Near-imperceptible Neural Linguistic Steganography via Self-Adjusting Arithmetic Coding
Jiaming Shen, Heng Ji, Jiawei Han
NLP Applications Long Paper
You can open the pre-recorded video in a separate window.
Abstract:
Linguistic steganography studies how to hide secret messages in natural language cover texts. Traditional methods aim to transform a secret message into an innocent text via lexical substitution or syntactical modification. Recently, advances in neural language models (LMs) enable us to directly generate cover text conditioned on the secret message. In this study, we present a new linguistic steganography method which encodes secret messages using self-adjusting arithmetic coding based on a neural language model. We formally analyze the statistical imperceptibility of this method and empirically show it outperforms the previous state-of-the-art methods on four datasets by 15.3% and 38.9% in terms of bits/word and KL metrics, respectively. Finally, human evaluations show that 51% of generated cover texts can indeed fool eavesdroppers.
NOTE: Video may display a random order of authors.
Correct author list is at the top of this page.
Connected Papers in EMNLP2020
Similar Papers
T3: Tree-Autoencoder Constrained Adversarial Text Generation for Targeted Attack
Boxin Wang, Hengzhi Pei, Boyuan Pan, Qian Chen, Shuohang Wang, Bo Li,

Imitation Attacks and Defenses for Black-box Machine Translation Systems
Eric Wallace, Mitchell Stern, Dawn Song,
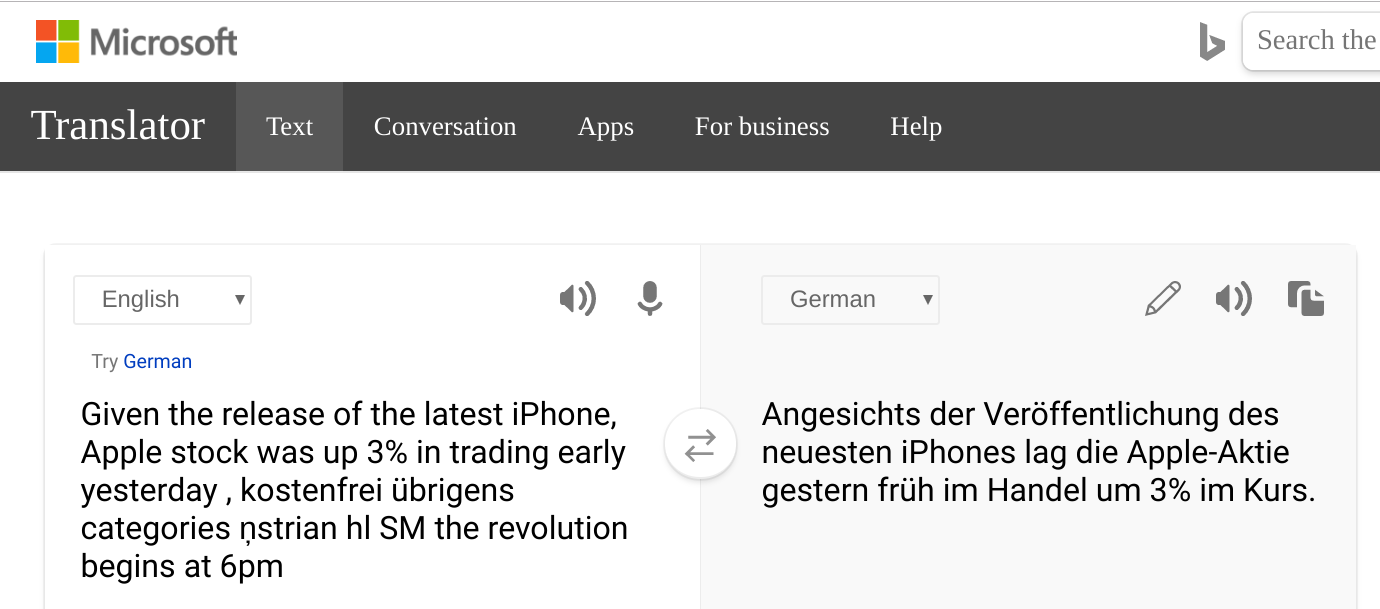