CapWAP: Image Captioning with a Purpose
Adam Fisch, Kenton Lee, Ming-Wei Chang, Jonathan Clark, Regina Barzilay
Language Grounding to Vision, Robotics and Beyond Long Paper
You can open the pre-recorded video in a separate window.
Abstract:
The traditional image captioning task uses generic reference captions to provide textual information about images. Different user populations, however, will care about different visual aspects of images. In this paper, we propose a new task, Captioning with A Purpose (CapWAP). Our goal is to develop systems that can be tailored to be useful for the information needs of an intended population, rather than merely provide generic information about an image. In this task, we use question-answer (QA) pairs---a natural expression of information need---from users, instead of reference captions, for both training and post-inference evaluation. We show that it is possible to use reinforcement learning to directly optimize for the intended information need, by rewarding outputs that allow a question answering model to provide correct answers to sampled user questions. We convert several visual question answering datasets into CapWAP datasets, and demonstrate that under a variety of scenarios our purposeful captioning system learns to anticipate and fulfill specific information needs better than its generic counterparts, as measured by QA performance on user questions from unseen images, when using the caption alone as context.
NOTE: Video may display a random order of authors.
Correct author list is at the top of this page.
Connected Papers in EMNLP2020
Similar Papers
New Protocols and Negative Results for Textual Entailment Data Collection
Samuel R. Bowman, Jennimaria Palomaki, Livio Baldini Soares, Emily Pitler,

Video2Commonsense: Generating Commonsense Descriptions to Enrich Video Captioning
Zhiyuan Fang, Tejas Gokhale, Pratyay Banerjee, Chitta Baral, Yezhou Yang,
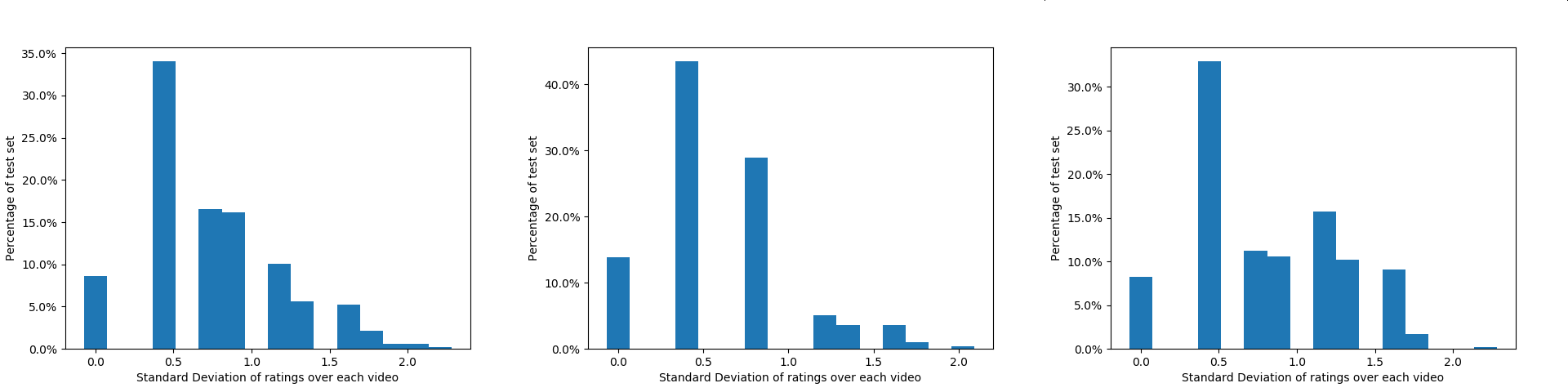
MUTANT: A Training Paradigm for Out-of-Distribution Generalization in Visual Question Answering
Tejas Gokhale, Pratyay Banerjee, Chitta Baral, Yezhou Yang,
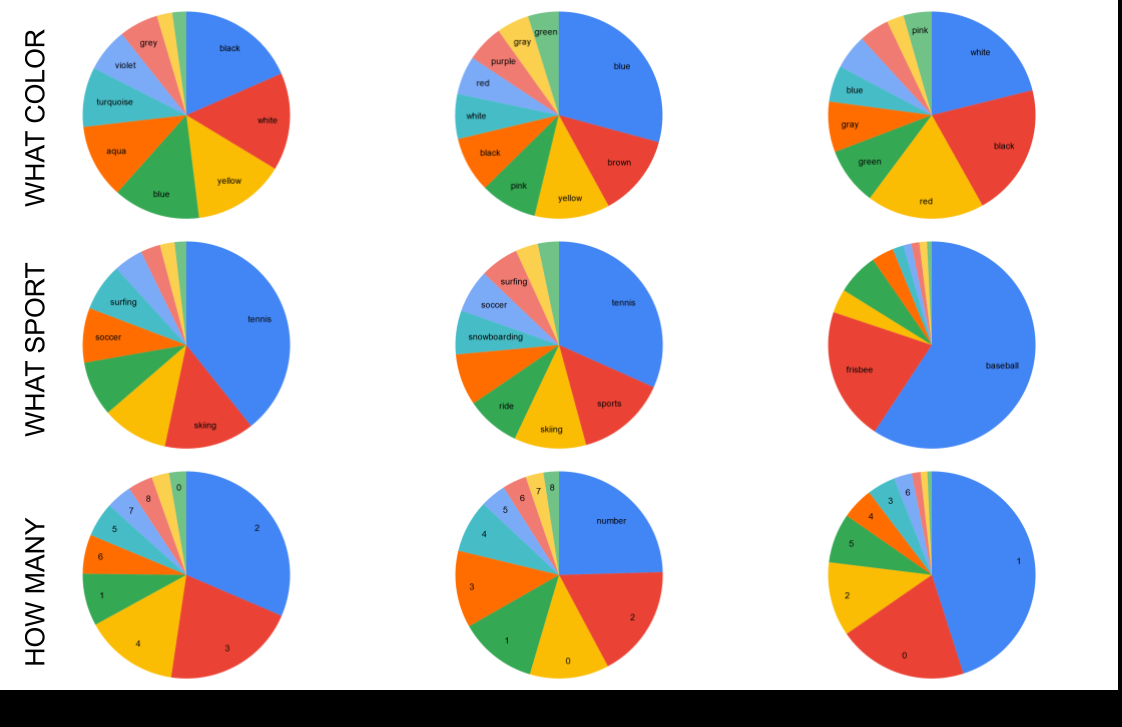
The World is Not Binary: Learning to Rank with Grayscale Data for Dialogue Response Selection
Zibo Lin, Deng Cai, Yan Wang, Xiaojiang Liu, Haitao Zheng, Shuming Shi,
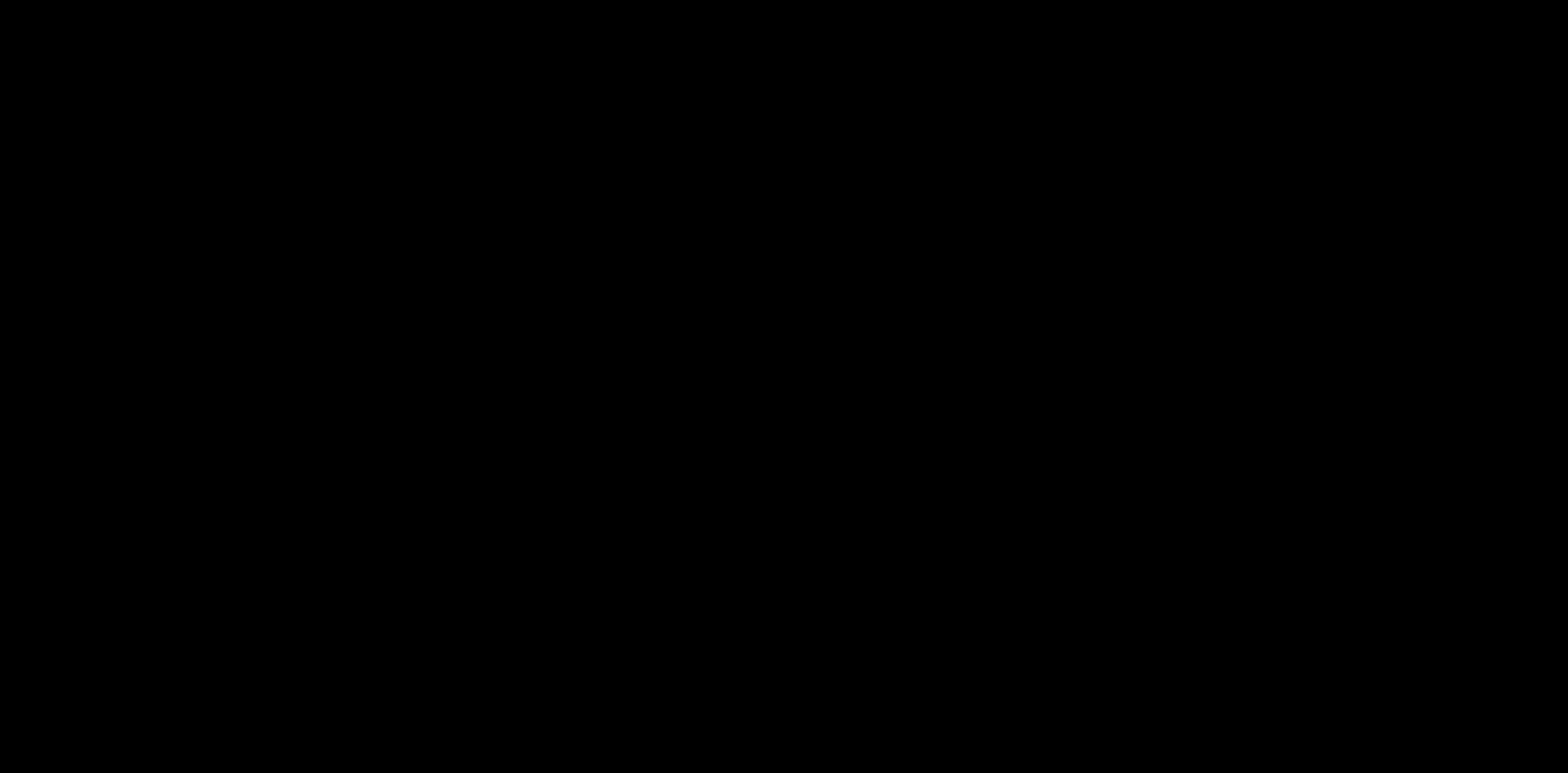