A Spectral Method for Unsupervised Multi-Document Summarization
Kexiang Wang, Baobao Chang, Zhifang Sui
Summarization Long Paper
You can open the pre-recorded video in a separate window.
Abstract:
Multi-document summarization (MDS) aims at producing a good-quality summary for several related documents. In this paper, we propose a spectral-based hypothesis, which states that the goodness of summary candidate is closely linked to its so-called spectral impact. Here spectral impact considers the perturbation to the dominant eigenvalue of affinity matrix when dropping the summary candidate from the document cluster. The hypothesis is validated by three theoretical perspectives: semantic scaling, propagation dynamics and matrix perturbation. According to the hypothesis, we formulate the MDS task as the combinatorial optimization of spectral impact and propose an accelerated greedy solution based on a surrogate of spectral impact. The evaluation results on various datasets demonstrate: (1) The performance of the summary candidate is positively correlated with its spectral impact, which accords with our hypothesis; (2) Our spectral-based method has a competitive result as compared to state-of-the-art MDS systems.
NOTE: Video may display a random order of authors.
Correct author list is at the top of this page.
Connected Papers in EMNLP2020
Similar Papers
Multi-document Summarization with Maximal Marginal Relevance-guided Reinforcement Learning
Yuning Mao, Yanru Qu, Yiqing Xie, Xiang Ren, Jiawei Han,
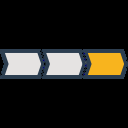
Unsupervised Reference-Free Summary Quality Evaluation via Contrastive Learning
Hanlu Wu, Tengfei Ma, Lingfei Wu, Tariro Manyumwa, Shouling Ji,

Q-learning with Language Model for Edit-based Unsupervised Summarization
Ryosuke Kohita, Akifumi Wachi, Yang Zhao, Ryuki Tachibana,
