Do “Undocumented Workers” == “Illegal Aliens”? Differentiating Denotation and Connotation in Vector Spaces
Albert Webson, Zhizhong Chen, Carsten Eickhoff, Ellie Pavlick
Semantics: Lexical Semantics Long Paper
You can open the pre-recorded video in a separate window.
Abstract:
In politics, neologisms are frequently invented for partisan objectives. For example, ``undocumented workers” and ``illegal aliens” refer to the same group of people (i.e., they have the same denotation), but they carry clearly different connotations. Examples like these have traditionally posed a challenge to reference-based semantic theories and led to increasing acceptance of alternative theories (e.g., Two-Factor Semantics) among philosophers and cognitive scientists. In NLP, however, popular pretrained models encode both denotation and connotation as one entangled representation. In this study, we propose an adversarial nerual netowrk that decomposes a pretrained representation as independent denotation and connotation representations. For intrinsic interpretability, we show that words with the same denotation but different connotations (e.g., “immigrants" vs. “aliens", “estate tax" vs. “death tax") move closer to each other in denotation space while moving further apart in connotation space. For extrinsic application, we train an information retrieval system with our disentangled representations and show that the denotation vectors improve the viewpoint diversity of document rankings.
NOTE: Video may display a random order of authors.
Correct author list is at the top of this page.
Connected Papers in EMNLP2020
Similar Papers
Social Chemistry 101: Learning to Reason about Social and Moral Norms
Maxwell Forbes, Jena D. Hwang, Vered Shwartz, Maarten Sap, Yejin Choi,
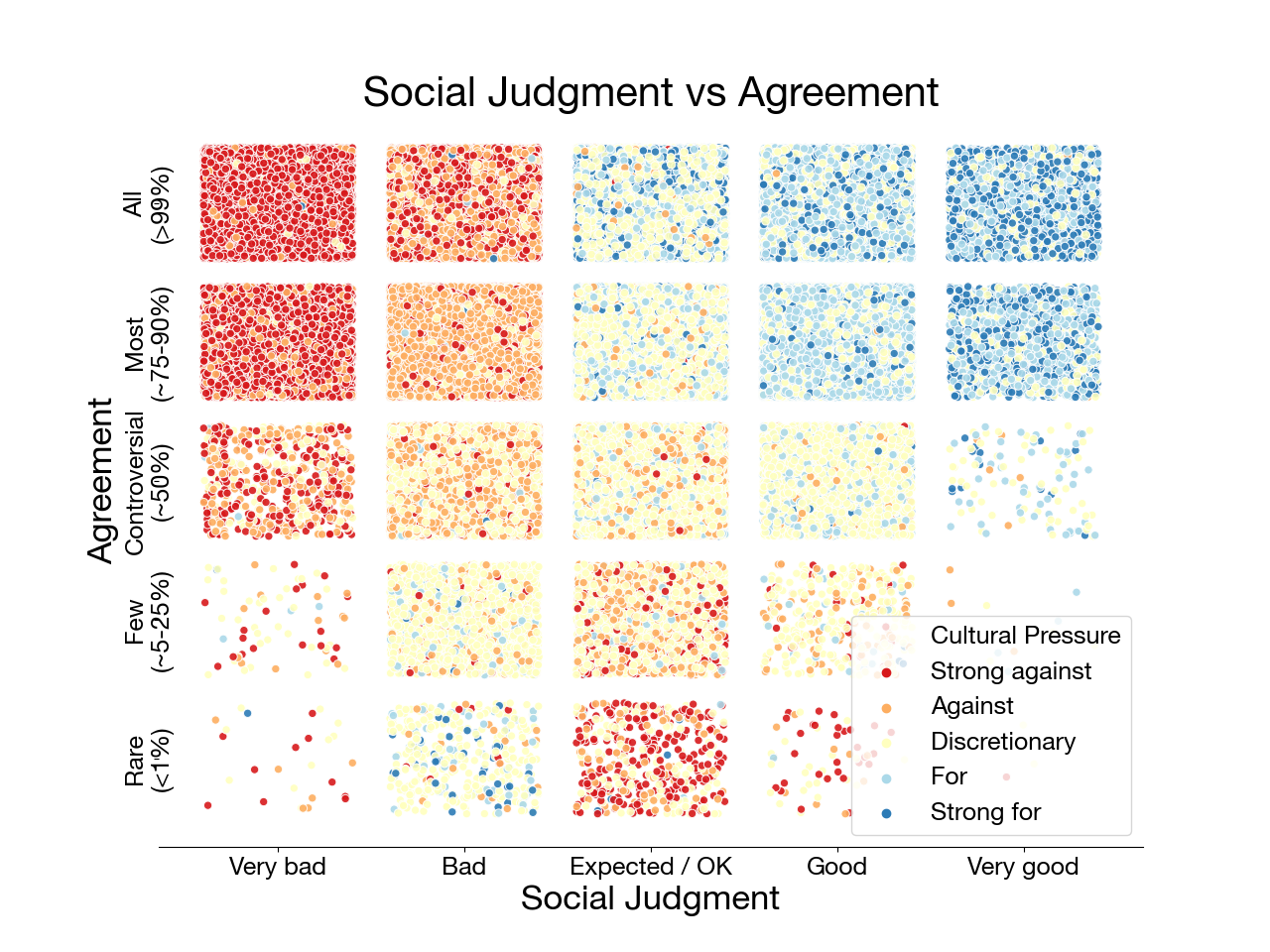
“You are grounded!”: Latent Name Artifacts in Pre-trained Language Models
Vered Shwartz, Rachel Rudinger, Oyvind Tafjord,

Nurse is Closer to Woman than Surgeon? Mitigating Gender-Biased Proximities in Word Embeddings
Vaibhav Kumar, Tenzin Bhotia, Vaibhav Kumar, Tanmoy Chakraborty,

Type B Reflexivization as an Unambiguous Testbed for Multilingual Multi-Task Gender Bias
Ana Valeria González, Maria Barrett, Rasmus Hvingelby, Kellie Webster, Anders Søgaard,
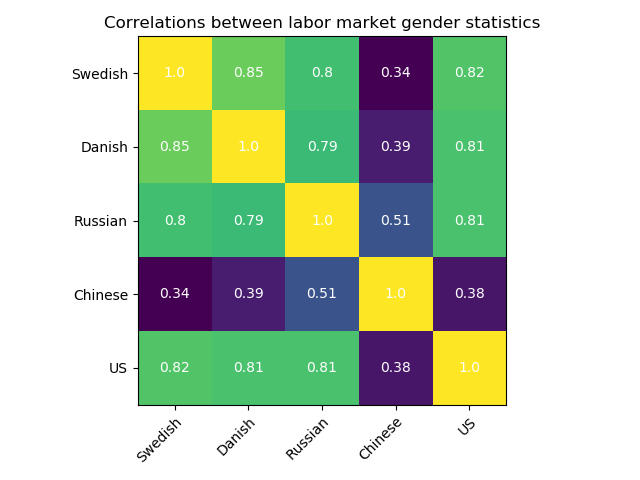