Neural Topic Modeling by Incorporating Document Relationship Graph
Deyu Zhou, Xuemeng Hu, Rui Wang
NLP Applications Short Paper
You can open the pre-recorded video in a separate window.
Abstract:
Graph Neural Networks (GNNs) that capture the relationships between graph nodes via message passing have been a hot research direction in the natural language processing community. In this paper, we propose Graph Topic Model (GTM), a GNN based neural topic model that represents a corpus as a document relationship graph. Documents and words in the corpus become nodes in the graph and are connected based on document-word co-occurrences. By introducing the graph structure, the relationships between documents are established through their shared words and thus the topical representation of a document is enriched by aggregating information from its neighboring nodes using graph convolution. Extensive experiments on three datasets were conducted and the results demonstrate the effectiveness of the proposed approach.
NOTE: Video may display a random order of authors.
Correct author list is at the top of this page.
Connected Papers in EMNLP2020
Similar Papers
Modeling Global and Local Node Contexts for Text Generation from Knowledge Graphs
Leonardo F. R. Ribeiro, Yue Zhang, Claire Gardent, Iryna Gurevych,

Hierarchical Graph Network for Multi-hop Question Answering
Yuwei Fang, Siqi Sun, Zhe Gan, Rohit Pillai, Shuohang Wang, Jingjing Liu,
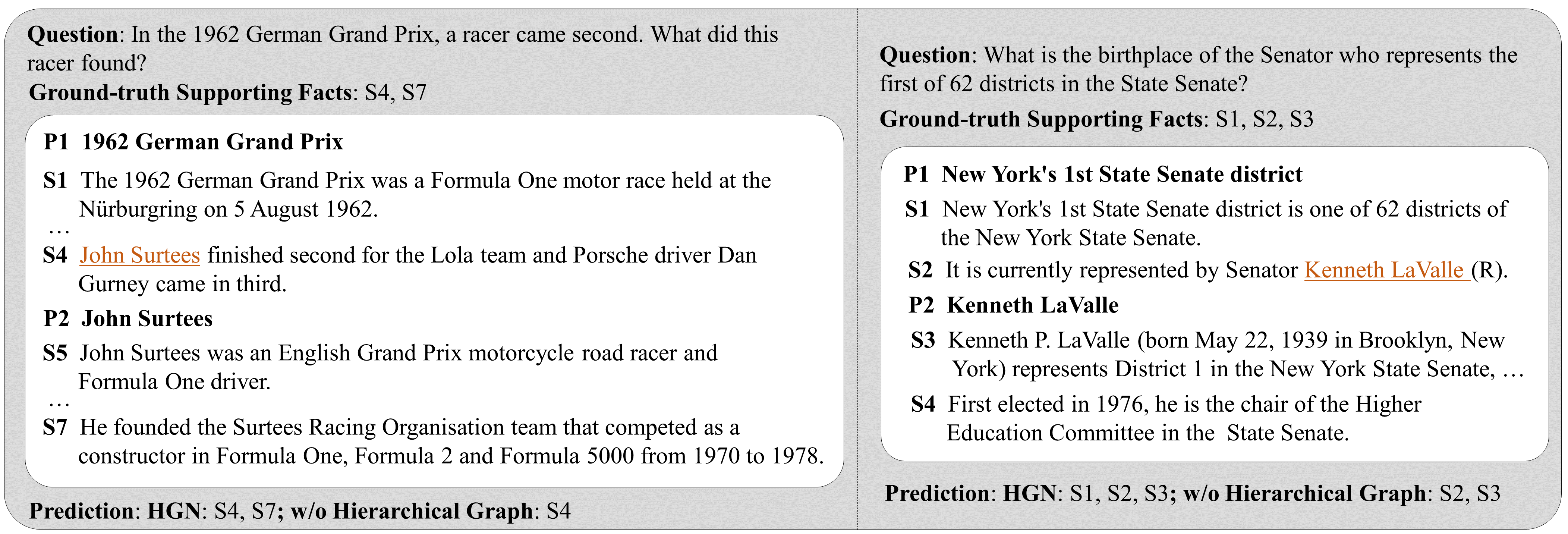
Be More with Less: Hypergraph Attention Networks for Inductive Text Classification
Kaize Ding, Jianling Wang, Jundong Li, Dingcheng Li, Huan Liu,
