Dynamic Data Selection and Weighting for Iterative Back-Translation
Zi-Yi Dou, Antonios Anastasopoulos, Graham Neubig
Machine Translation and Multilinguality Long Paper
You can open the pre-recorded video in a separate window.
Abstract:
Back-translation has proven to be an effective method to utilize monolingual data in neural machine translation (NMT), and iteratively conducting back-translation can further improve the model performance. Selecting which monolingual data to back-translate is crucial, as we require that the resulting synthetic data are of high quality and reflect the target domain. To achieve these two goals, data selection and weighting strategies have been proposed, with a common practice being to select samples close to the target domain but also dissimilar to the average general-domain text. In this paper, we provide insights into this commonly used approach and generalize it to a dynamic curriculum learning strategy, which is applied to iterative back-translation models. In addition, we propose weighting strategies based on both the current quality of the sentence and its improvement over the previous iteration. We evaluate our models on domain adaptation, low-resource, and high-resource MT settings and on two language pairs. Experimental results demonstrate that our methods achieve improvements of up to~1.8 BLEU points over competitive baselines.
NOTE: Video may display a random order of authors.
Correct author list is at the top of this page.
Connected Papers in EMNLP2020
Similar Papers
Pronoun-Targeted Fine-tuning for NMT with Hybrid Losses
Prathyusha Jwalapuram, Shafiq Joty, Youlin Shen,

Pre-training Multilingual Neural Machine Translation by Leveraging Alignment Information
Zehui Lin, Xiao Pan, Mingxuan Wang, Xipeng Qiu, Jiangtao Feng, Hao Zhou, Lei Li,

Multi-task Learning for Multilingual Neural Machine Translation
Yiren Wang, ChengXiang Zhai, Hany Hassan,
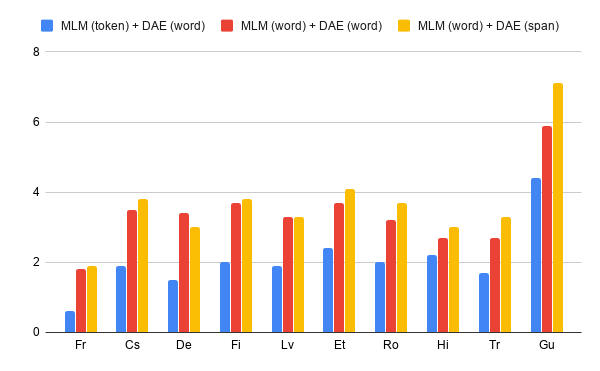
Dynamic Context Selection for Document-level Neural Machine Translation via Reinforcement Learning
Xiaomian Kang, Yang Zhao, Jiajun Zhang, Chengqing Zong,
