Semantic Label Smoothing for Sequence to Sequence Problems
Michal Lukasik, Himanshu Jain, Aditya Menon, Seungyeon Kim, Srinadh Bhojanapalli, Felix Yu, Sanjiv Kumar
Machine Learning for NLP Short Paper
You can open the pre-recorded video in a separate window.
Abstract:
Label smoothing has been shown to be an effective regularization strategy in classification, that prevents overfitting and helps in label de-noising. However, extending such methods directly to seq2seq settings, such as Machine Translation, is challenging: the large target output space of such problems makes it intractable to apply label smoothing over all possible outputs. Most existing approaches for seq2seq settings either do token level smoothing, or smooth over sequences generated by randomly substituting tokens in the target sequence. Unlike these works, in this paper, we propose a technique that smooths over \emph{well formed} relevant sequences that not only have sufficient n-gram overlap with the target sequence, but are also \emph{semantically similar}. Our method shows a consistent and significant improvement over the state-of-the-art techniques on different datasets.
NOTE: Video may display a random order of authors.
Correct author list is at the top of this page.
Connected Papers in EMNLP2020
Similar Papers
Uncertainty-Aware Semantic Augmentation for Neural Machine Translation
Xiangpeng Wei, Heng Yu, Yue Hu, Rongxiang Weng, Luxi Xing, Weihua Luo,
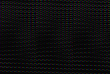
An Unsupervised Sentence Embedding Method by Mutual Information Maximization
Yan Zhang, Ruidan He, Zuozhu Liu, Kwan Hui Lim, Lidong Bing,
