Deep Weighted MaxSAT for Aspect-based Opinion Extraction
Meixi Wu, Wenya Wang, Sinno Jialin Pan
Sentiment Analysis, Stylistic Analysis, and Argument Mining Long Paper
You can open the pre-recorded video in a separate window.
Abstract:
Though deep learning has achieved significant success in various NLP tasks, most deep learning models lack the capability of encoding explicit domain knowledge to model complex causal relationships among different types of variables. On the other hand, logic rules offer a compact expression to represent the causal relationships to guide the training process. Logic programs can be cast as a satisfiability problem which aims to find truth assignments to logic variables by maximizing the number of satisfiable clauses (MaxSAT). We adopt the MaxSAT semantics to model logic inference process and smoothly incorporate a weighted version of MaxSAT that connects deep neural networks and a graphical model in a joint framework. The joint model feeds deep learning outputs to a weighted MaxSAT layer to rectify the erroneous predictions and can be trained via end-to-end gradient descent. Our proposed model associates the benefits of high-level feature learning, knowledge reasoning, and structured learning with observable performance gain for the task of aspect-based opinion extraction.
NOTE: Video may display a random order of authors.
Correct author list is at the top of this page.
Connected Papers in EMNLP2020
Similar Papers
Domain Knowledge Empowered Structured Neural Net for End-to-End Event Temporal Relation Extraction
Rujun Han, Yichao Zhou, Nanyun Peng,
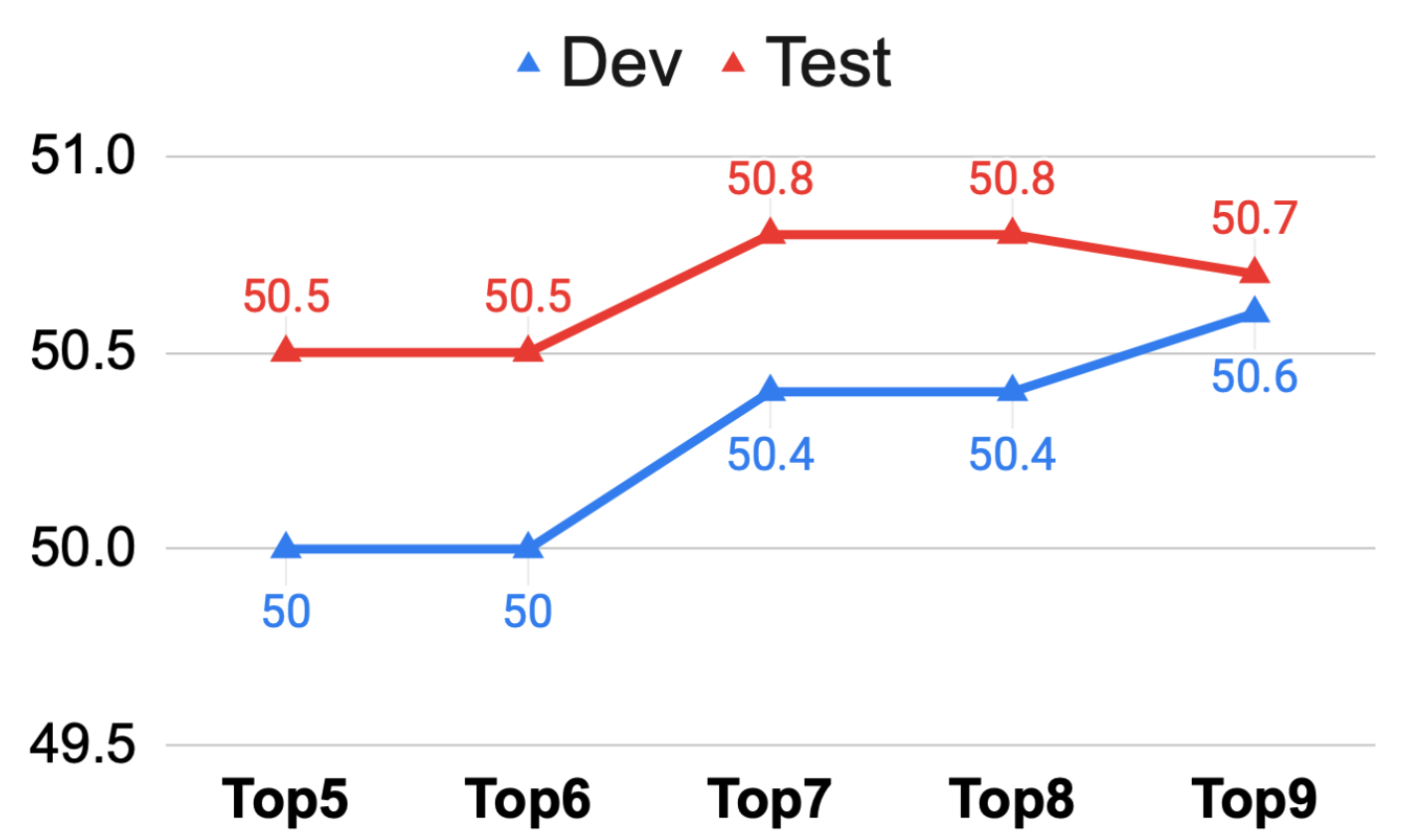
Knowledge-guided Open Attribute Value Extraction with Reinforcement Learning
Ye Liu, Sheng Zhang, Rui Song, Suo Feng, Yanghua Xiao,
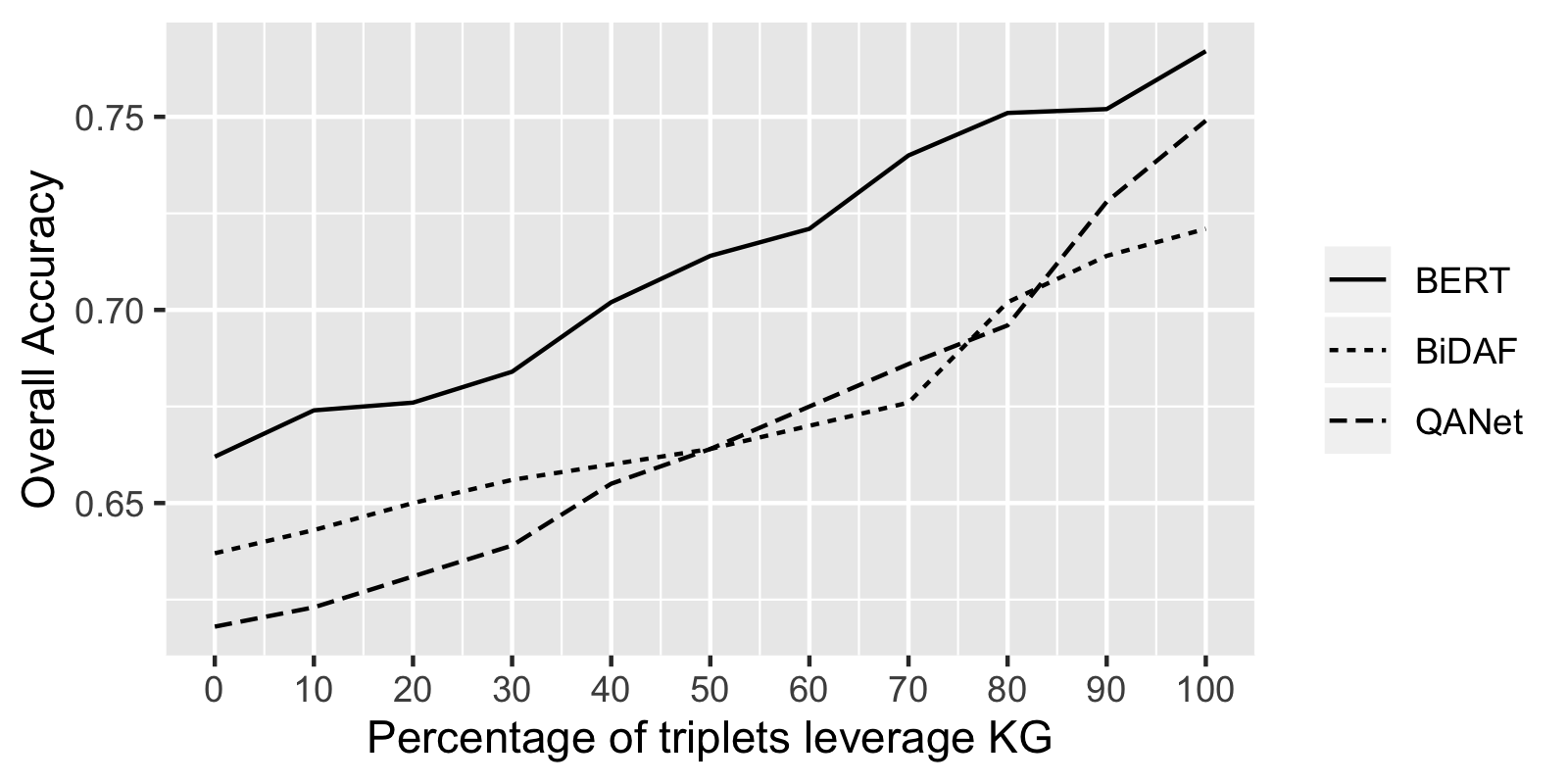
Q-learning with Language Model for Edit-based Unsupervised Summarization
Ryosuke Kohita, Akifumi Wachi, Yang Zhao, Ryuki Tachibana,

Meta Fine-Tuning Neural Language Models for Multi-Domain Text Mining
Chengyu Wang, Minghui Qiu, Jun Huang, Xiaofeng He,
