The World is Not Binary: Learning to Rank with Grayscale Data for Dialogue Response Selection
Zibo Lin, Deng Cai, Yan Wang, Xiaojiang Liu, Haitao Zheng, Shuming Shi
Dialog and Interactive Systems Long Paper
You can open the pre-recorded video in a separate window.
Abstract:
Response selection plays a vital role in building retrieval-based conversation systems. Despite that response selection is naturally a learning-to-rank problem, most prior works take a point-wise view and train binary classifiers for this task: each response candidate is labeled either relevant (one) or irrelevant (zero). On the one hand, this formalization can be sub-optimal due to its ignorance of the diversity of response quality. On the other hand, annotating grayscale data for learning-to-rank can be prohibitively expensive and challenging. In this work, we show that grayscale data can be automatically constructed without human effort. Our method employs off-the-shelf response retrieval models and response generation models as automatic grayscale data generators. With the constructed grayscale data, we propose multi-level ranking objectives for training, which can (1) teach a matching model to capture more fine-grained context-response relevance difference and (2) reduce the train-test discrepancy in terms of distractor strength. Our method is simple, effective, and universal. Experiments on three benchmark datasets and four state-of-the-art matching models show that the proposed approach brings significant and consistent performance improvements.
NOTE: Video may display a random order of authors.
Correct author list is at the top of this page.
Connected Papers in EMNLP2020
Similar Papers
Dialogue Response Ranking Training with Large-Scale Human Feedback Data
Xiang Gao, Yizhe Zhang, Michel Galley, Chris Brockett, Bill Dolan,
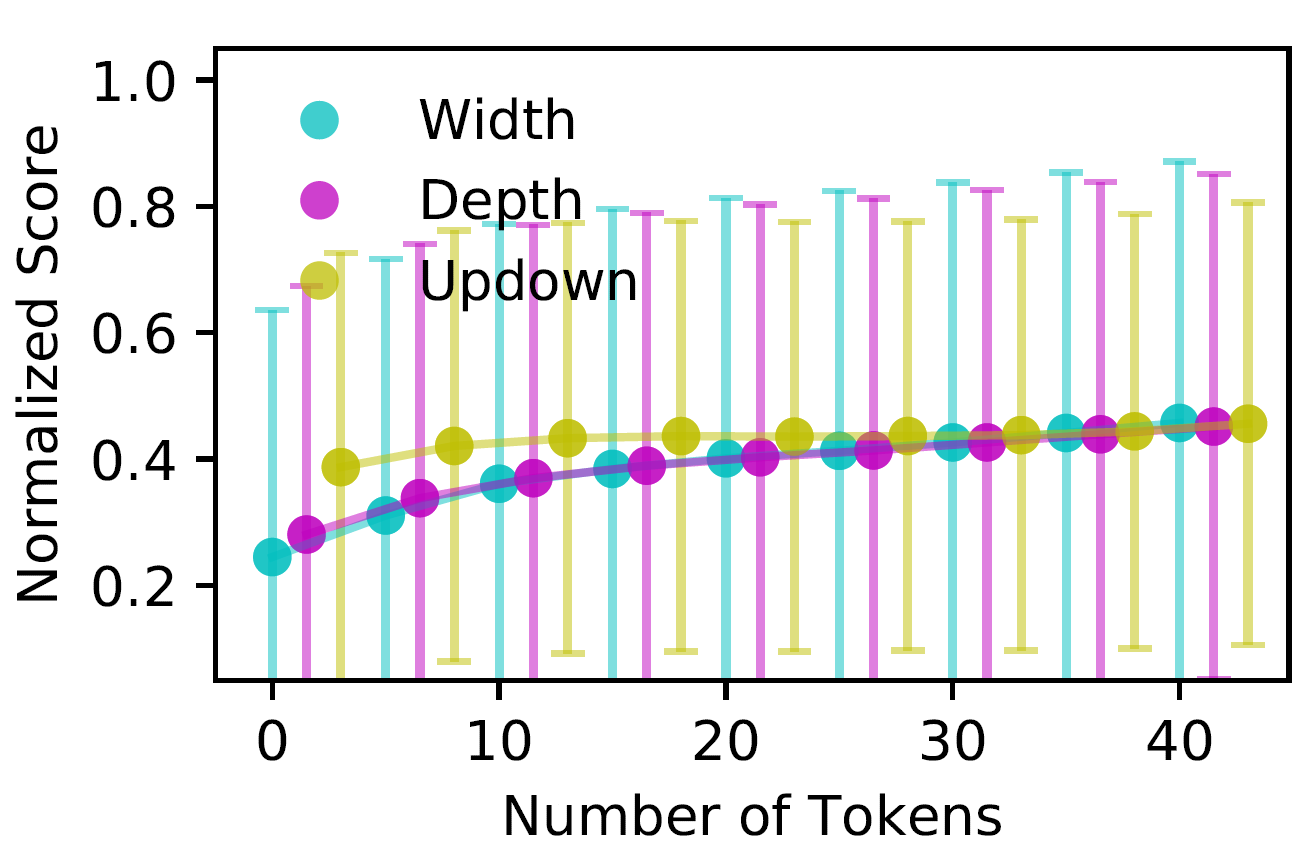
Q-learning with Language Model for Edit-based Unsupervised Summarization
Ryosuke Kohita, Akifumi Wachi, Yang Zhao, Ryuki Tachibana,

MUTANT: A Training Paradigm for Out-of-Distribution Generalization in Visual Question Answering
Tejas Gokhale, Pratyay Banerjee, Chitta Baral, Yezhou Yang,
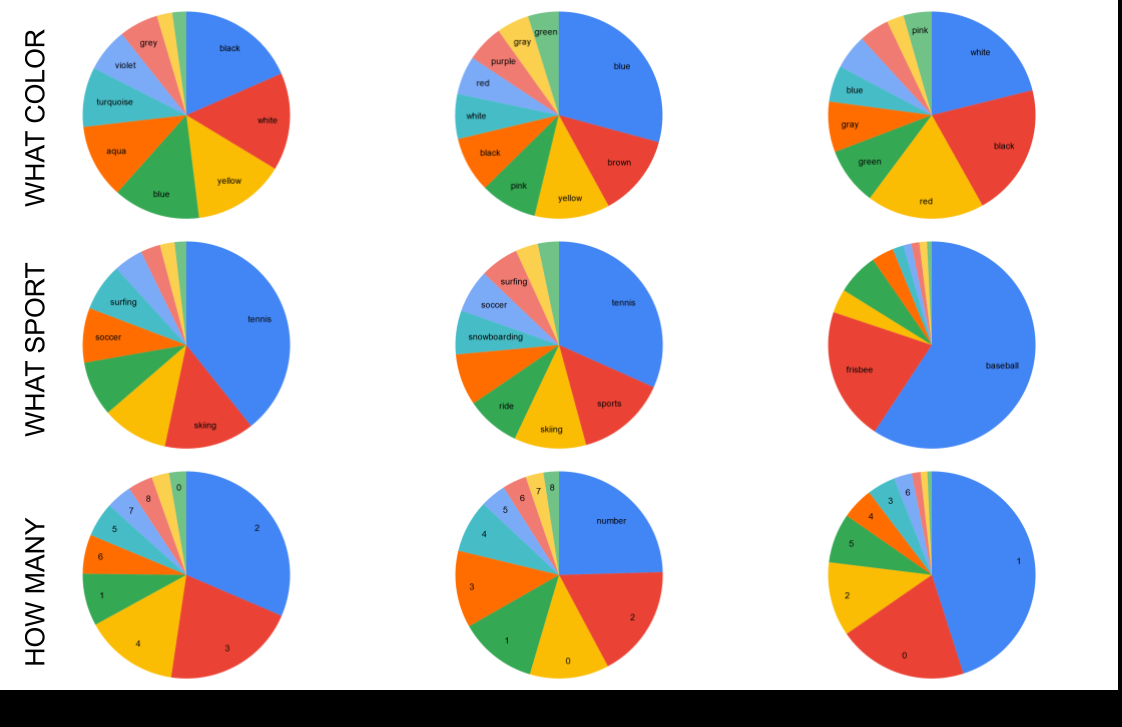
Generating Dialogue Responses from a Semantic Latent Space
Wei-Jen Ko, Avik Ray, Yilin Shen, Hongxia Jin,
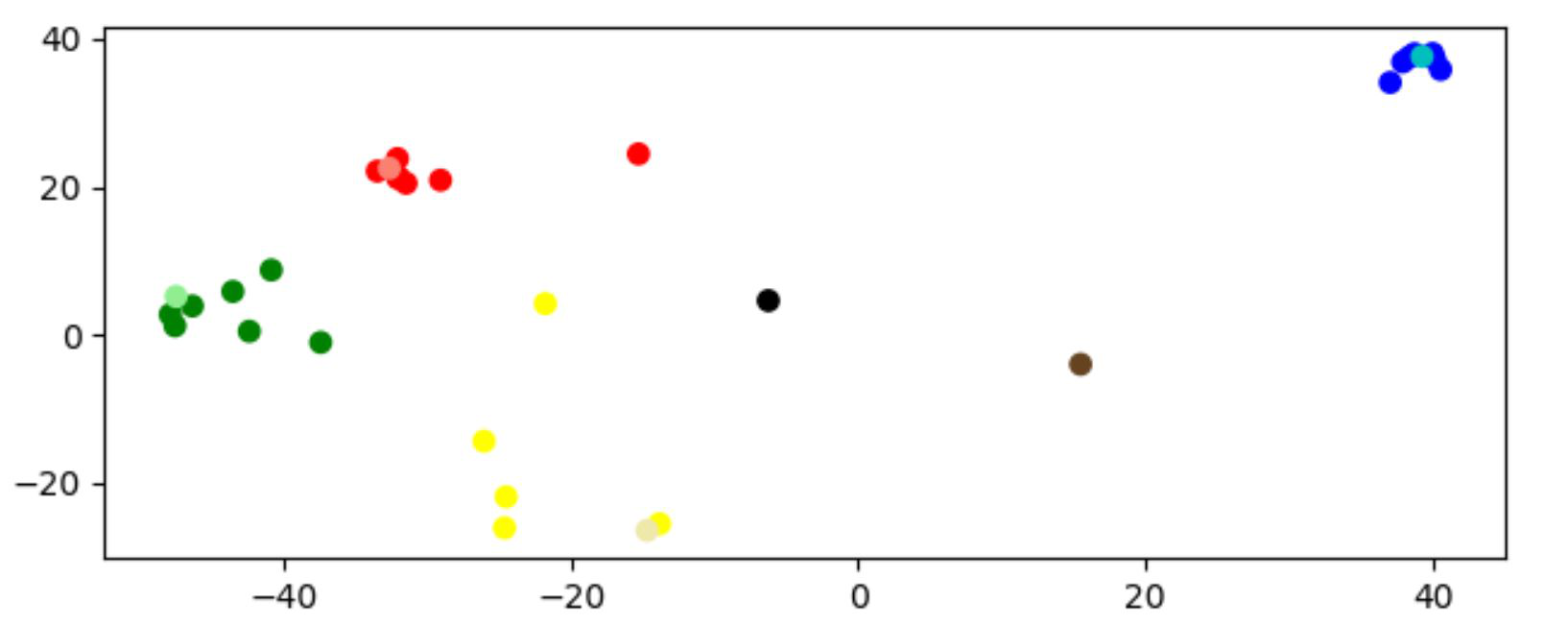