Self-Supervised Meta-Learning for Few-Shot Natural Language Classification Tasks
Trapit Bansal, Rishikesh Jha, Tsendsuren Munkhdalai, Andrew McCallum
Machine Learning for NLP Long Paper
You can open the pre-recorded video in a separate window.
Abstract:
Self-supervised pre-training of transformer models has revolutionized NLP applications. Such pre-training with language modeling objectives provides a useful initial point for parameters that generalize well to new tasks with fine-tuning. However, fine-tuning is still data inefficient --- when there are few labeled examples, accuracy can be low. Data efficiency can be improved by optimizing pre-training directly for future fine-tuning with few examples; this can be treated as a meta-learning problem. However, standard meta-learning techniques require many training tasks in order to generalize; unfortunately, finding a diverse set of such supervised tasks is usually difficult. This paper proposes a self-supervised approach to generate a large, rich, meta-learning task distribution from unlabeled text. This is achieved using a cloze-style objective, but creating separate multi-class classification tasks by gathering tokens-to-be blanked from among only a handful of vocabulary terms. This yields as many unique meta-training tasks as the number of subsets of vocabulary terms. We meta-train a transformer model on this distribution of tasks using a recent meta-learning framework. On 17 NLP tasks, we show that this meta-training leads to better few-shot generalization than language-model pre-training followed by finetuning. Furthermore, we show how the self-supervised tasks can be combined with supervised tasks for meta-learning, providing substantial accuracy gains over previous supervised meta-learning.
