Translation Quality Estimation by Jointly Learning to Score and Rank
Jingyi Zhang, Josef van Genabith
Machine Translation and Multilinguality Short Paper
You can open the pre-recorded video in a separate window.
Abstract:
The translation quality estimation (QE) task, particularly the QE as a Metric task, aims to evaluate the general quality of a translation based on the translation and the source sentence without using reference translations. Supervised learning of this QE task requires human evaluation of translation quality as training data. Human evaluation of translation quality can be performed in different ways, including assigning an absolute score to a translation or ranking different translations. In order to make use of different types of human evaluation data for supervised learning, we present a multi-task learning QE model that jointly learns two tasks: score a translation and rank two translations. Our QE model exploits cross-lingual sentence embeddings from pre-trained multilingual language models. We obtain new state-of-the-art results on the WMT 2019 QE as a Metric task and outperform sentBLEU on the WMT 2019 Metrics task.
NOTE: Video may display a random order of authors.
Correct author list is at the top of this page.
Connected Papers in EMNLP2020
Similar Papers
Unsupervised Quality Estimation for Neural Machine Translation
Marina Fomicheva, Shuo Sun, Lisa Yankovskaya, Frédéric Blain, Francisco Guzmán, Mark Fishel, Nikolaos Aletras, Vishrav Chaudhary, Lucia Specia,

Dynamic Data Selection and Weighting for Iterative Back-Translation
Zi-Yi Dou, Antonios Anastasopoulos, Graham Neubig,
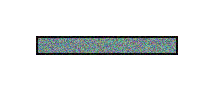
Simulated multiple reference training improves low-resource machine translation
Huda Khayrallah, Brian Thompson, Matt Post, Philipp Koehn,
