Stepwise Extractive Summarization and Planning with Structured Transformers
Shashi Narayan, Joshua Maynez, Jakub Adamek, Daniele Pighin, Blaz Bratanic, Ryan McDonald
Summarization Long Paper
You can open the pre-recorded video in a separate window.
Abstract:
We propose encoder-centric stepwise models for extractive summarization using structured transformers -- HiBERT and Extended Transformers. We enable stepwise summarization by injecting the previously generated summary into the structured transformer as an auxiliary sub-structure. Our models are not only efficient in modeling the structure of long inputs, but they also do not rely on task-specific redundancy-aware modeling, making them a general purpose extractive content planner for different tasks. When evaluated on CNN/DailyMail extractive summarization, stepwise models achieve state-of-the-art performance in terms of Rouge without any redundancy aware modeling or sentence filtering. This also holds true for Rotowire table-to-text generation, where our models surpass previously reported metrics for content selection, planning and ordering, highlighting the strength of stepwise modeling. Amongst the two structured transformers we test, stepwise Extended Transformers provides the best performance across both datasets and sets a new standard for these challenges.
NOTE: Video may display a random order of authors.
Correct author list is at the top of this page.
Connected Papers in EMNLP2020
Similar Papers
On Extractive and Abstractive Neural Document Summarization with Transformer Language Models
Jonathan Pilault, Raymond Li, Sandeep Subramanian, Chris Pal,
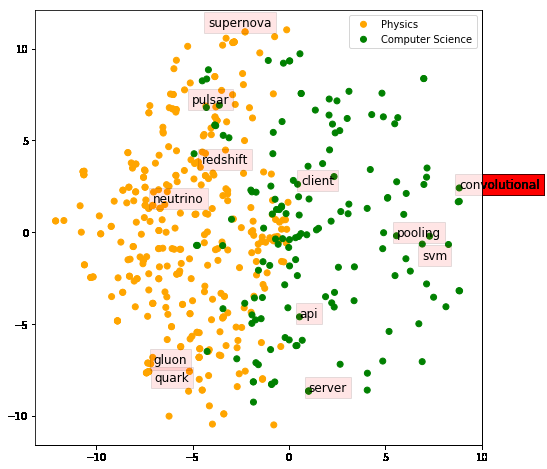
Neural Extractive Summarization with Hierarchical Attentive Heterogeneous Graph Network
Ruipeng Jia, Yanan Cao, Hengzhu Tang, Fang Fang, Cong Cao, Shi Wang,
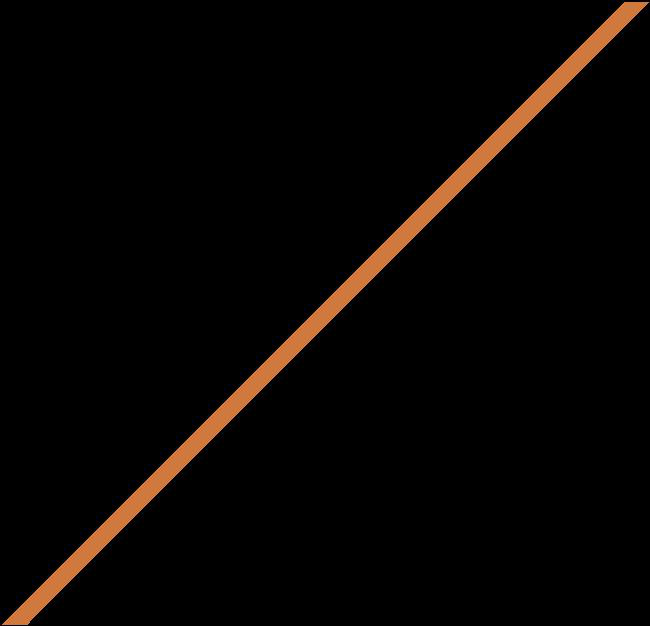
ETC: Encoding Long and Structured Inputs in Transformers
Joshua Ainslie, Santiago Ontanon, Chris Alberti, Vaclav Cvicek, Zachary Fisher, Philip Pham, Anirudh Ravula, Sumit Sanghai, Qifan Wang, Li Yang,
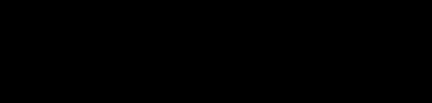