Data Rejuvenation: Exploiting Inactive Training Examples for Neural Machine Translation
Wenxiang Jiao, Xing Wang, Shilin He, Irwin King, Michael Lyu, Zhaopeng Tu
Machine Translation and Multilinguality Long Paper
You can open the pre-recorded video in a separate window.
Abstract:
Large-scale training datasets lie at the core of the recent success of neural machine translation (NMT) models. However, the complex patterns and potential noises in the large-scale data make training NMT models difficult. In this work, we explore to identify the inactive training examples which contribute less to the model performance, and show that the existence of inactive examples depends on the data distribution. We further introduce data rejuvenation to improve the training of NMT models on large-scale datasets by exploiting inactive examples. The proposed framework consists of three phases. First, we train an identification model on the original training data, and use it to distinguish inactive examples and active examples by their sentence-level output probabilities. Then, we train a rejuvenation model on the active examples, which is used to re-label the inactive examples with forward- translation. Finally, the rejuvenated examples and the active examples are combined to train the final NMT model. Experimental results on WMT14 English-German and English-French datasets show that the proposed data rejuvenation consistently and significantly improves performance for several strong NMT models. Extensive analyses reveal that our approach stabilizes and accelerates the training process of NMT models, resulting in final models with better generalization capability.
NOTE: Video may display a random order of authors.
Correct author list is at the top of this page.
Connected Papers in EMNLP2020
Similar Papers
Data Weighted Training Strategies for Grammatical Error Correction
Jared Lichtarge, Chris Alberti, Shankar Kumar,

Can Automatic Post-Editing Improve NMT?
Shamil Chollampatt, Raymond Hendy Susanto, Liling Tan, Ewa Szymanska,
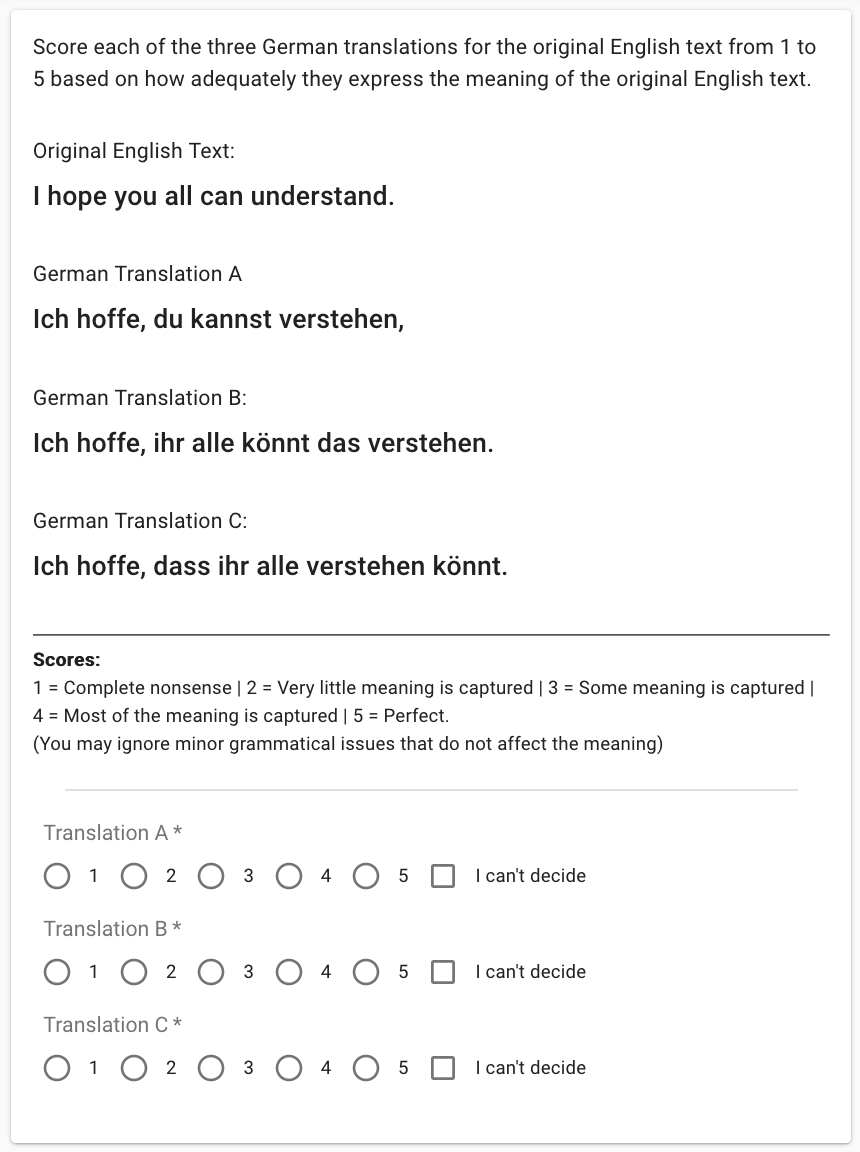
Language Model Prior for Low-Resource Neural Machine Translation
Christos Baziotis, Barry Haddow, Alexandra Birch,
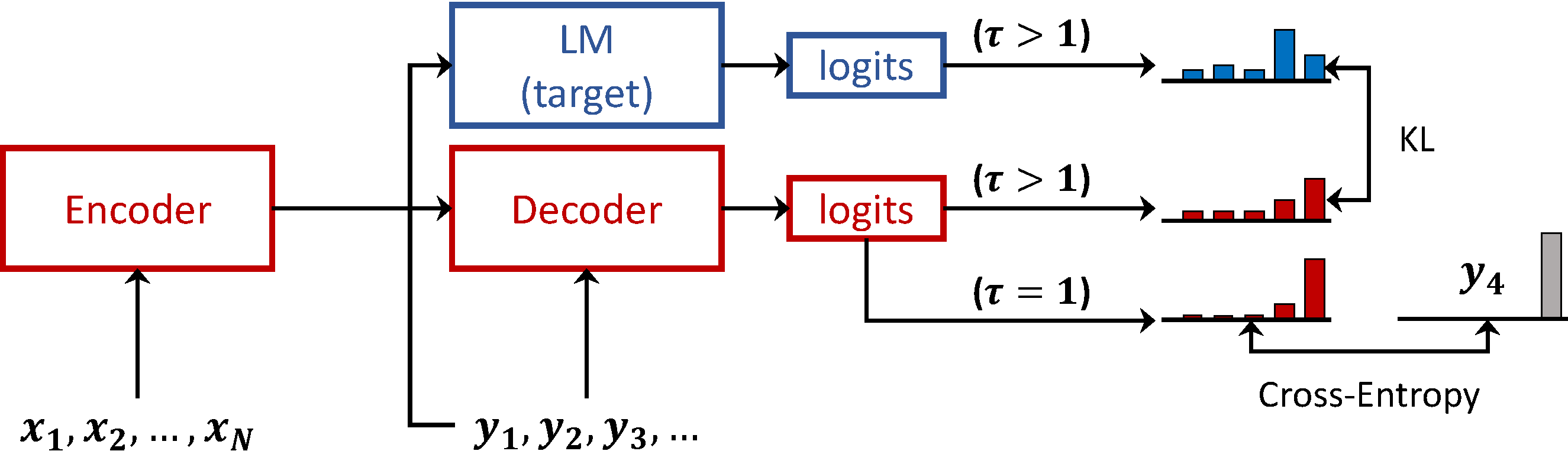
On the Sparsity of Neural Machine Translation Models
Yong Wang, Longyue Wang, Victor Li, Zhaopeng Tu,
