Dissecting Span Identification Tasks with Performance Prediction
Sean Papay, Roman Klinger, Sebastian Padó
Interpretability and Analysis of Models for NLP Long Paper
You can open the pre-recorded video in a separate window.
Abstract:
Span identification (in short, span ID) tasks such as chunking, NER, or code-switching detection, ask models to identify and classify relevant spans in a text. Despite being a staple of NLP, and sharing a common structure, there is little insight on how these tasks' properties influence their difficulty, and thus little guidance on what model families work well on span ID tasks, and why. We analyze span ID tasks via performance prediction, estimating how well neural architectures do on different tasks. Our contributions are: (a) we identify key properties of span ID tasks that can inform performance prediction; (b) we carry out a large-scale experiment on English data, building a model to predict performance for unseen span ID tasks that can support architecture choices; (c), we investigate the parameters of the meta model, yielding new insights on how model and task properties interact to affect span ID performance. We find, e.g., that span frequency is especially important for LSTMs, and that CRFs help when spans are infrequent and boundaries non-distinctive.
NOTE: Video may display a random order of authors.
Correct author list is at the top of this page.
Connected Papers in EMNLP2020
Similar Papers
A Simple and Effective Model for Answering Multi-span Questions
Elad Segal, Avia Efrat, Mor Shoham, Amir Globerson, Jonathan Berant,

oLMpics - On what Language Model Pre-training Captures
Alon Talmor, Yanai Elazar, Yoav Goldberg, Jonathan Berant,

Probing Pretrained Language Models for Lexical Semantics
Ivan Vulić, Edoardo Maria Ponti, Robert Litschko, Goran Glavaš, Anna Korhonen,
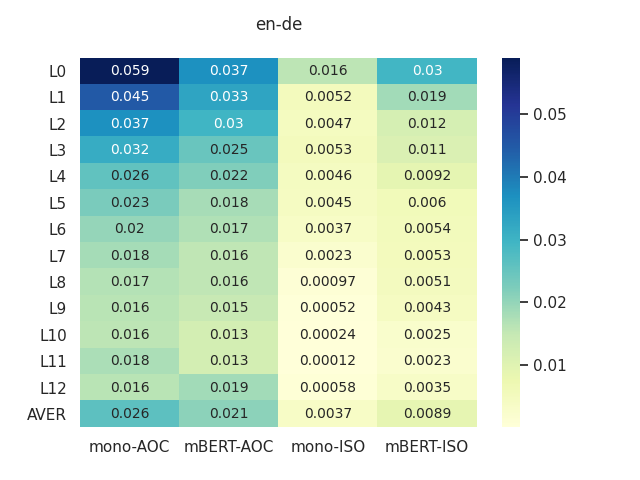