, synthesizing prior work in this area. We instantiate this frame- work with a new English language dataset, ZEST, structured for task-oriented evaluation on unseen tasks. Formulating task descriptions as questions, we ensure each is general enough to apply to many possible inputs, thus comprehensively evaluating a model’s ability to solve each task. Moreover, the dataset’s structure tests specific types of systematic generalization. We find that the state-of-the-art T5 model achieves a score of 12% on ZEST, leaving a significant challenge for NLP researchers.
NOTE: Video may display a random order of authors.
Correct author list is at the top of this page.
Connected Papers in EMNLP2020
Similar Papers
Universal Natural Language Processing with Limited Annotations: Try Few-shot Textual Entailment as a Start
Wenpeng Yin, Nazneen Fatema Rajani, Dragomir Radev, Richard Socher, Caiming Xiong,
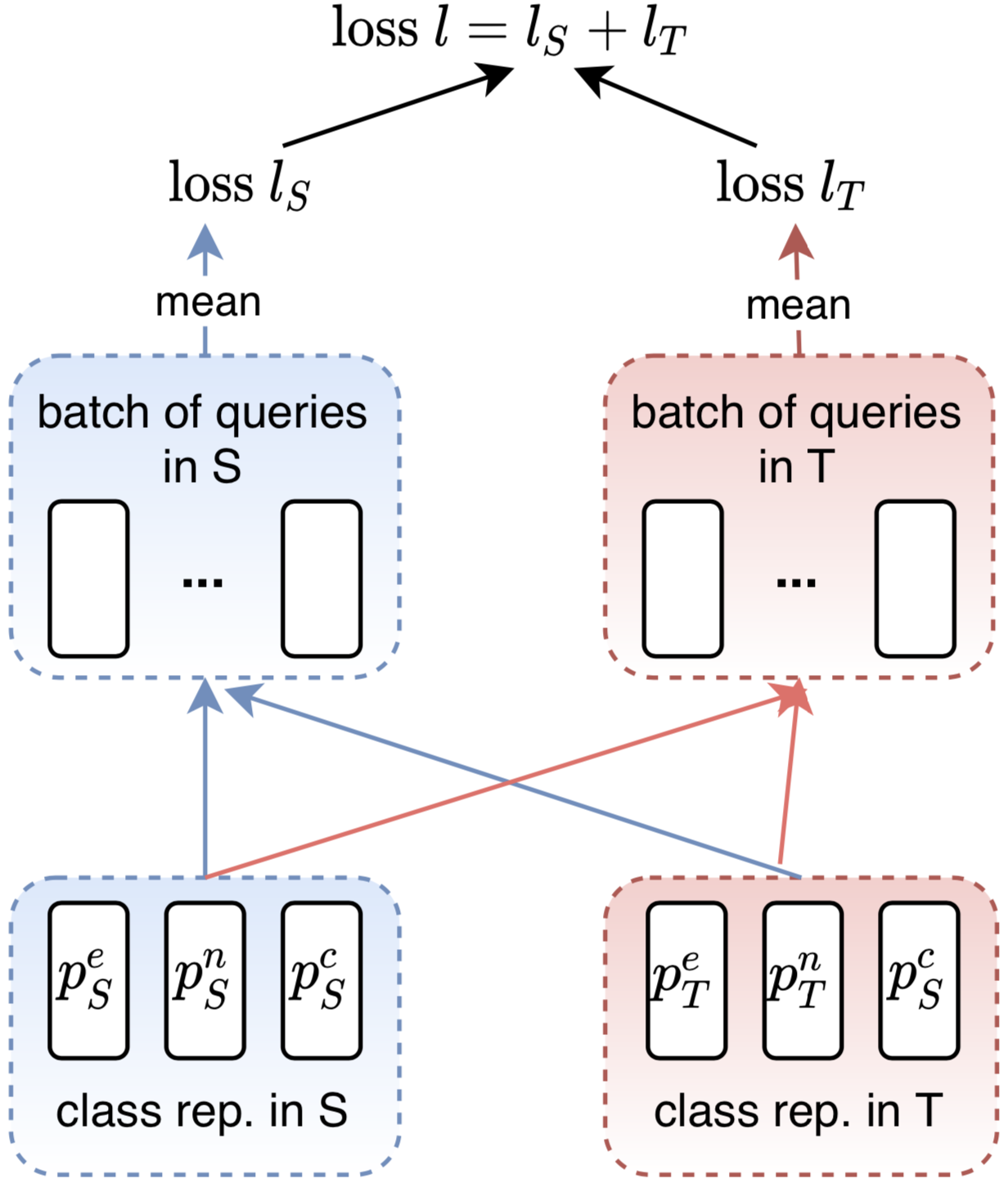
New Protocols and Negative Results for Textual Entailment Data Collection
Samuel R. Bowman, Jennimaria Palomaki, Livio Baldini Soares, Emily Pitler,

Efficient Meta Lifelong-Learning with Limited Memory
Zirui Wang, Sanket Vaibhav Mehta, Barnabas Poczos, Jaime Carbonell,
