Effectively pretraining a speech translation decoder with Machine Translation data
Ashkan Alinejad, Anoop Sarkar
Speech and Multimodality Short Paper
You can open the pre-recorded video in a separate window.
Abstract:
Directly translating from speech to text using an end-to-end approach is still challenging for many language pairs due to insufficient data. Although pretraining the encoder parameters using the Automatic Speech Recognition (ASR) task improves the results in low resource settings, attempting to use pretrained parameters from the Neural Machine Translation (NMT) task has been largely unsuccessful in previous works. In this paper, we will show that by using an adversarial regularizer, we can bring the encoder representations of the ASR and NMT tasks closer even though they are in different modalities, and how this helps us effectively use a pretrained NMT decoder for speech translation.
NOTE: Video may display a random order of authors.
Correct author list is at the top of this page.
Connected Papers in EMNLP2020
Similar Papers
Consistent Transcription and Translation of Speech
Matthias Sperber, Hendra Setiawan, Christian Gollan, Udhay Nallasamy, Matthias Paulik,

Simulated multiple reference training improves low-resource machine translation
Huda Khayrallah, Brian Thompson, Matt Post, Philipp Koehn,

Distilling Multiple Domains for Neural Machine Translation
Anna Currey, Prashant Mathur, Georgiana Dinu,
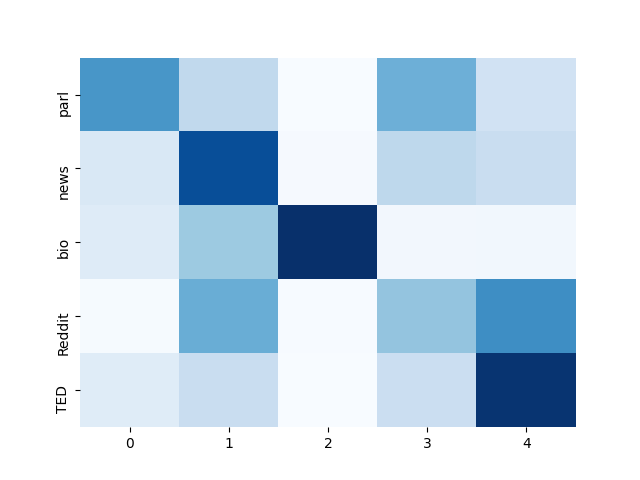