PARADE: A New Dataset for Paraphrase Identification Requiring Computer Science Domain Knowledge
Yun He, Zhuoer Wang, Yin Zhang, Ruihong Huang, James Caverlee
Semantics: Sentence-level Semantics, Textual Inference and Other areas Long Paper
You can open the pre-recorded video in a separate window.
Abstract:
We present a new benchmark dataset called PARADE for paraphrase identification that requires specialized domain knowledge. PARADE contains paraphrases that overlap very little at the lexical and syntactic level but are semantically equivalent based on computer science domain knowledge, as well as non-paraphrases that overlap greatly at the lexical and syntactic level but are not semantically equivalent based on this domain knowledge. Experiments show that both state-of-the-art neural models and non-expert human annotators have poor performance on PARADE. For example, BERT after fine-tuning achieves an F1 score of 0.709, which is much lower than its performance on other paraphrase identification datasets. PARADE can serve as a resource for researchers interested in testing models that incorporate domain knowledge. We make our data and code freely available.
NOTE: Video may display a random order of authors.
Correct author list is at the top of this page.
Connected Papers in EMNLP2020
Similar Papers
Small but Mighty: New Benchmarks for Split and Rephrase
Li Zhang, Huaiyu Zhu, Siddhartha Brahma, Yunyao Li,
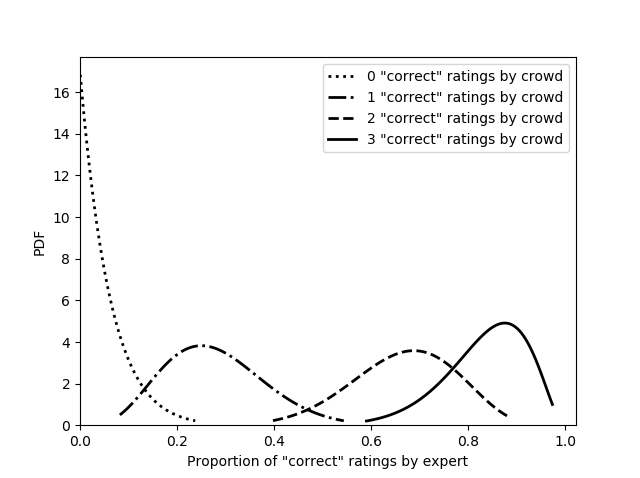
Compositional and Lexical Semantics in RoBERTa, BERT and DistilBERT: A Case Study on CoQA
Ieva Staliūnaitė, Ignacio Iacobacci,

Learning from Context or Names? An Empirical Study on Neural Relation Extraction
Hao Peng, Tianyu Gao, Xu Han, Yankai Lin, Peng Li, Zhiyuan Liu, Maosong Sun, Jie Zhou,
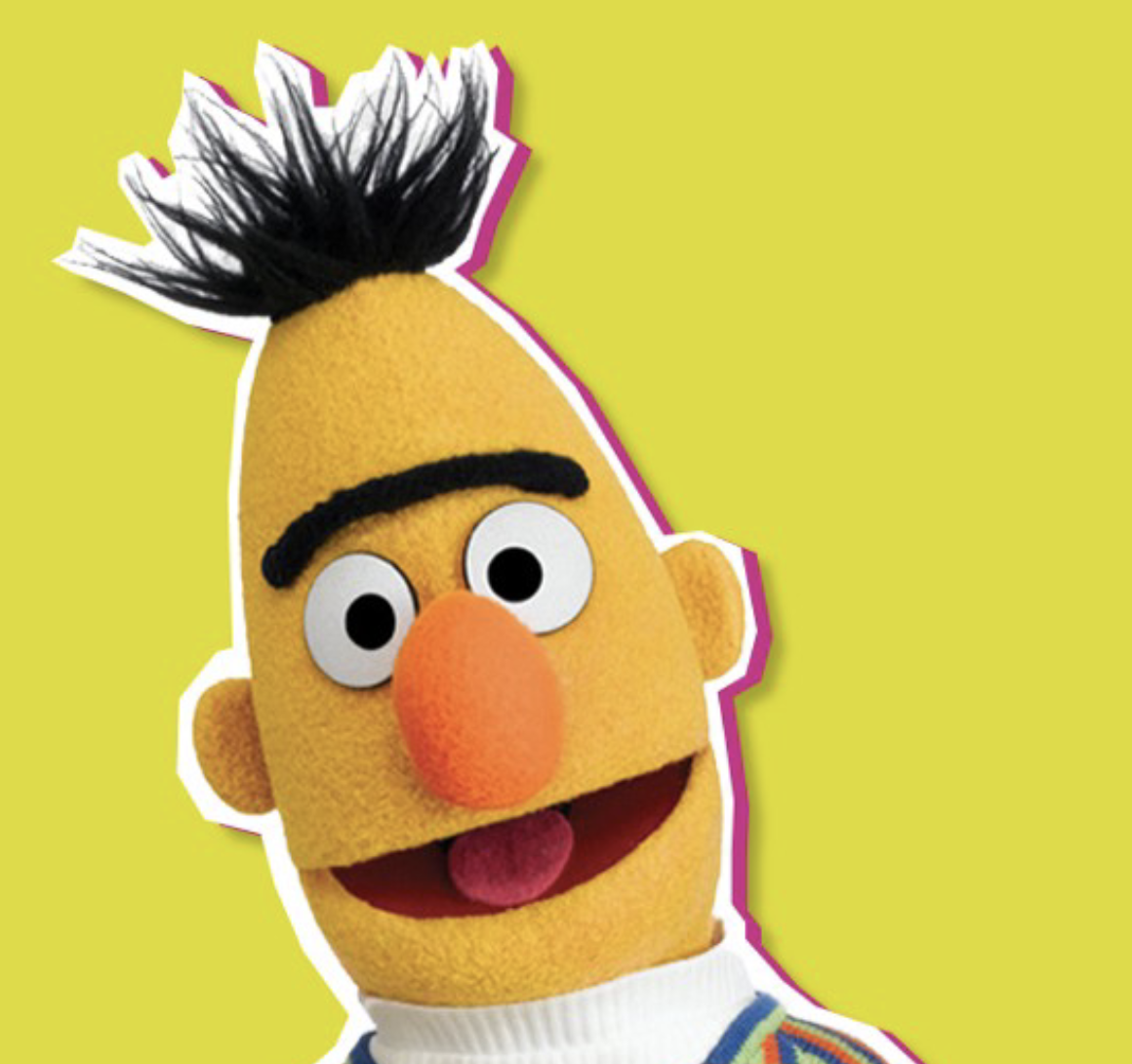