Improving Neural Topic Models using Knowledge Distillation
Alexander Miserlis Hoyle, Pranav Goel, Philip Resnik
Information Retrieval and Text Mining Long Paper
You can open the pre-recorded video in a separate window.
Abstract:
Topic models are often used to identify human-interpretable topics to help make sense of large document collections. We use knowledge distillation to combine the best attributes of probabilistic topic models and pretrained transformers. Our modular method can be straightforwardly applied with any neural topic model to improve topic quality, which we demonstrate using two models having disparate architectures, obtaining state-of-the-art topic coherence. We show that our adaptable framework not only improves performance in the aggregate over all estimated topics, as is commonly reported, but also in head-to-head comparisons of aligned topics.
NOTE: Video may display a random order of authors.
Correct author list is at the top of this page.
Connected Papers in EMNLP2020
Similar Papers
Modeling Content Importance for Summarization with Pre-trained Language Models
Liqiang Xiao, Lu Wang, Hao He, Yaohui Jin,
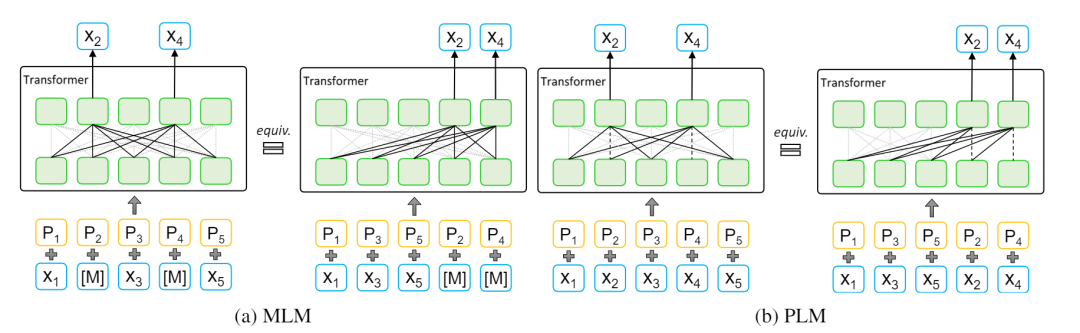
DualTKB: A Dual Learning Bridge between Text and Knowledge Base
Pierre Dognin, Igor Melnyk, Inkit Padhi, Cicero Nogueira dos Santos, Payel Das,
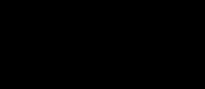
Summarizing Text on Any Aspects: A Knowledge-Informed Weakly-Supervised Approach
Bowen Tan, Lianhui Qin, Eric Xing, Zhiting Hu,
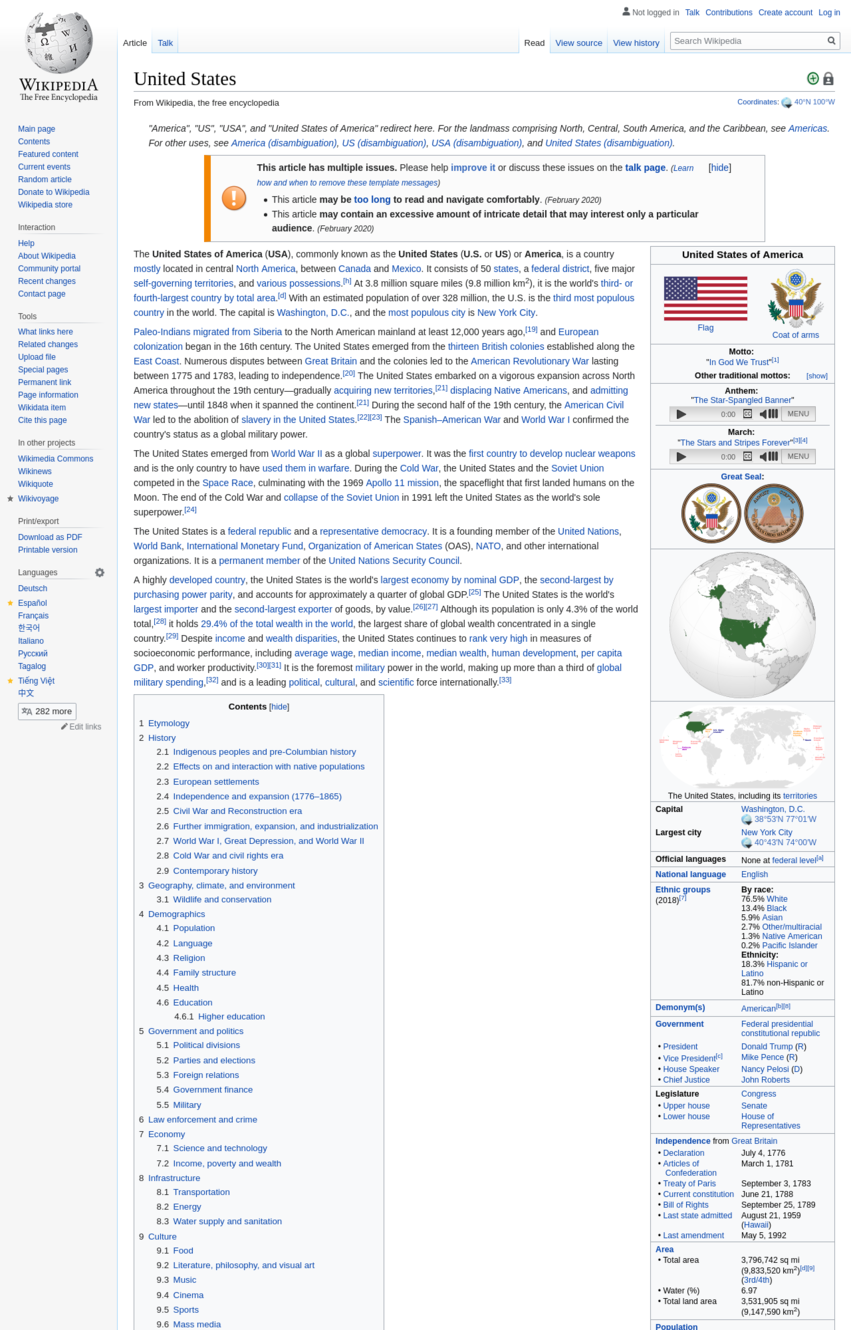