Tired of Topic Models? Clusters of Pretrained Word Embeddings Make for Fast and Good Topics too!
Suzanna Sia, Ayush Dalmia, Sabrina J. Mielke
Information Retrieval and Text Mining Short Paper
You can open the pre-recorded video in a separate window.
Abstract:
Topic models are a useful analysis tool to uncover the underlying themes within document collections. The dominant approach is to use probabilistic topic models that posit a generative story, but in this paper we propose an alternative way to obtain topics: clustering pre-trained word embeddings while incorporating document information for weighted clustering and reranking top words. We provide benchmarks for the combination of different word embeddings and clustering algorithms, and analyse their performance under dimensionality reduction with PCA. The best performing combination for our approach performs as well as classical topic models, but with lower runtime and computational complexity.
NOTE: Video may display a random order of authors.
Correct author list is at the top of this page.
Connected Papers in EMNLP2020
Similar Papers
Improving Neural Topic Models using Knowledge Distillation
Alexander Miserlis Hoyle, Pranav Goel, Philip Resnik,

Compositional Demographic Word Embeddings
Charles Welch, Jonathan K. Kummerfeld, Verónica Pérez-Rosas, Rada Mihalcea,

Short Text Topic Modeling with Topic Distribution Quantization and Negative Sampling Decoder
Xiaobao Wu, Chunping Li, Yan Zhu, Yishu Miao,

Methods for Numeracy-Preserving Word Embeddings
Dhanasekar Sundararaman, Shijing Si, Vivek Subramanian, Guoyin Wang, Devamanyu Hazarika, Lawrence Carin,
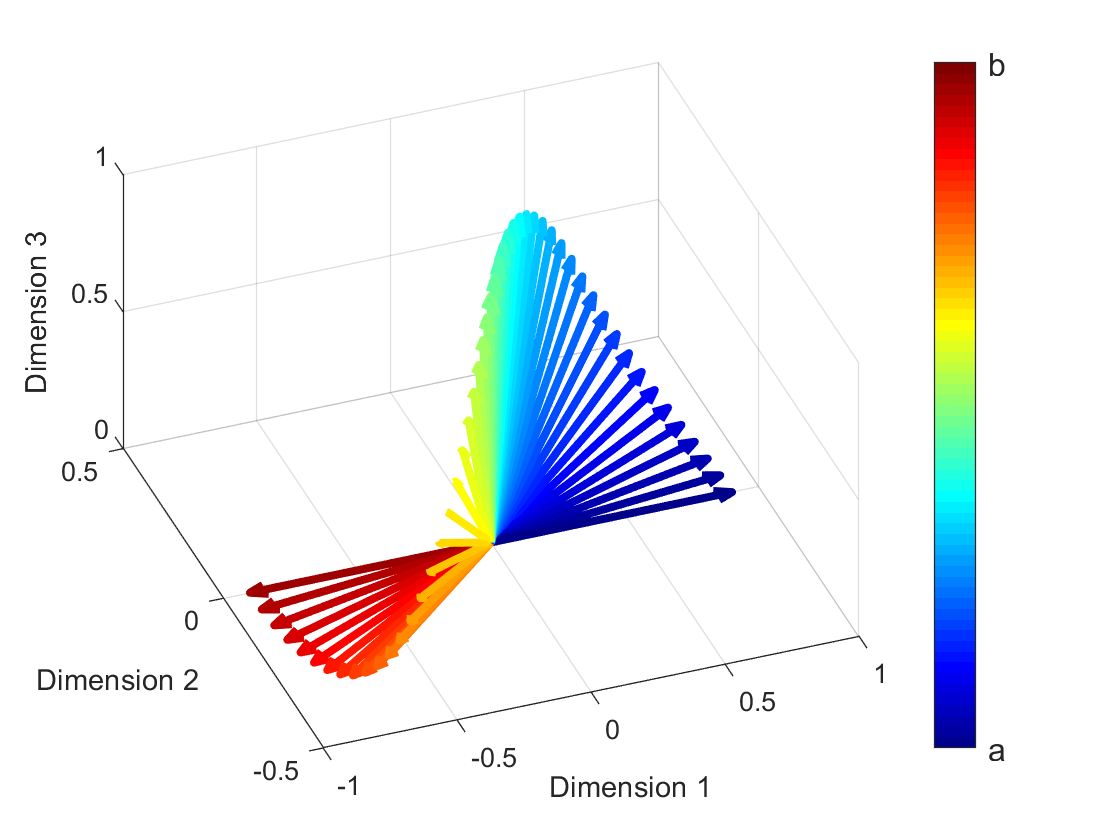