IGSQL: Database Schema Interaction Graph Based Neural Model for Context-Dependent Text-to-SQL Generation
Yitao Cai, Xiaojun Wan
Semantics: Sentence-level Semantics, Textual Inference and Other areas Long Paper
You can open the pre-recorded video in a separate window.
Abstract:
Context-dependent text-to-SQL task has drawn much attention in recent years. Previous models on context-dependent text-to-SQL task only concentrate on utilizing historic user inputs. In this work, in addition to using encoders to capture historic information of user inputs, we propose a database schema interaction graph encoder to utilize historic information of database schema items. In decoding phase, we introduce a gate mechanism to weigh the importance of different vocabularies and then make the prediction of SQL tokens. We evaluate our model on the benchmark SParC and CoSQL datasets, which are two large complex context-dependent cross-domain text-to-SQL datasets. Our model outperforms previous state-of-the-art model by a large margin and achieves new state-of-the-art results on the two datasets. The comparison and ablation results demonstrate the efficacy of our model and the usefulness of the database schema interaction graph encoder.
NOTE: Video may display a random order of authors.
Correct author list is at the top of this page.
Connected Papers in EMNLP2020
Similar Papers
ENT-DESC: Entity Description Generation by Exploring Knowledge Graph
Liying Cheng, Dekun Wu, Lidong Bing, Yan Zhang, Zhanming Jie, Wei Lu, Luo Si,

Modeling Global and Local Node Contexts for Text Generation from Knowledge Graphs
Leonardo F. R. Ribeiro, Yue Zhang, Claire Gardent, Iryna Gurevych,

Re-examining the Role of Schema Linking in Text-to-SQL
Wenqiang Lei, Weixin Wang, Zhixin Ma, Tian Gan, Wei Lu, Min-Yen Kan, Tat-Seng Chua,
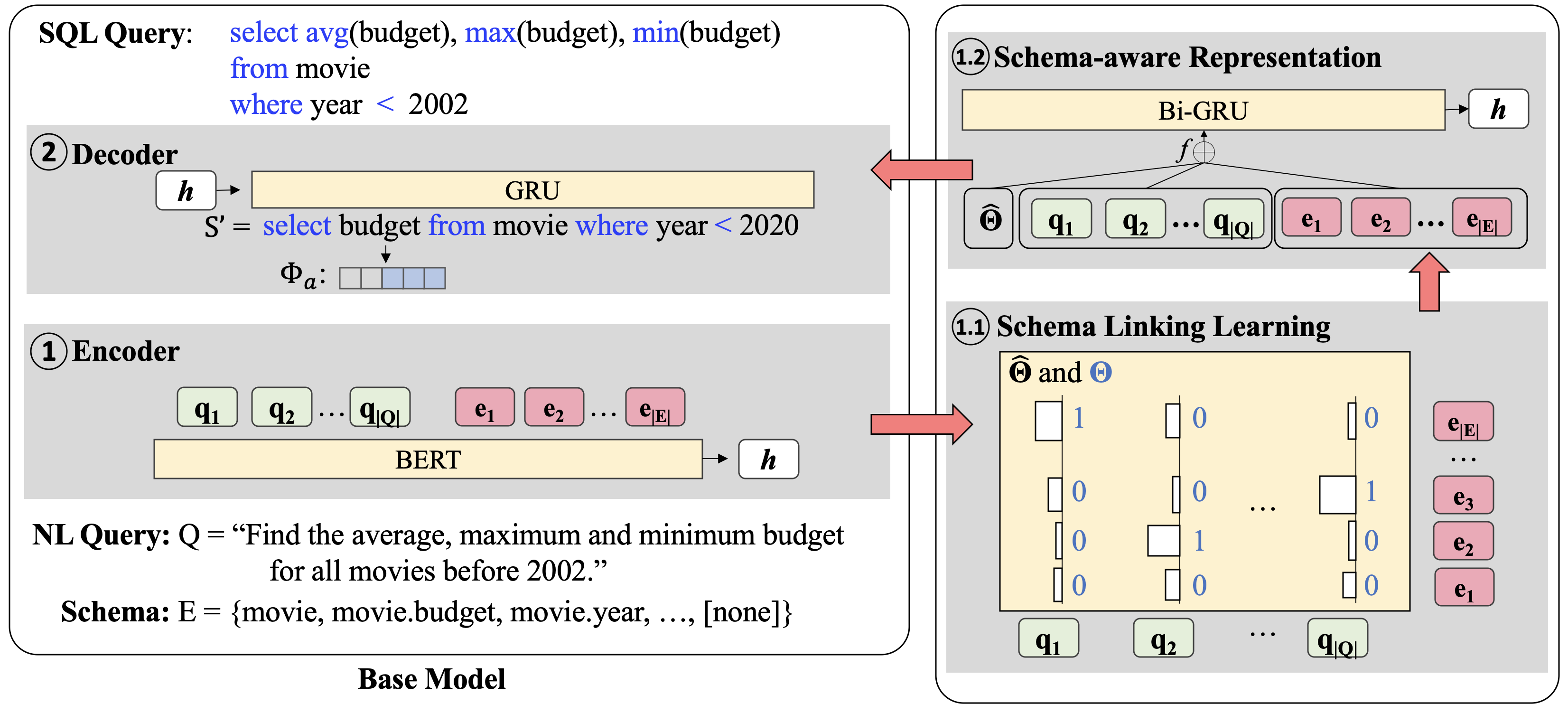