ENT-DESC: Entity Description Generation by Exploring Knowledge Graph
Liying Cheng, Dekun Wu, Lidong Bing, Yan Zhang, Zhanming Jie, Wei Lu, Luo Si
Language Generation Long Paper
You can open the pre-recorded video in a separate window.
Abstract:
Previous works on knowledge-to-text generation take as input a few RDF triples or key-value pairs conveying the knowledge of some entities to generate a natural language description. Existing datasets, such as WIKIBIO, WebNLG, and E2E, basically have a good alignment between an input triple/pair set and its output text. However, in practice, the input knowledge could be more than enough, since the output description may only cover the most significant knowledge. In this paper, we introduce a large-scale and challenging dataset to facilitate the study of such a practical scenario in KG-to-text. Our dataset involves retrieving abundant knowledge of various types of main entities from a large knowledge graph (KG), which makes the current graph-to-sequence models severely suffer from the problems of information loss and parameter explosion while generating the descriptions. We address these challenges by proposing a multi-graph structure that is able to represent the original graph information more comprehensively. Furthermore, we also incorporate aggregation methods that learn to extract the rich graph information. Extensive experiments demonstrate the effectiveness of our model architecture.
NOTE: Video may display a random order of authors.
Correct author list is at the top of this page.
Connected Papers in EMNLP2020
Similar Papers
An Unsupervised Joint System for Text Generation from Knowledge Graphs and Semantic Parsing
Martin Schmitt, Sahand Sharifzadeh, Volker Tresp, Hinrich Schütze,
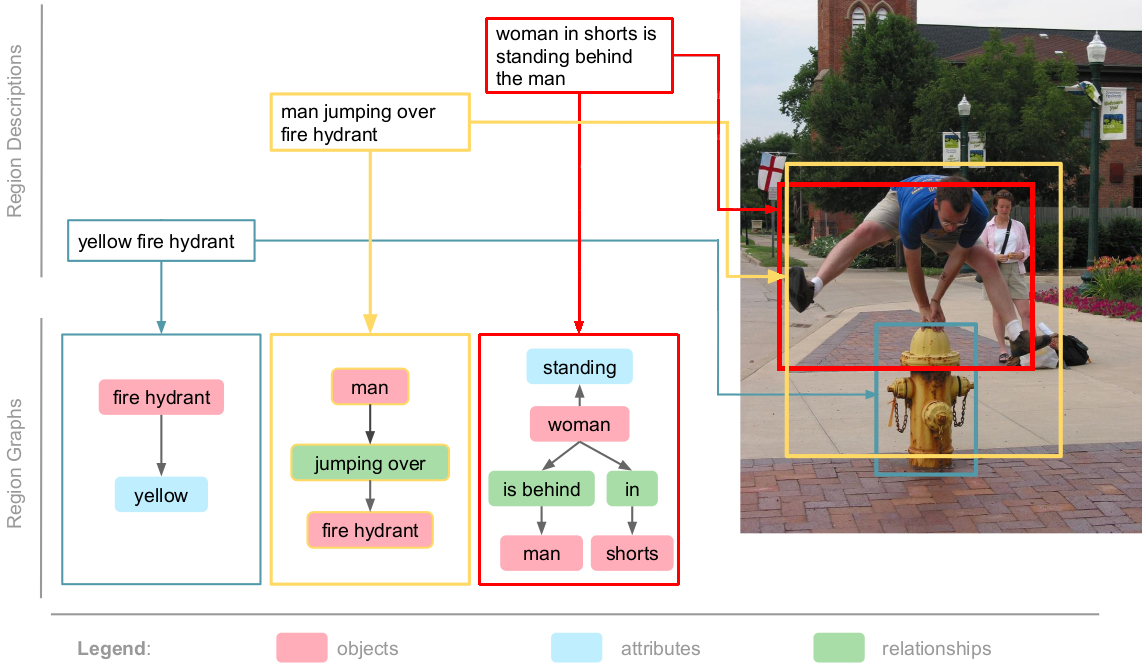
Double Graph Based Reasoning for Document-level Relation Extraction
Shuang Zeng, Runxin Xu, Baobao Chang, Lei Li,

Exploiting Structured Knowledge in Text via Graph-Guided Representation Learning
Tao Shen, Yi Mao, Pengcheng He, Guodong Long, Adam Trischler, Weizhu Chen,

Distilling Structured Knowledge for Text-Based Relational Reasoning
Jin Dong, Marc-Antoine Rondeau, William L. Hamilton,
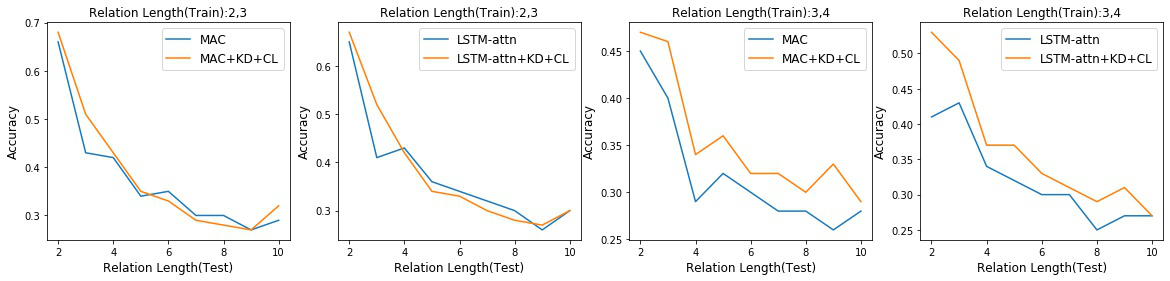