Knowledge-guided Open Attribute Value Extraction with Reinforcement Learning
Ye Liu, Sheng Zhang, Rui Song, Suo Feng, Yanghua Xiao
Information Extraction Long Paper
You can open the pre-recorded video in a separate window.
Abstract:
Open attribute value extraction for emerging entities is an important but challenging task. A lot of previous works formulate the problem as a question-answering (QA) task. While the collections of articles from web corpus provide updated information about the emerging entities, the retrieved texts can be noisy, irrelevant, thus leading to inaccurate answers. Effectively filtering out noisy articles as well as bad answers is the key to improve extraction accuracy. Knowledge graph (KG), which contains rich, well organized information about entities, provides a good resource to address the challenge. In this work, we propose a knowledge-guided reinforcement learning (RL) framework for open attribute value extraction. Informed by relevant knowledge in KG, we trained a deep Q-network to sequentially compare extracted answers to improve extraction accuracy. The proposed framework is applicable to different information extraction system. Our experimental results show that our method outperforms the baselines by 16.5 - 27.8%.
NOTE: Video may display a random order of authors.
Correct author list is at the top of this page.
Connected Papers in EMNLP2020
Similar Papers
Adaptive Attentional Network for Few-Shot Knowledge Graph Completion
Jiawei Sheng, Shu Guo, Zhenyu Chen, Juwei Yue, Lihong Wang, Tingwen Liu, Hongbo Xu,

OpenIE6: Iterative Grid Labeling and Coordination Analysis for Open Information Extraction
Keshav Kolluru, Vaibhav Adlakha, Samarth Aggarwal, Mausam, Soumen Chakrabarti,
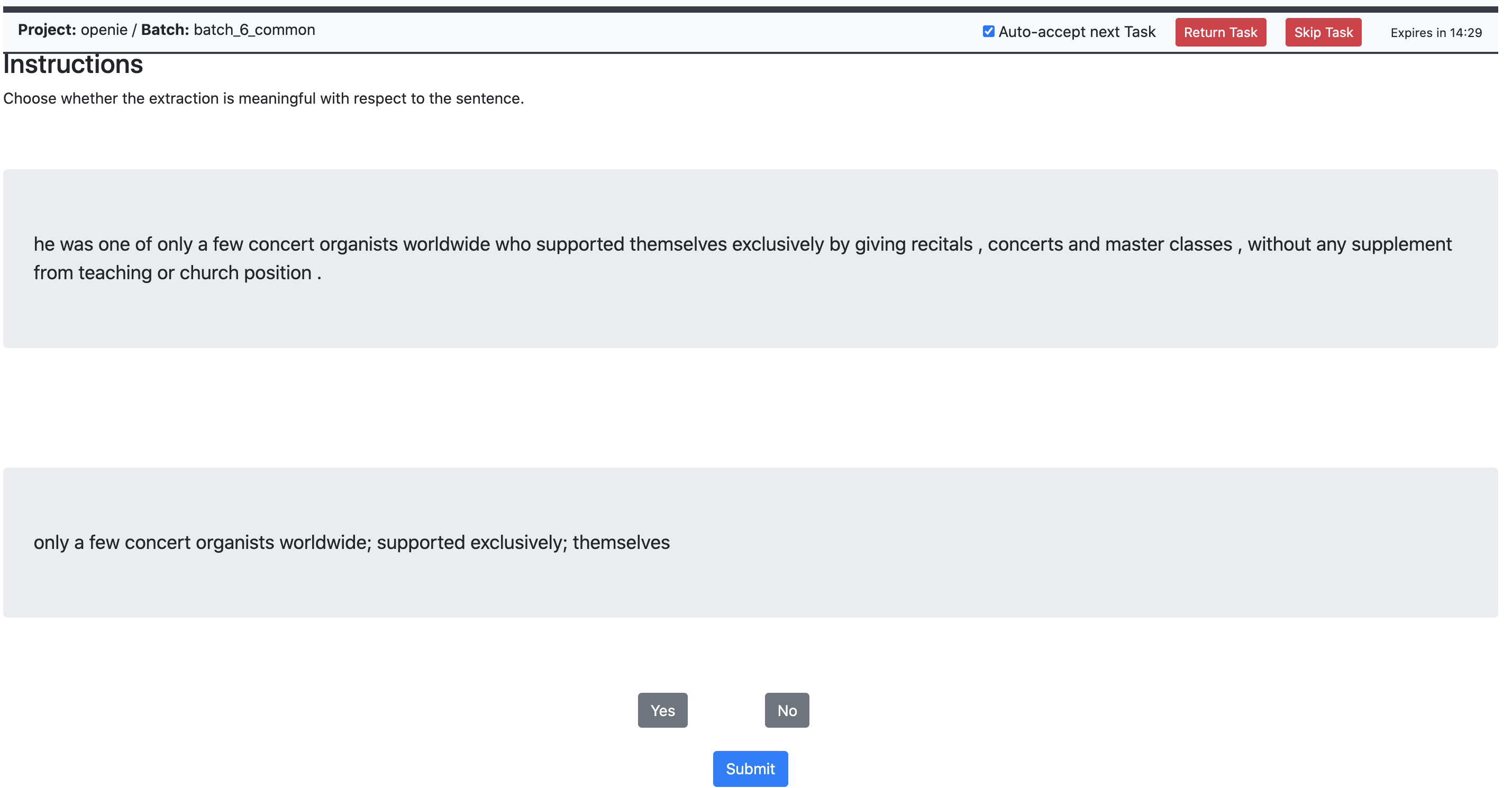
Systematic Comparison of Neural Architectures and Training Approaches for Open Information Extraction
Patrick Hohenecker, Frank Mtumbuka, Vid Kocijan, Thomas Lukasiewicz,

Learning from Context or Names? An Empirical Study on Neural Relation Extraction
Hao Peng, Tianyu Gao, Xu Han, Yankai Lin, Peng Li, Zhiyuan Liu, Maosong Sun, Jie Zhou,
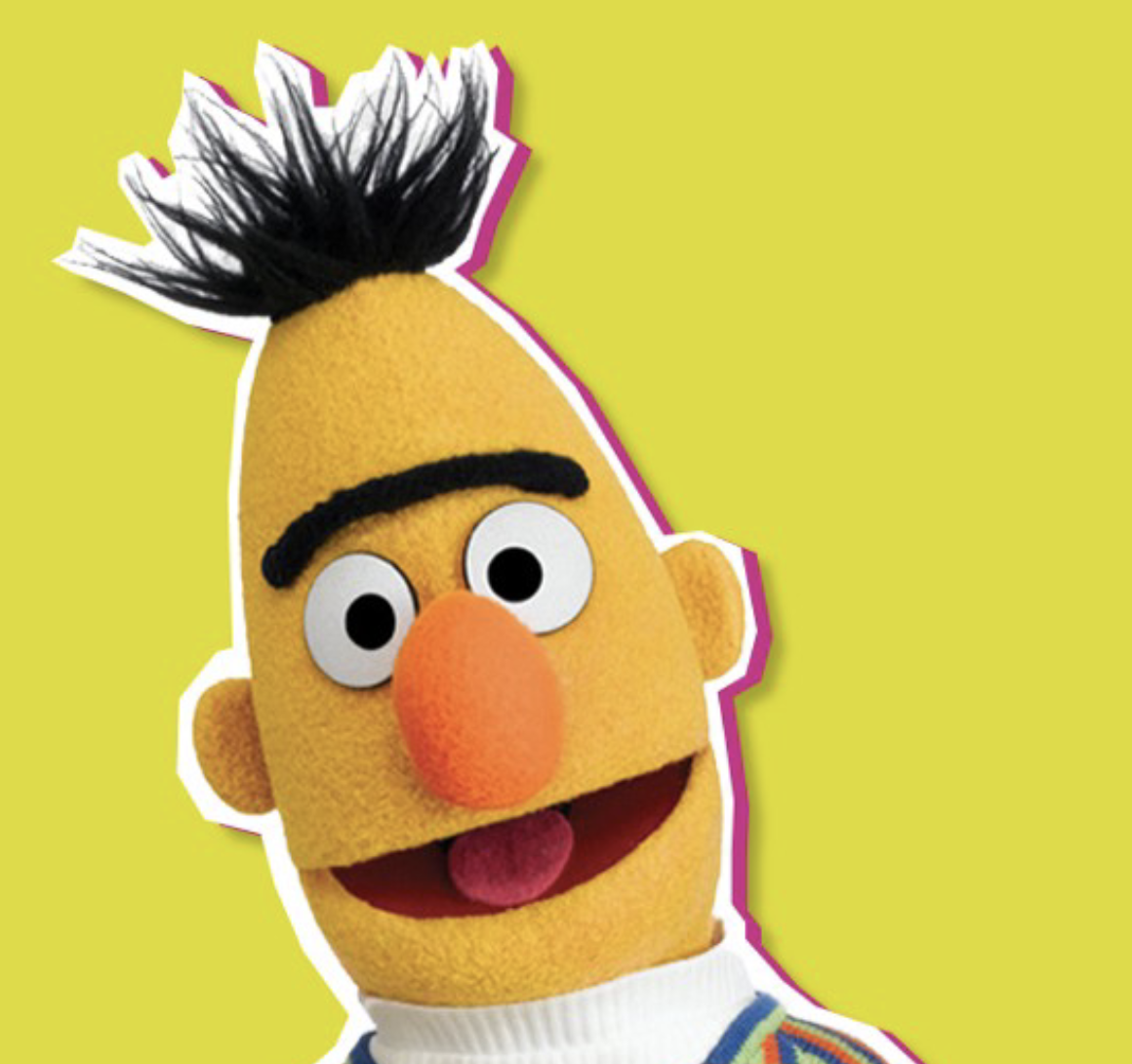