Learning Adaptive Segmentation Policy for Simultaneous Translation
Ruiqing Zhang, Chuanqiang Zhang, Zhongjun He, Hua Wu, Haifeng Wang
Machine Translation and Multilinguality Long Paper
You can open the pre-recorded video in a separate window.
Abstract:
Balancing accuracy and latency is a great challenge for simultaneous translation. To achieve high accuracy, the model usually needs to wait for more streaming text before translation, which results in increased latency. However, keeping low latency would probably hurt accuracy. Therefore, it is essential to segment the ASR output into appropriate units for translation. Inspired by human interpreters, we propose a novel adaptive segmentation policy for simultaneous translation. The policy learns to segment the source text by considering possible translations produced by the translation model, maintaining consistency between the segmentation and translation. Experimental results on Chinese-English and German-English translation show that our method achieves a better accuracy-latency trade-off over recently proposed state-of-the-art methods.
NOTE: Video may display a random order of authors.
Correct author list is at the top of this page.
Connected Papers in EMNLP2020
Similar Papers
Simultaneous Machine Translation with Visual Context
Ozan Caglayan, Julia Ive, Veneta Haralampieva, Pranava Madhyastha, Loïc Barrault, Lucia Specia,
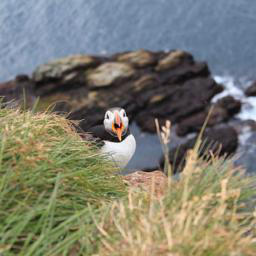
SIMULEVAL: An Evaluation Toolkit for Simultaneous Translation
Xutai Ma, Mohammad Javad Dousti, Changhan Wang, Jiatao Gu, Juan Pino,
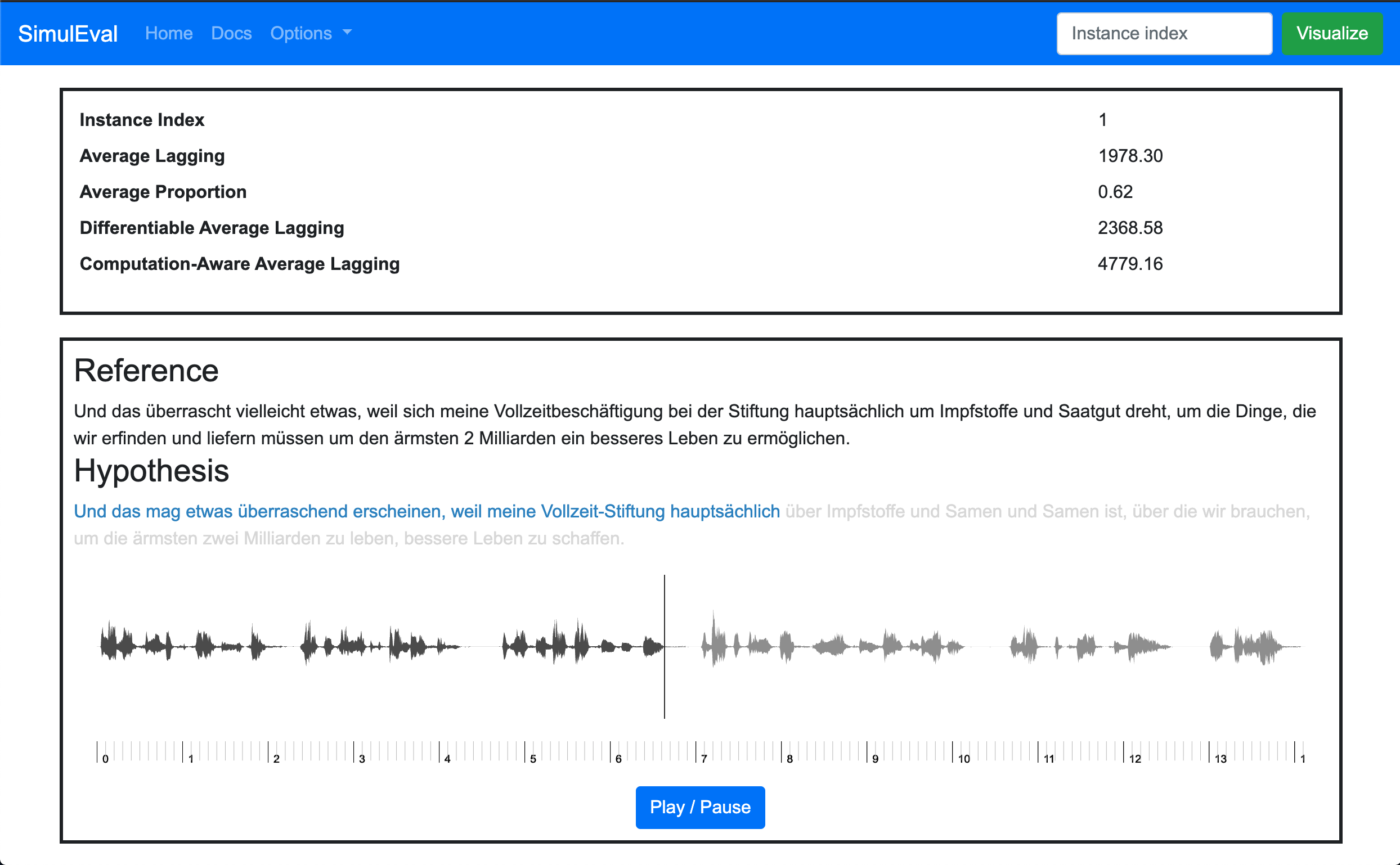
Dynamic Data Selection and Weighting for Iterative Back-Translation
Zi-Yi Dou, Antonios Anastasopoulos, Graham Neubig,
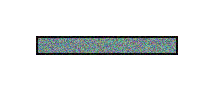
Incorporating a Local Translation Mechanism into Non-autoregressive Translation
Xiang Kong, Zhisong Zhang, Eduard Hovy,
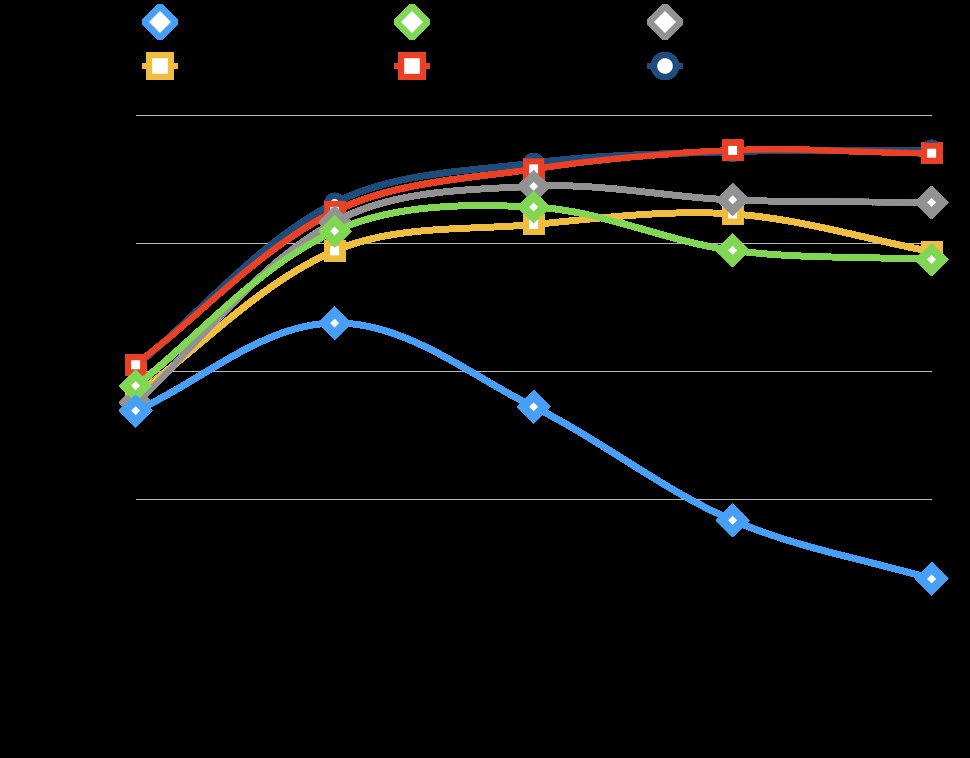