An Empirical Study of Pre-trained Transformers for Arabic Information Extraction
Wuwei Lan, Yang Chen, Wei Xu, Alan Ritter
NLP Applications Short Paper
You can open the pre-recorded video in a separate window.
Abstract:
Multilingual pre-trained Transformers, such as mBERT (Devlin et al., 2019) and XLM-RoBERTa (Conneau et al., 2020a), have been shown to enable effective cross-lingual zero-shot transfer. However, their performance on Arabic information extraction (IE) tasks is not very well studied. In this paper, we pre-train a customized bilingual BERT, dubbed GigaBERT, that is designed specifically for Arabic NLP and English-to-Arabic zero-shot transfer learning. We study GigaBERT's effectiveness on zero-short transfer across four IE tasks: named entity recognition, part-of-speech tagging, argument role labeling, and relation extraction. Our best model significantly outperforms mBERT, XLM-RoBERTa, and AraBERT (Antoun et al., 2020) in both the supervised and zero-shot transfer settings. We have made our pre-trained models publicly available at: https://github.com/lanwuwei/GigaBERT.
NOTE: Video may display a random order of authors.
Correct author list is at the top of this page.
Connected Papers in EMNLP2020
Similar Papers
Multilingual Denoising Pre-training for Neural Machine Translation
Jiatao Gu, Yinhan Liu, Naman Goyal, Xian Li, Sergey Edunov, Marjan Ghazvininejad, Mike Lewis, Luke Zettlemoyer,

MAD-X: An Adapter-Based Framework for Multi-Task Cross-Lingual Transfer
Jonas Pfeiffer, Ivan Vulić, Iryna Gurevych, Sebastian Ruder,

XL-WiC: A Multilingual Benchmark for Evaluating Semantic Contextualization
Alessandro Raganato, Tommaso Pasini, Jose Camacho-Collados, Mohammad Taher Pilehvar,
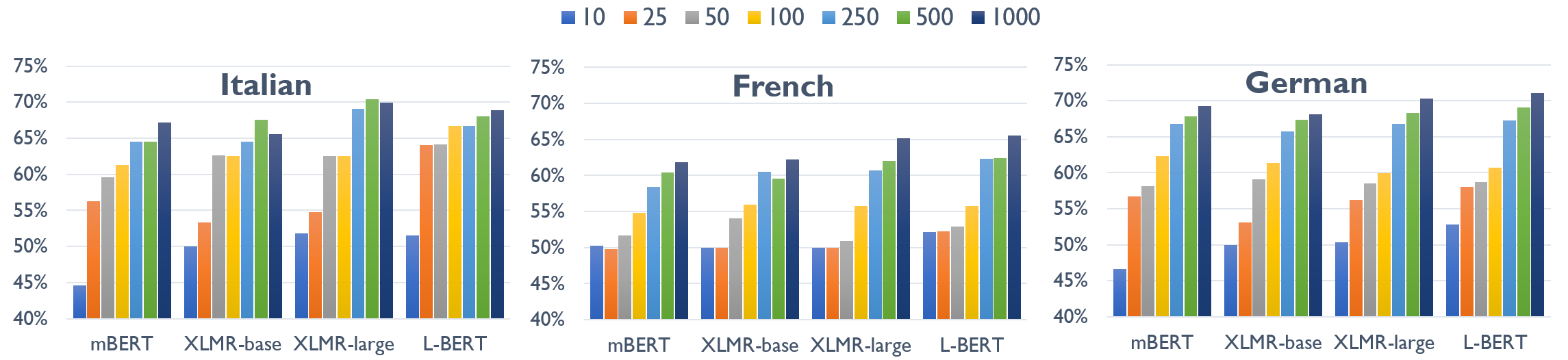
Transfer Learning and Distant Supervision for Multilingual Transformer Models: A Study on African Languages
Michael A. Hedderich, David Adelani, Dawei Zhu, Jesujoba Alabi, Udia Markus, Dietrich Klakow,
