AdapterHub: A Framework for Adapting Transformers
Jonas Pfeiffer, Andreas Rücklé, Clifton Poth, Aishwarya Kamath, Ivan Vulić, Sebastian Ruder, Kyunghyun Cho, Iryna Gurevych
Demo Paper
Abstract:
The current modus operandi in NLP involves downloading and fine-tuning pre-trained models consisting of millions or billions of parameters. Storing and sharing such large trained models is expensive, slow, and time-consuming, which impedes progress towards more general and versatile NLP methods that learn from and for many tasks. Adapters---small learnt bottleneck layers inserted within each layer of a pre-trained model--- ameliorate this issue by avoiding full fine-tuning of the entire model. However, sharing and integrating adapter layers is not straightforward. We propose AdapterHub, a framework that allows dynamic "stiching-in" of pre-trained adapters for different tasks and languages. The framework, built on top of the popular HuggingFace Transformers library, enables extremely easy and quick adaptations of state-of-the-art pre-trained models (e.g., BERT, RoBERTa, XLM-R) across tasks and languages. Downloading, sharing, and training adapters is as seamless as possible using minimal changes to the training scripts and a specialized infrastructure. Our framework enables scalable and easy access to sharing of task-specific models, particularly in low-resource scenarios. AdapterHub includes all recent adapter architectures and can be found at AdapterHub.ml
Similar Papers
Why Skip If You Can Combine: A Simple Knowledge Distillation Technique for Intermediate Layers
Yimeng Wu, Peyman Passban, Mehdi Rezagholizadeh, Qun Liu,
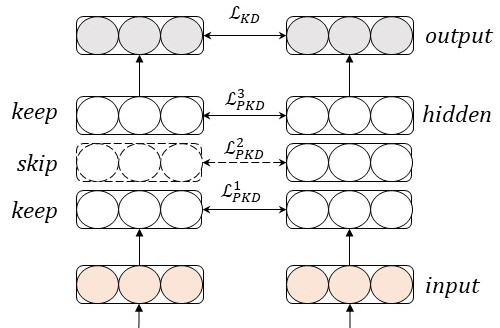
Understanding the Difficulty of Training Transformers
Liyuan Liu, Xiaodong Liu, Jianfeng Gao, Weizhu Chen, Jiawei Han,
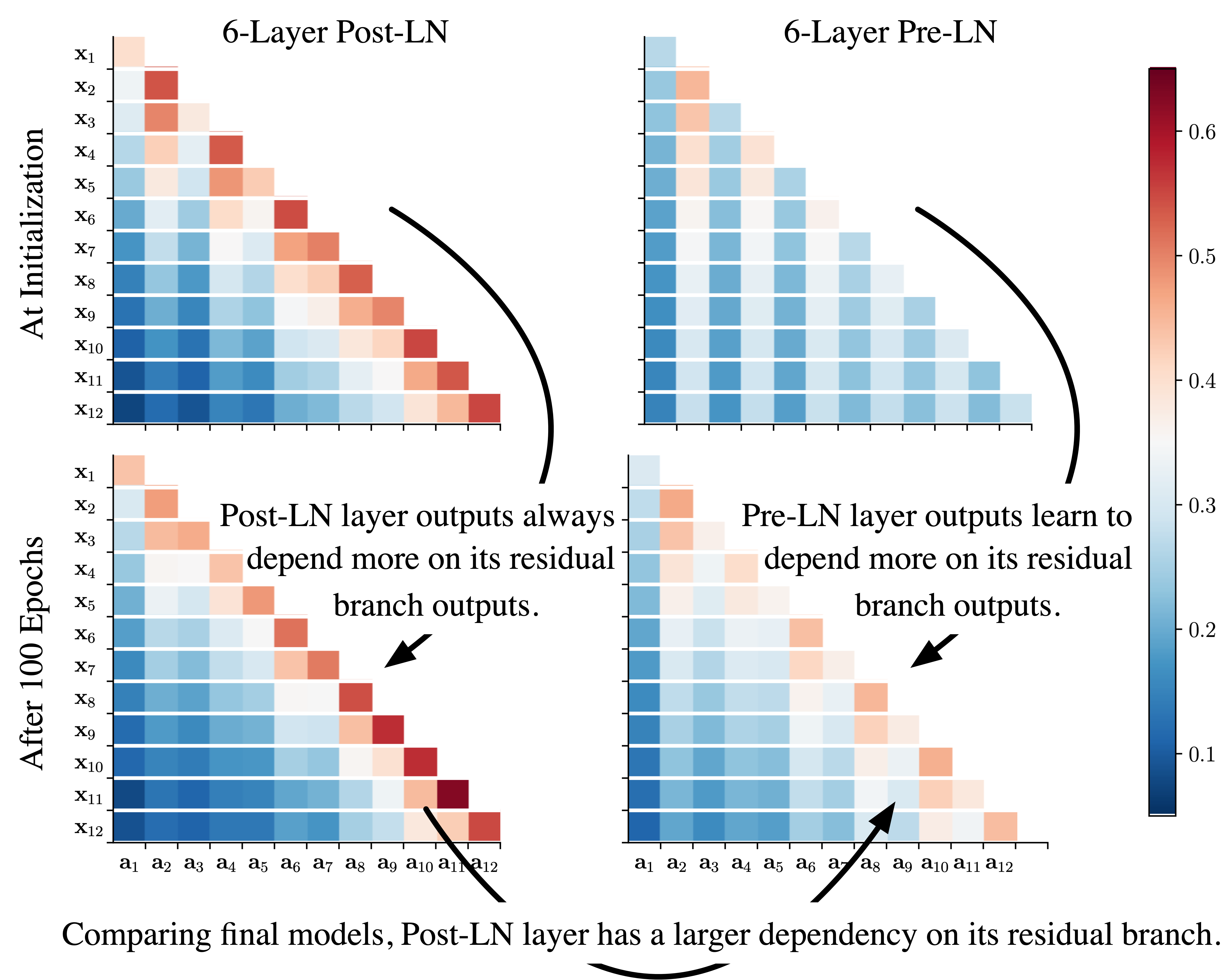
Transformers: State-of-the-Art Natural Language Processing
Thomas Wolf, Julien Chaumond, Lysandre Debut, Victor Sanh, Clement Delangue, Anthony Moi, Pierric Cistac, Morgan Funtowicz, Joe Davison, Sam Shleifer, Remi Louf, Patrick von Platen, Tim Rault, Yacine Jernite, Teven Le Scao, Sylvain Gugger, Julien Plu, Clara Ma, Canwei Shen, Mariama Drame, Quentin Lhoest, Alexander Rush,
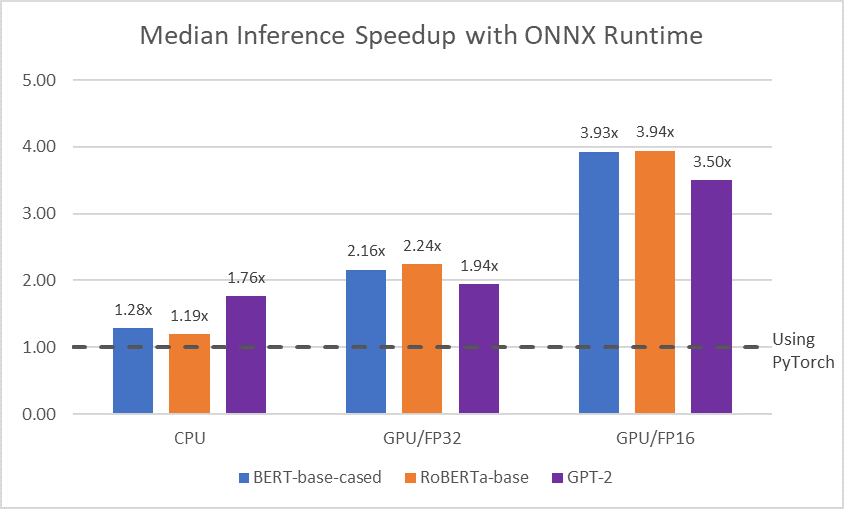
Grounded Compositional Outputs for Adaptive Language Modeling
Nikolaos Pappas, Phoebe Mulcaire, Noah A. Smith,
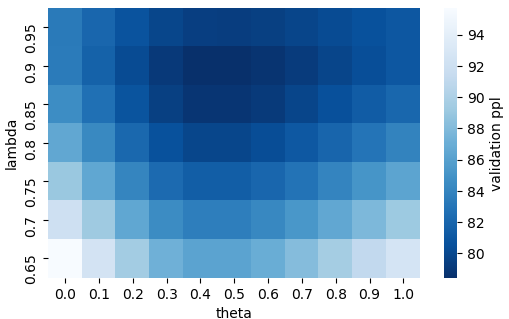