Multi-hop Inference for Question-driven Summarization
Yang Deng, Wenxuan Zhang, Wai Lam
Summarization Long Paper
Abstract:
Question-driven summarization has been recently studied as an effective approach to summarizing the source document to produce concise but informative answers for non-factoid questions. In this work, we propose a novel question-driven abstractive summarization method, Multi-hop Selective Generator (MSG), to incorporate multi-hop reasoning into question-driven summarization and, meanwhile, provide justifications for the generated summaries. Specifically, we jointly model the relevance to the question and the interrelation among different sentences via a human-like multi-hop inference module, which captures important sentences for justifying the summarized answer. A gated selective pointer generator network with a multi-view coverage mechanism is designed to integrate diverse information from different perspectives. Experimental results show that the proposed method consistently outperforms state-of-the-art methods on two non-factoid QA datasets, namely WikiHow and PubMedQA.
Connected Papers in EMNLP2020
Similar Papers
Scalable Multi-Hop Relational Reasoning for Knowledge-Aware Question Answering
Yanlin Feng, Xinyue Chen, Bill Yuchen Lin, Peifeng Wang, Jun Yan, Xiang Ren,
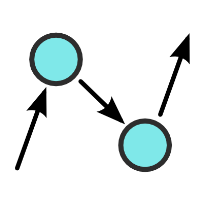
Hierarchical Graph Network for Multi-hop Question Answering
Yuwei Fang, Siqi Sun, Zhe Gan, Rohit Pillai, Shuohang Wang, Jingjing Liu,
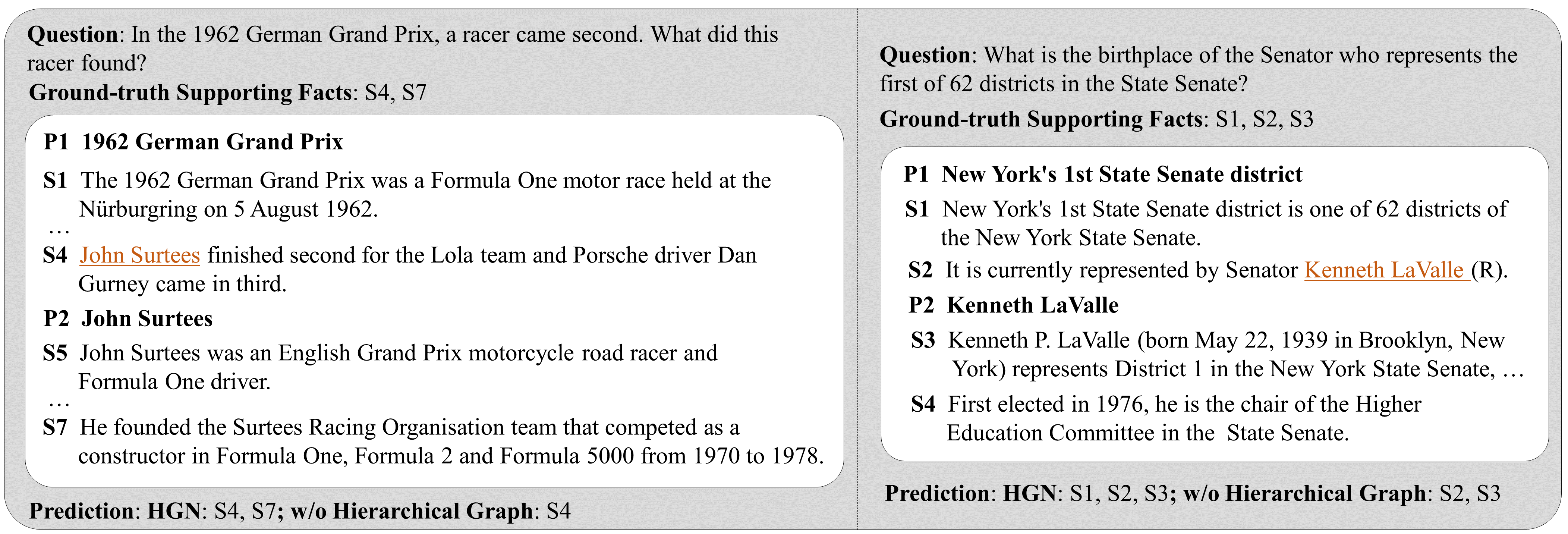
PathQG: Neural Question Generation from Facts
Siyuan Wang, Zhongyu Wei, Zhihao Fan, Zengfeng Huang, Weijian Sun, Qi Zhang, Xuanjing Huang,
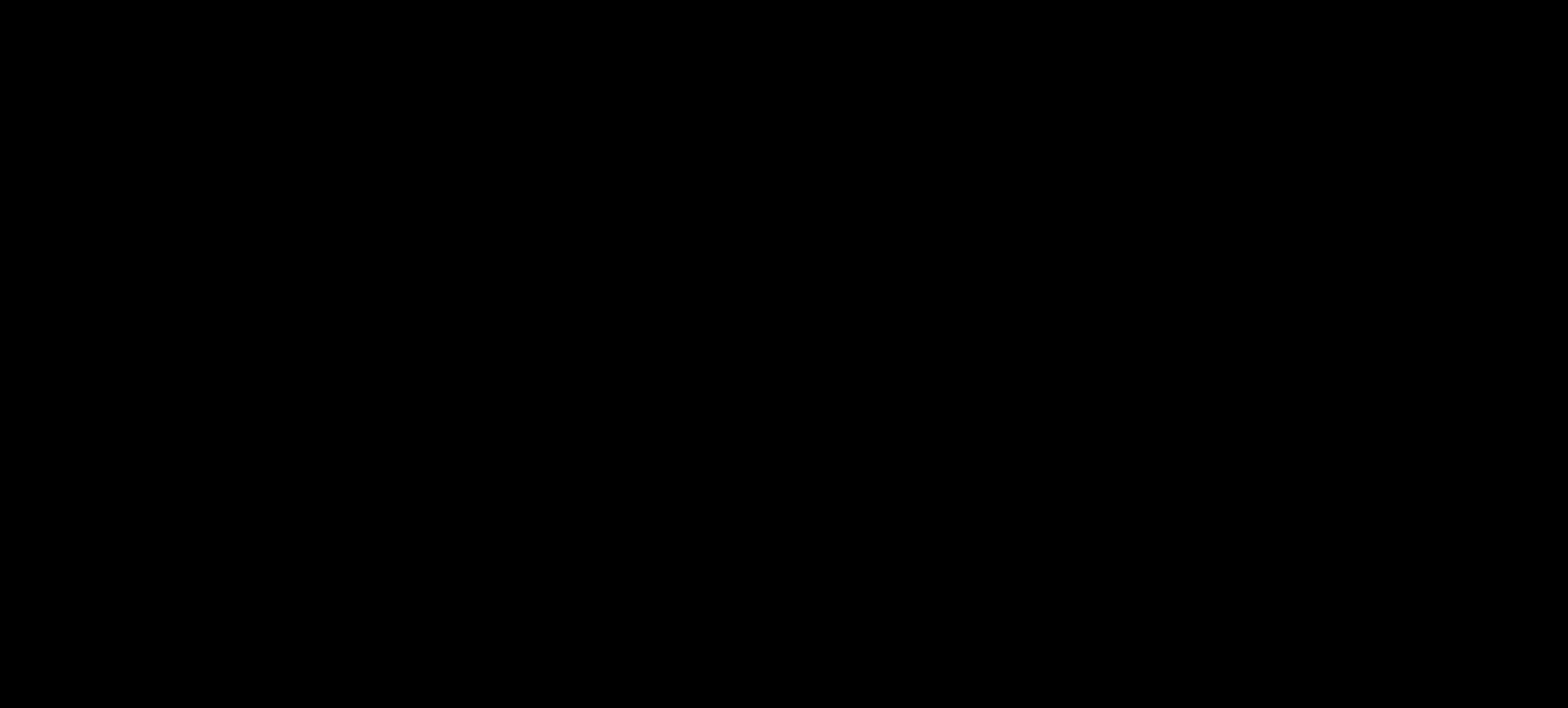