NOTE: Video may display a random order of authors.
Correct author list is at the top of this page.
Connected Papers in EMNLP2020
Similar Papers
Zero-Shot Cross-Lingual Transfer with Meta Learning
Farhad Nooralahzadeh, Giannis Bekoulis, Johannes Bjerva, Isabelle Augenstein,
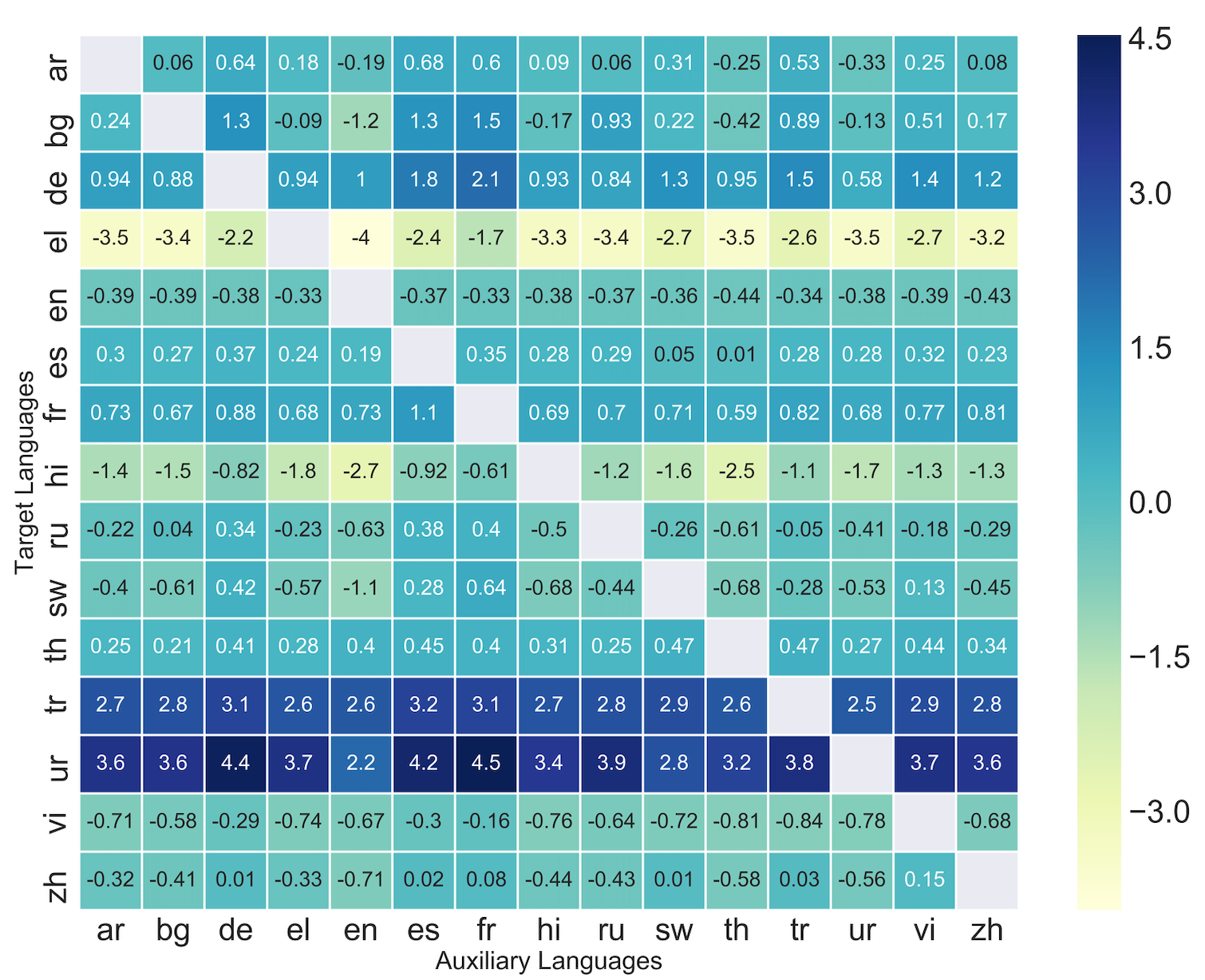
Cold-start Active Learning through Self-supervised Language Modeling
Michelle Yuan, Hsuan-Tien Lin, Jordan Boyd-Graber,

KGPT: Knowledge-Grounded Pre-Training for Data-to-Text Generation
Wenhu Chen, Yu Su, Xifeng Yan, William Yang Wang,
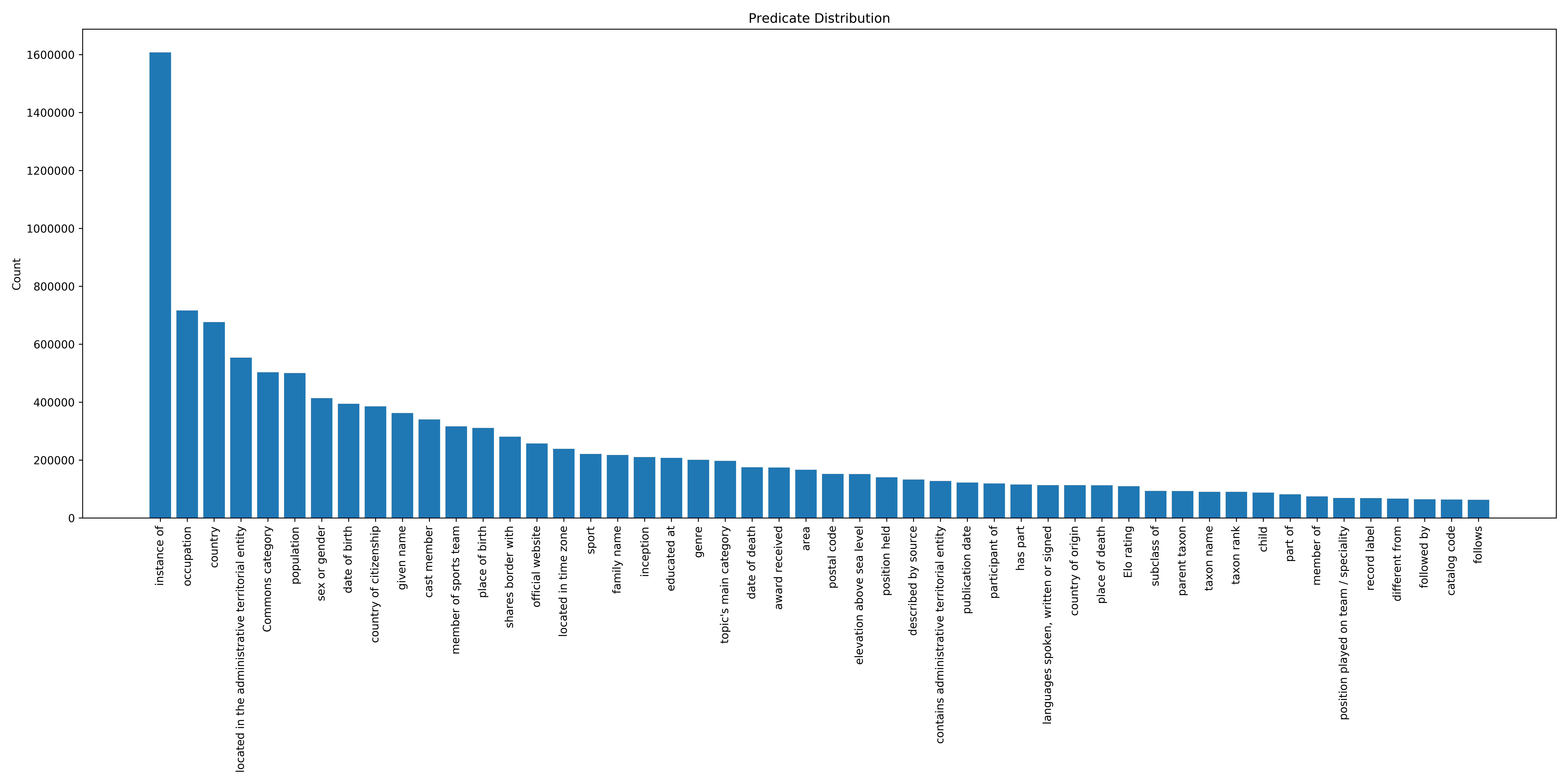
Learning Which Features Matter: RoBERTa Acquires a Preference for Linguistic Generalizations (Eventually)
Alex Warstadt, Yian Zhang, Xiaocheng Li, Haokun Liu, Samuel R. Bowman,
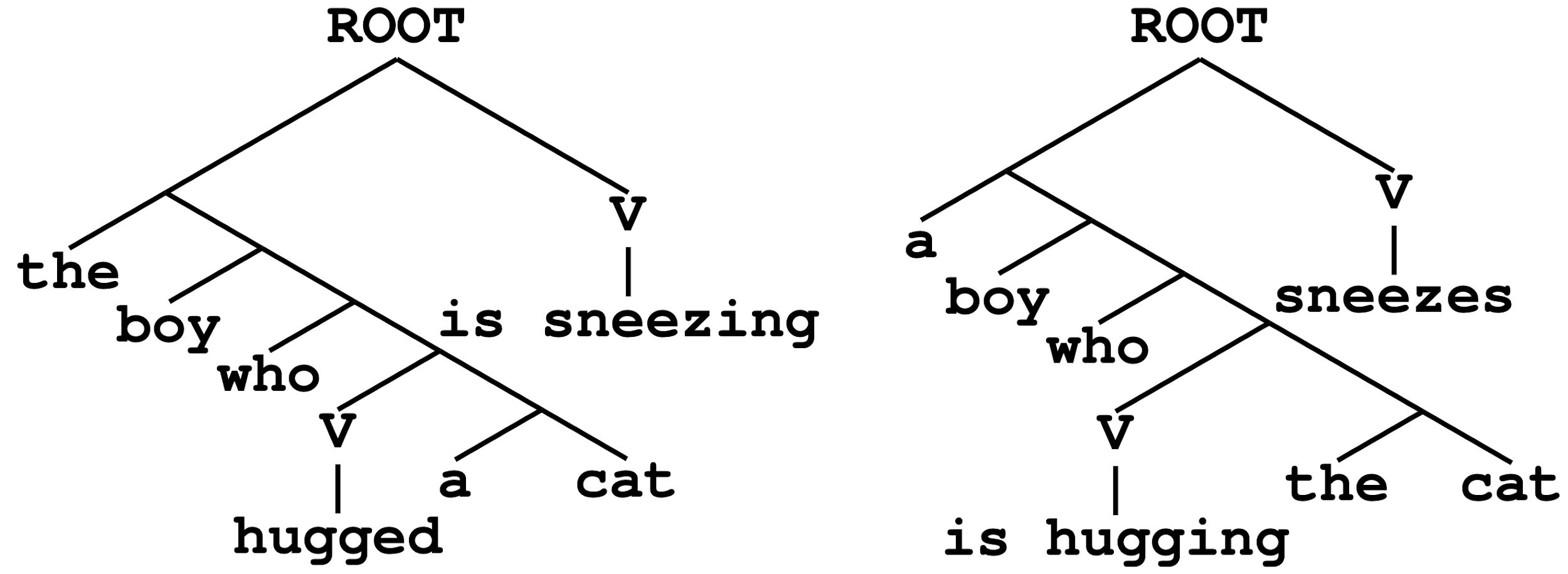