Compositional Demographic Word Embeddings
Charles Welch, Jonathan K. Kummerfeld, Verónica Pérez-Rosas, Rada Mihalcea
Semantics: Lexical Semantics Long Paper
You can open the pre-recorded video in a separate window.
Abstract:
Word embeddings are usually derived from corpora containing text from many individuals, thus leading to general purpose representations rather than individually personalized representations. While personalized embeddings can be useful to improve language model performance and other language processing tasks, they can only be computed for people with a large amount of longitudinal data, which is not the case for new users. We propose a new form of personalized word embeddings that use demographic-specific word representations derived compositionally from full or partial demographic information for a user (i.e., gender, age, location, religion). We show that the resulting demographic-aware word representations outperform generic word representations on two tasks for English: language modeling and word associations. We further explore the trade-off between the number of available attributes and their relative effectiveness and discuss the ethical implications of using them.
NOTE: Video may display a random order of authors.
Correct author list is at the top of this page.
Connected Papers in EMNLP2020
Similar Papers
Tired of Topic Models? Clusters of Pretrained Word Embeddings Make for Fast and Good Topics too!
Suzanna Sia, Ayush Dalmia, Sabrina J. Mielke,
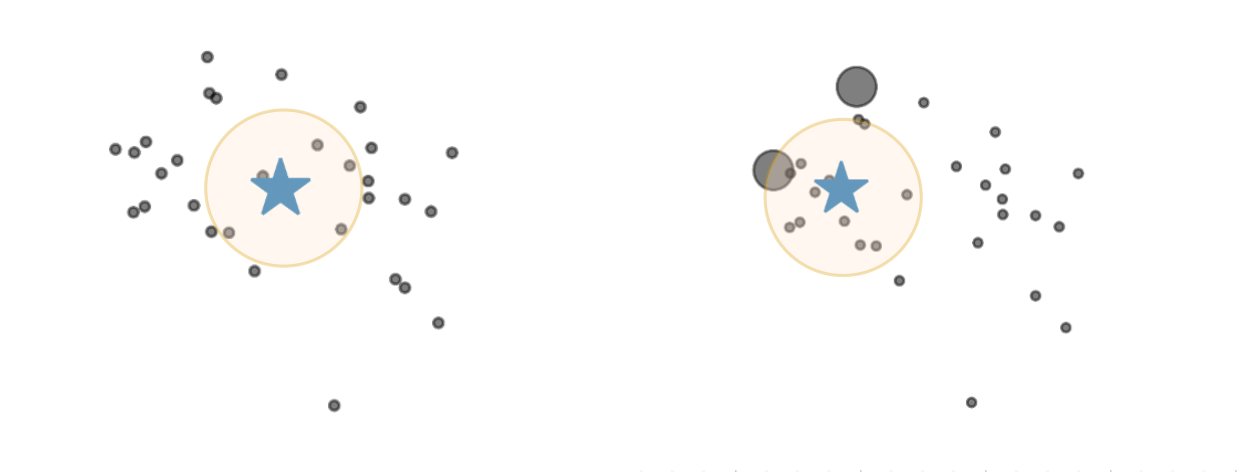
Interactive Refinement of Cross-Lingual Word Embeddings
Michelle Yuan, Mozhi Zhang, Benjamin Van Durme, Leah Findlater, Jordan Boyd-Graber,
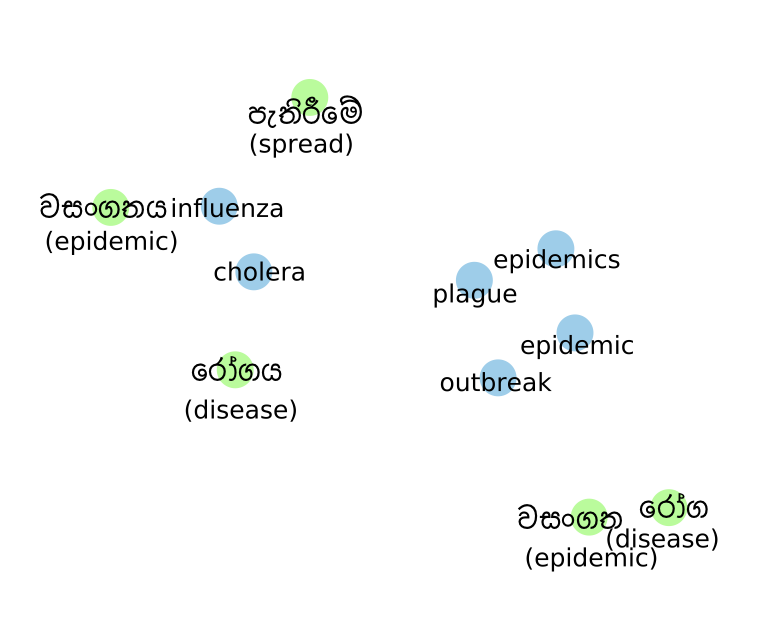