Exploring and Predicting Transferability across NLP Tasks
Tu Vu, Tong Wang, Tsendsuren Munkhdalai, Alessandro Sordoni, Adam Trischler, Andrew Mattarella-Micke, Subhransu Maji, Mohit Iyyer
Machine Learning for NLP Long Paper
You can open the pre-recorded video in a separate window.
Abstract:
Recent advances in NLP demonstrate the effectiveness of training large-scale language models and transferring them to downstream tasks. Can fine-tuning these models on tasks other than language modeling further improve performance? In this paper, we conduct an extensive study of the transferability between 33 NLP tasks across three broad classes of problems (text classification, question answering, and sequence labeling). Our results show that transfer learning is more beneficial than previously thought, especially when target task data is scarce, and can improve performance even with low-data source tasks that differ substantially from the target task (e.g., part-of-speech tagging transfers well to the DROP QA dataset). We also develop task embeddings that can be used to predict the most transferable source tasks for a given target task, and we validate their effectiveness in experiments controlled for source and target data size. Overall, our experiments reveal that factors such as data size, task and domain similarity, and task complexity all play a role in determining transferability.
NOTE: Video may display a random order of authors.
Correct author list is at the top of this page.
Connected Papers in EMNLP2020
Similar Papers
New Protocols and Negative Results for Textual Entailment Data Collection
Samuel R. Bowman, Jennimaria Palomaki, Livio Baldini Soares, Emily Pitler,

From Zero to Hero: On the Limitations of Zero-Shot Language Transfer with Multilingual Transformers
Anne Lauscher, Vinit Ravishankar, Ivan Vulić, Goran Glavaš,
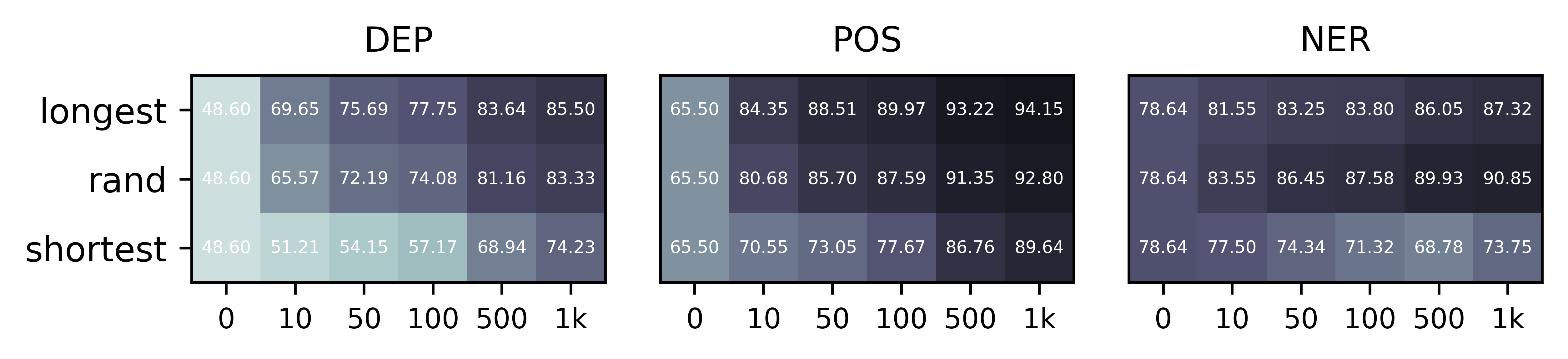
Zero-Shot Cross-Lingual Transfer with Meta Learning
Farhad Nooralahzadeh, Giannis Bekoulis, Johannes Bjerva, Isabelle Augenstein,
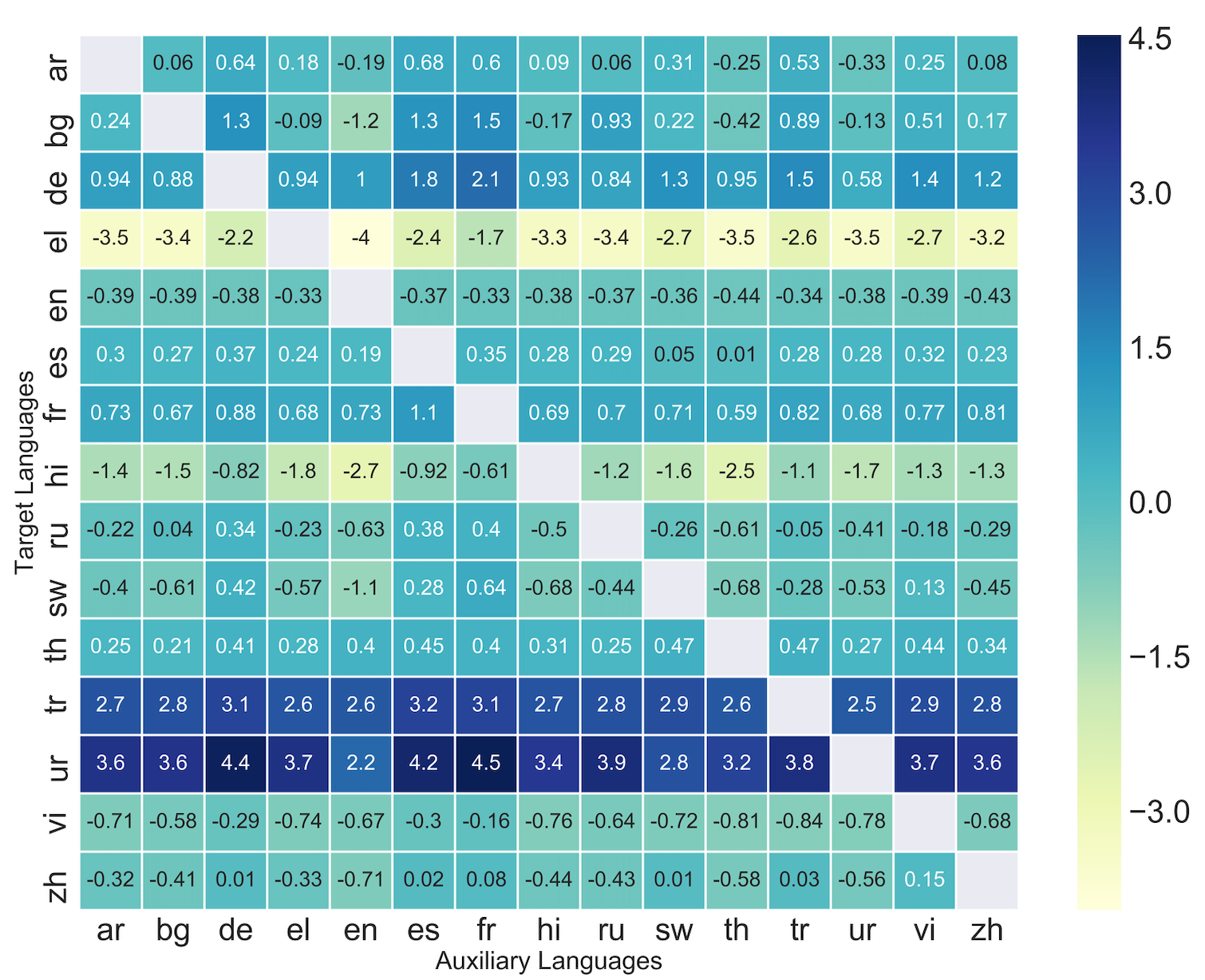