Modeling Global and Local Node Contexts for Text Generation from Knowledge Graphs
Leonardo F. R. Ribeiro, Yue Zhang, Claire Gardent, Iryna Gurevych
Language Generation Tacl Paper
You can open the pre-recorded video in a separate window.
Abstract:
Recent graph-to-text models generate text from graph-based data using either global or local aggregation to learn node representations. Global node encoding allows explicit communication between two distant nodes, thereby neglecting graph topology as all nodes are directly connected. In contrast, local node encoding considers the relations between neighbor nodes capturing the graph structure, but it can fail to capture long-range relations. In this work, we gather both encoding strategies, proposing novel neural models which encode an input graph combining both global and local node contexts, in order to learn better contextualized node embeddings. In our experiments, we demonstrate that our approaches lead to significant improvements on two graph-to-text datasets achieving BLEU scores of 18.01 on AGENDA dataset, and 63.69 on the WebNLG dataset for seen categories, outperforming state-of-the-art models by 3.7 and 3.1 points, respectively.
NOTE: Video may display a random order of authors.
Correct author list is at the top of this page.
Connected Papers in EMNLP2020
Similar Papers
Is Graph Structure Necessary for Multi-hop Question Answering?
Nan Shao, Yiming Cui, Ting Liu, Shijin Wang, Guoping Hu,
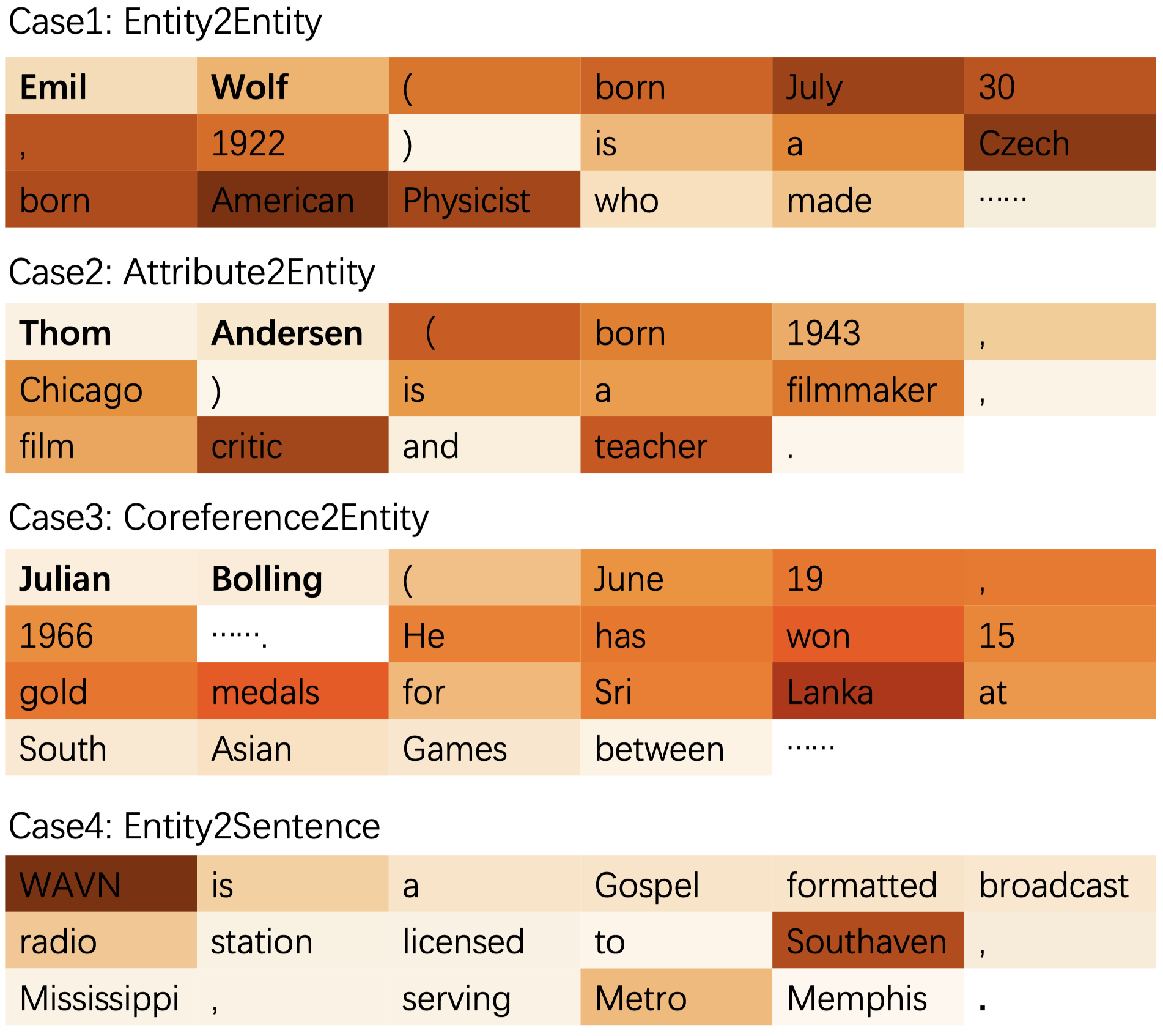
Exploiting Structured Knowledge in Text via Graph-Guided Representation Learning
Tao Shen, Yi Mao, Pengcheng He, Guodong Long, Adam Trischler, Weizhu Chen,

An Unsupervised Joint System for Text Generation from Knowledge Graphs and Semantic Parsing
Martin Schmitt, Sahand Sharifzadeh, Volker Tresp, Hinrich Schütze,
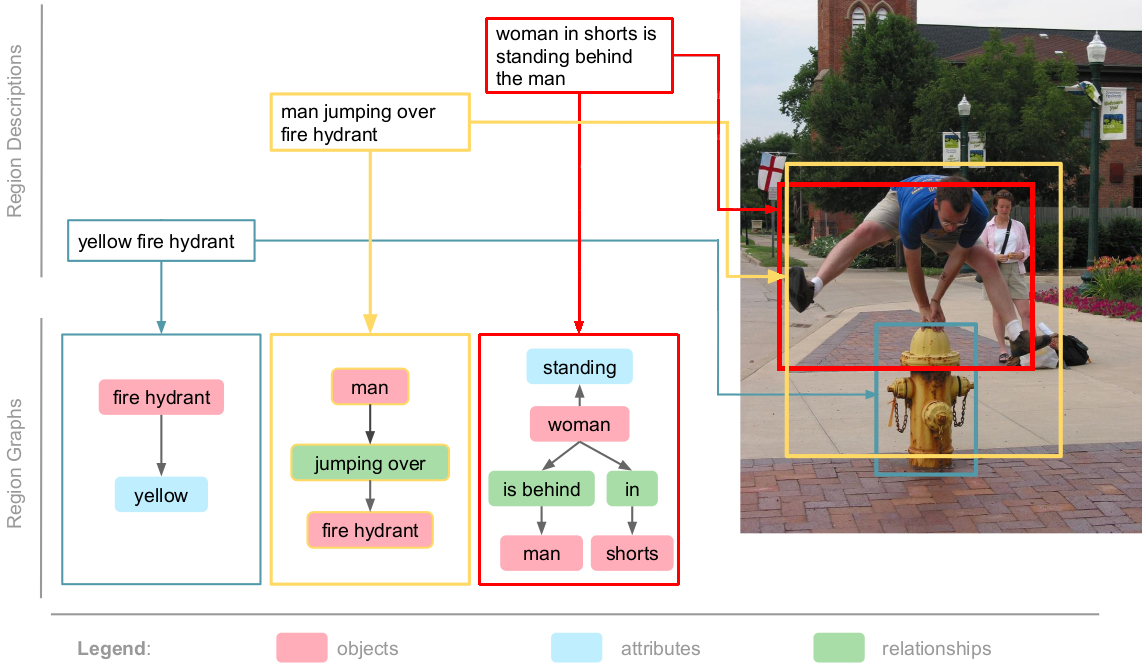
A Knowledge-Aware Sequence-to-Tree Network for Math Word Problem Solving
Qinzhuo Wu, Qi Zhang, Jinlan Fu, Xuanjing Huang,
