“You are grounded!”: Latent Name Artifacts in Pre-trained Language Models
Vered Shwartz, Rachel Rudinger, Oyvind Tafjord
Interpretability and Analysis of Models for NLP Short Paper
You can open the pre-recorded video in a separate window.
Abstract:
Pre-trained language models (LMs) may perpetuate biases originating in their training corpus to downstream models. We focus on artifacts associated with the representation of given names (e.g., Donald), which, depending on the corpus, may be associated with specific entities, as indicated by next token prediction (e.g., Trump). While helpful in some contexts, grounding happens also in under-specified or inappropriate contexts. For example, endings generated for `Donald is a' substantially differ from those of other names, and often have more-than-average negative sentiment. We demonstrate the potential effect on downstream tasks with reading comprehension probes where name perturbation changes the model answers. As a silver lining, our experiments suggest that additional pre-training on different corpora may mitigate this bias.
NOTE: Video may display a random order of authors.
Correct author list is at the top of this page.
Connected Papers in EMNLP2020
Similar Papers
Probing Pretrained Language Models for Lexical Semantics
Ivan Vulić, Edoardo Maria Ponti, Robert Litschko, Goran Glavaš, Anna Korhonen,
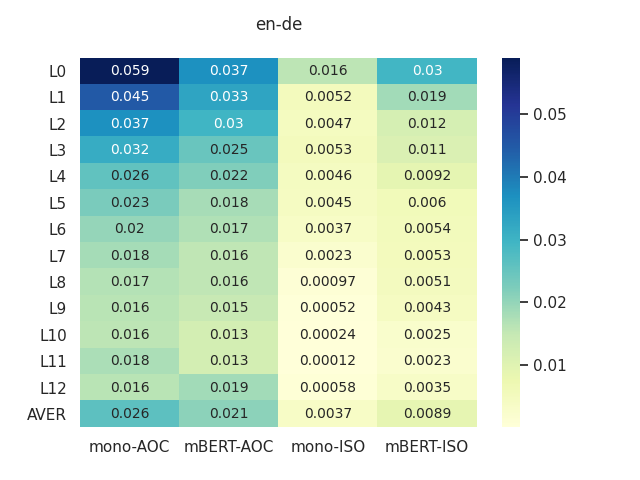
Investigating representations of verb bias in neural language models
Robert Hawkins, Takateru Yamakoshi, Thomas Griffiths, Adele Goldberg,

Asking without Telling: Exploring Latent Ontologies in Contextual Representations
Julian Michael, Jan A. Botha, Ian Tenney,
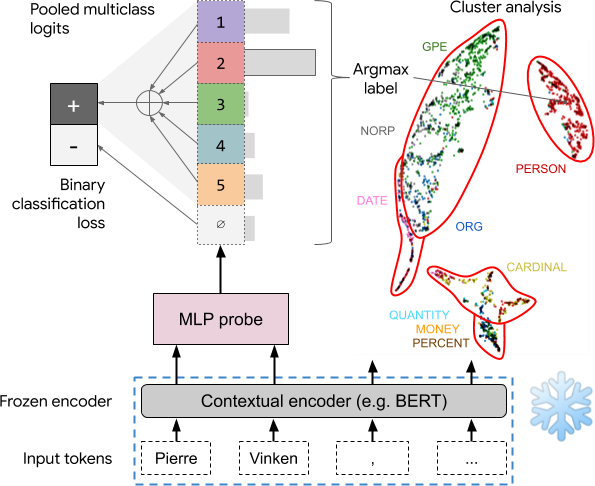
Substance over Style: Document-Level Targeted Content Transfer
Allison Hegel, Sudha Rao, Asli Celikyilmaz, Bill Dolan,
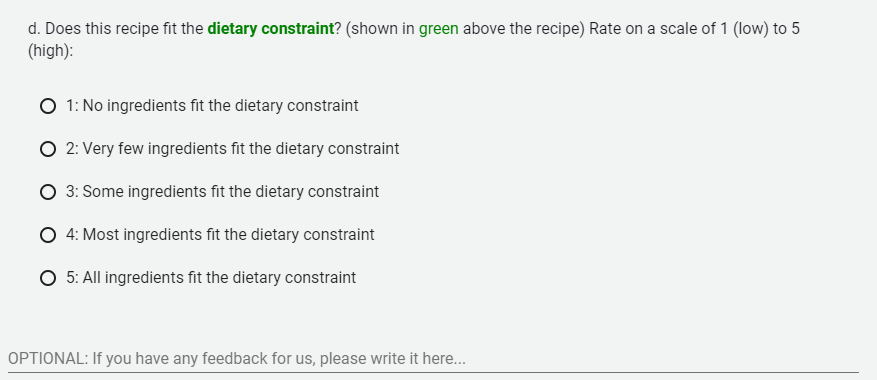