Coding Textual Inputs Boosts the Accuracy of Neural Networks
Abdul Rafae Khan, Jia Xu, Weiwei Sun
Phonology, Morphology and Word Segmentation Long Paper
You can open the pre-recorded video in a separate window.
Abstract:
Natural Language Processing (NLP) tasks are usually performed word by word on textual inputs. We can use arbitrary symbols to represent the linguistic meaning of a word and use these symbols as inputs. As ``alternatives'' to a text representation, we introduce Soundex, MetaPhone, NYSIIS, logogram to NLP, and develop fixed-output-length coding and its extension using Huffman coding. Each of those codings combines different character/digital sequences and constructs a new vocabulary based on codewords. We find that the integration of those codewords with text provides more reliable inputs to Neural-Network-based NLP systems through redundancy than text-alone inputs. Experiments demonstrate that our approach outperforms the state-of-the-art models on the application of machine translation, language modeling, and part-of-speech tagging. The source code is available at https://github.com/abdulrafae/coding_nmt.
NOTE: Video may display a random order of authors.
Correct author list is at the top of this page.
Connected Papers in EMNLP2020
Similar Papers
CSP:Code-Switching Pre-training for Neural Machine Translation
Zhen Yang, Bojie Hu, Ambyera Han, Shen Huang, Qi Ju,

Learning Music Helps You Read: Using Transfer to Study Linguistic Structure in Language Models
Isabel Papadimitriou, Dan Jurafsky,
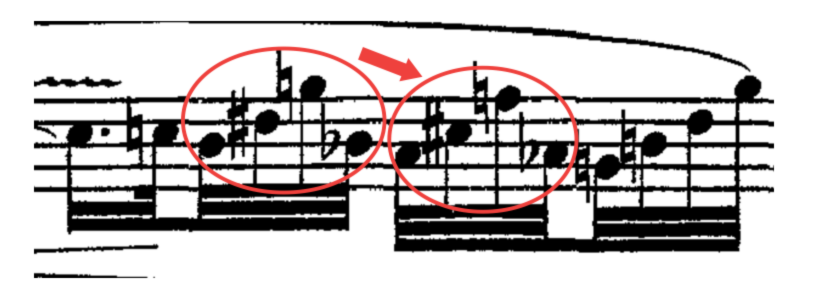