Type B Reflexivization as an Unambiguous Testbed for Multilingual Multi-Task Gender Bias
Ana Valeria González, Maria Barrett, Rasmus Hvingelby, Kellie Webster, Anders Søgaard
Interpretability and Analysis of Models for NLP Long Paper
You can open the pre-recorded video in a separate window.
Abstract:
The one-sided focus on English in previous studies of gender bias in NLP misses out on opportunities in other languages: English challenge datasets such as GAP and WinoGender highlight model preferences that are "hallucinatory", e.g., disambiguating gender-ambiguous occurrences of 'doctor' as male doctors. We show that for languages with type B reflexivization, e.g., Swedish and Russian, we can construct multi-task challenge datasets for detecting gender bias that lead to unambiguously wrong model predictions: In these languages, the direct translation of 'the doctor removed his mask' is not ambiguous between a coreferential reading and a disjoint reading. Instead, the coreferential reading requires a non-gendered pronoun, and the gendered, possessive pronouns are anti-reflexive. We present a multilingual, multi-task challenge dataset, which spans four languages and four NLP tasks and focuses only on this phenomenon. We find evidence for gender bias across all task-language combinations and correlate model bias with national labor market statistics.
NOTE: Video may display a random order of authors.
Correct author list is at the top of this page.
Connected Papers in EMNLP2020
Similar Papers
LAReQA: Language-Agnostic Answer Retrieval from a Multilingual Pool
Uma Roy, Noah Constant, Rami Al-Rfou, Aditya Barua, Aaron Phillips, Yinfei Yang,

XCOPA: A Multilingual Dataset for Causal Commonsense Reasoning
Edoardo Maria Ponti, Goran Glavaš, Olga Majewska, Qianchu Liu, Ivan Vulić, Anna Korhonen,

Probing Pretrained Language Models for Lexical Semantics
Ivan Vulić, Edoardo Maria Ponti, Robert Litschko, Goran Glavaš, Anna Korhonen,
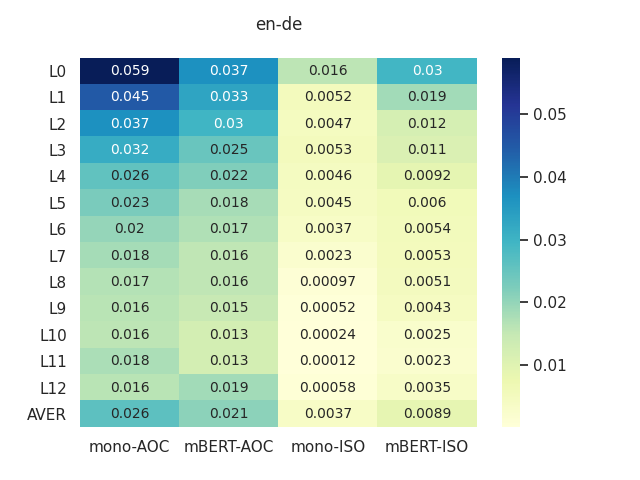