Calibrated Language Model Fine-Tuning for In- and Out-of-Distribution Data
Lingkai Kong, Haoming Jiang, Yuchen Zhuang, Jie Lyu, Tuo Zhao, Chao Zhang
Machine Learning for NLP Long Paper
You can open the pre-recorded video in a separate window.
Abstract:
Fine-tuned pre-trained language models can suffer from severe miscalibration for both in-distribution and out-of-distribution (OOD) data due to over-parameterization. To mitigate this issue, we propose a regularized fine-tuning method. Our method introduces two types of regularization for better calibration: (1) On-manifold regularization, which generates pseudo on-manifold samples through interpolation within the data manifold. Augmented training with these pseudo samples imposes a smoothness regularization to improve in-distribution calibration. (2) Off-manifold regularization, which encourages the model to output uniform distributions for pseudo off-manifold samples to address the over-confidence issue for OOD data. Our experiments demonstrate that the proposed method outperforms existing calibration methods for text classification in terms of expectation calibration error, misclassification detection, and OOD detection on six datasets. Our code can be found at https://github.com/Lingkai-Kong/Calibrated-BERT-Fine-Tuning.
NOTE: Video may display a random order of authors.
Correct author list is at the top of this page.
Connected Papers in EMNLP2020
Similar Papers
Feature Adaptation of Pre-Trained Language Models across Languages and Domains with Robust Self-Training
Hai Ye, Qingyu Tan, Ruidan He, Juntao Li, Hwee Tou Ng, Lidong Bing,

Discriminatively-Tuned Generative Classifiers for Robust Natural Language Inference
Xiaoan Ding, Tianyu Liu, Baobao Chang, Zhifang Sui, Kevin Gimpel,

Self-Supervised Meta-Learning for Few-Shot Natural Language Classification Tasks
Trapit Bansal, Rishikesh Jha, Tsendsuren Munkhdalai, Andrew McCallum,
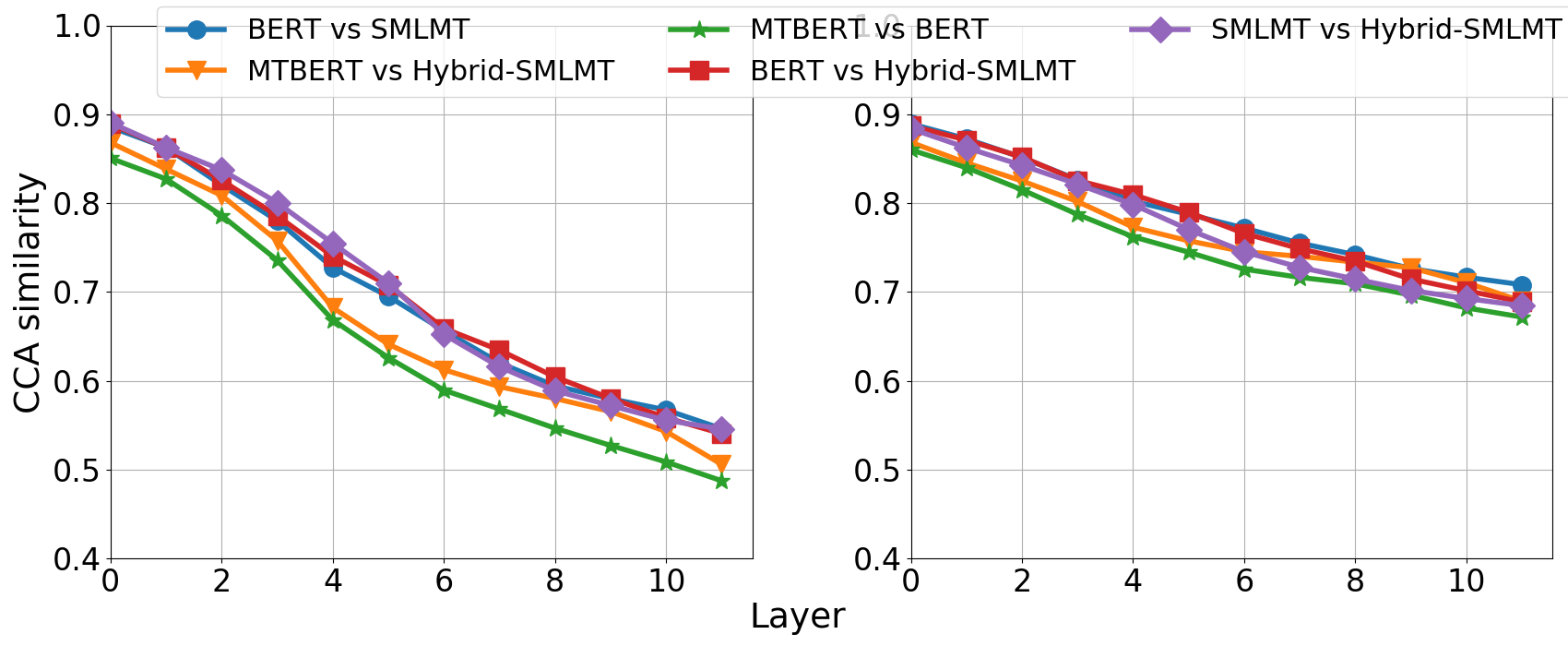
Local Additivity Based Data Augmentation for Semi-supervised NER
Jiaao Chen, Zhenghui Wang, Ran Tian, Zichao Yang, Diyi Yang,
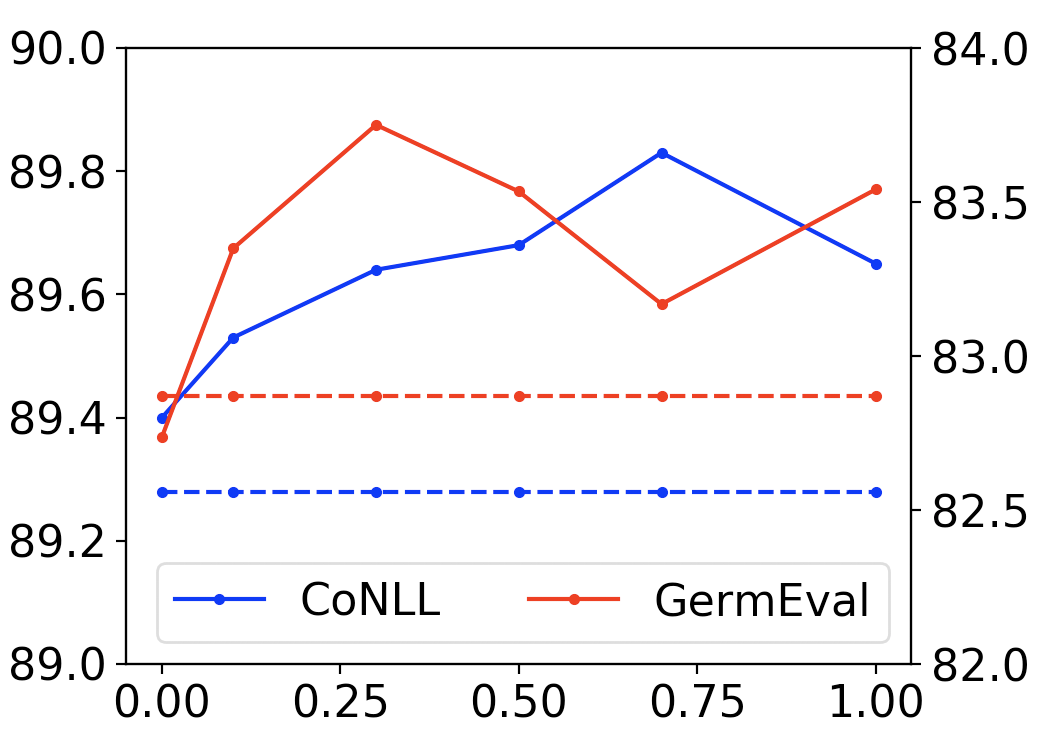