Text Graph Transformer for Document Classification
Haopeng Zhang, Jiawei Zhang
Information Retrieval and Text Mining Short Paper
You can open the pre-recorded video in a separate window.
Abstract:
Text classification is a fundamental problem in natural language processing. Recent studies applied graph neural network (GNN) techniques to capture global word co-occurrence in a corpus. However, previous works are not scalable to large-sized corpus and ignore the heterogeneity of the text graph. To address these problems, we introduce a novel Transformer based heterogeneous graph neural network, namely Text Graph Transformer (TG-Transformer). Our model learns effective node representations by capturing structure and heterogeneity from the text graph. We propose a mini-batch text graph sampling method that significantly reduces computing and memory costs to handle large-sized corpus. Extensive experiments have been conducted on several benchmark datasets, and the results demonstrate that TG-Transformer outperforms state-of-the-art approaches on text classification task.
NOTE: Video may display a random order of authors.
Correct author list is at the top of this page.
Connected Papers in EMNLP2020
Similar Papers
Is Graph Structure Necessary for Multi-hop Question Answering?
Nan Shao, Yiming Cui, Ting Liu, Shijin Wang, Guoping Hu,
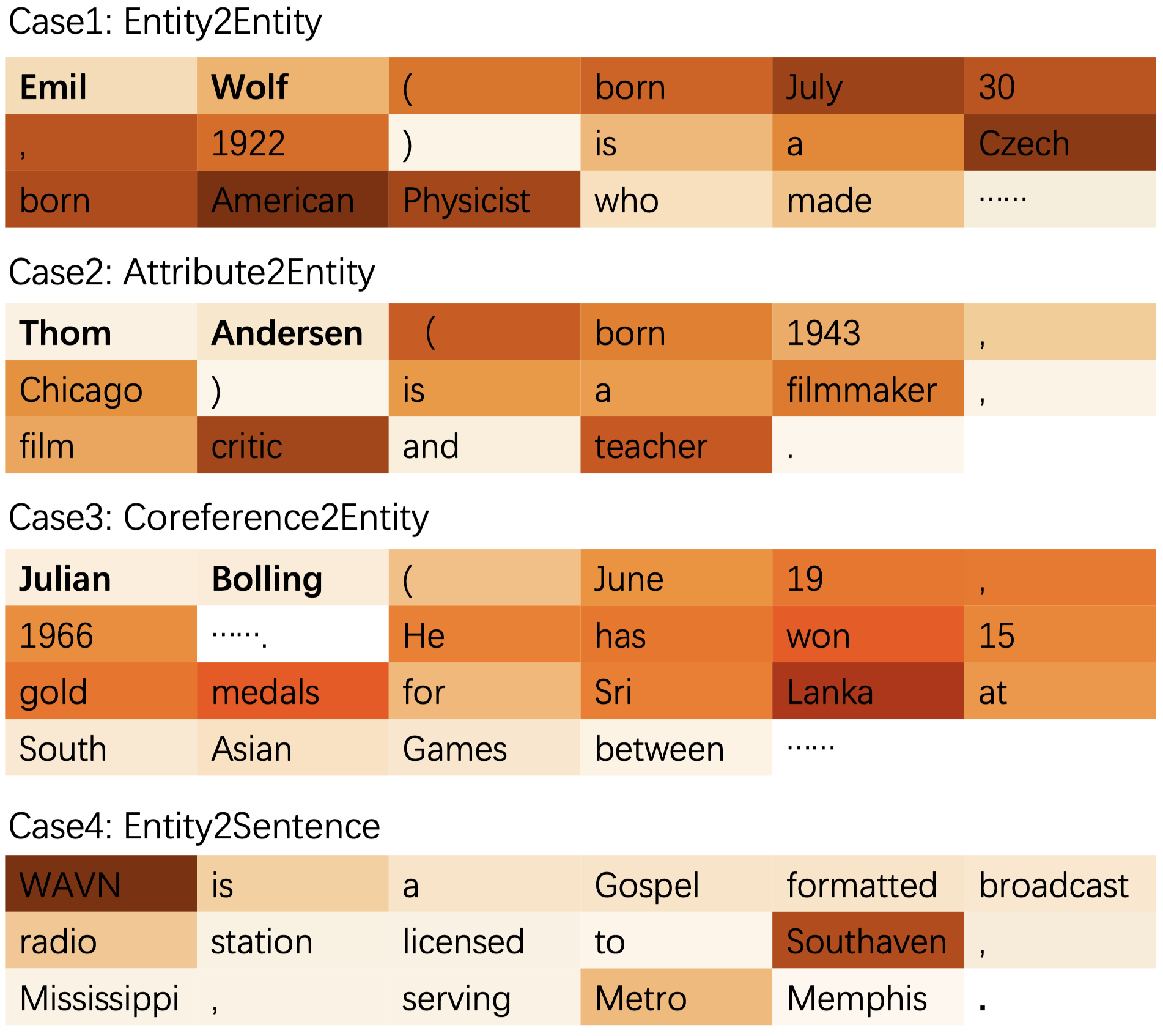
Modeling Global and Local Node Contexts for Text Generation from Knowledge Graphs
Leonardo F. R. Ribeiro, Yue Zhang, Claire Gardent, Iryna Gurevych,

Neural Extractive Summarization with Hierarchical Attentive Heterogeneous Graph Network
Ruipeng Jia, Yanan Cao, Hengzhu Tang, Fang Fang, Cong Cao, Shi Wang,
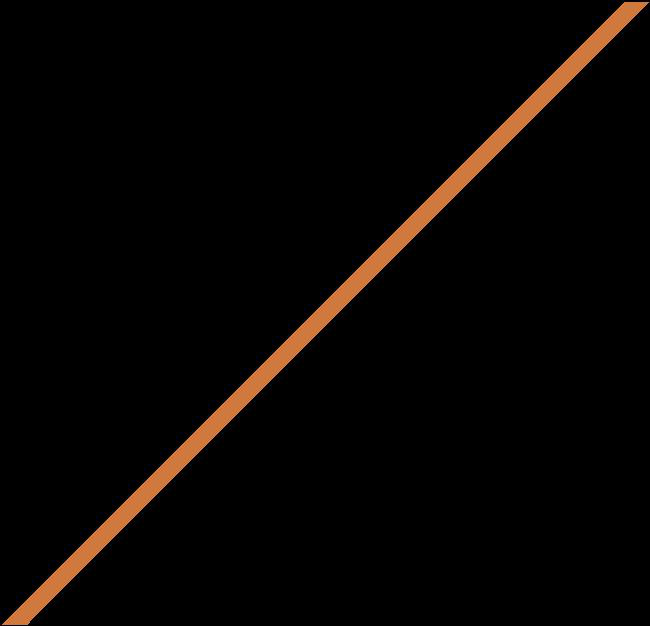