FedED: Federated Learning via Ensemble Distillation for Medical Relation Extraction
Dianbo Sui, Yubo Chen, Jun Zhao, Yantao Jia, Yuantao Xie, Weijian Sun
Information Extraction Long Paper
You can open the pre-recorded video in a separate window.
Abstract:
Unlike other domains, medical texts are inevitably accompanied by private information, so sharing or copying these texts is strictly restricted. However, training a medical relation extraction model requires collecting these privacy-sensitive texts and storing them on one machine, which comes in conflict with privacy protection. In this paper, we propose a privacy-preserving medical relation extraction model based on federated learning, which enables training a central model with no single piece of private local data being shared or exchanged. Though federated learning has distinct advantages in privacy protection, it suffers from the communication bottleneck, which is mainly caused by the need to upload cumbersome local parameters. To overcome this bottleneck, we leverage a strategy based on knowledge distillation. Such a strategy uses the uploaded predictions of ensemble local models to train the central model without requiring uploading local parameters. Experiments on three publicly available medical relation extraction datasets demonstrate the effectiveness of our method.
NOTE: Video may display a random order of authors.
Correct author list is at the top of this page.
Connected Papers in EMNLP2020
Similar Papers
COMETA: A Corpus for Medical Entity Linking in the Social Media
Marco Basaldella, Fangyu Liu, Ehsan Shareghi, Nigel Collier,

New Protocols and Negative Results for Textual Entailment Data Collection
Samuel R. Bowman, Jennimaria Palomaki, Livio Baldini Soares, Emily Pitler,

LibKGE - A knowledge graph embedding library for reproducible research
Samuel Broscheit, Daniel Ruffinelli, Adrian Kochsiek, Patrick Betz, Rainer Gemulla,

AdapterHub: A Framework for Adapting Transformers
Jonas Pfeiffer, Andreas Rücklé, Clifton Poth, Aishwarya Kamath, Ivan Vulić, Sebastian Ruder, Kyunghyun Cho, Iryna Gurevych,
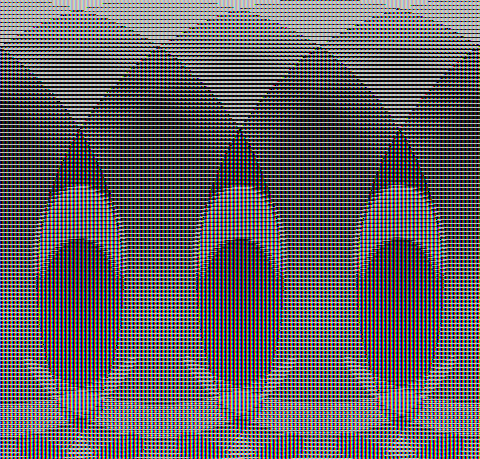