AmbigQA: Answering Ambiguous Open-domain Questions
Sewon Min, Julian Michael, Hannaneh Hajishirzi, Luke Zettlemoyer
Question Answering Long Paper
You can open the pre-recorded video in a separate window.
Abstract:
Ambiguity is inherent to open-domain question answering; especially when exploring new topics, it can be difficult to ask questions that have a single, unambiguous answer. In this paper, we introduce AmbigQA, a new open-domain question answering task which involves finding every plausible answer, and then rewriting the question for each one to resolve the ambiguity. To study this task, we construct AmbigNQ, a dataset covering 14,042 questions from NQ-open, an existing open-domain QA benchmark. We find that over half of the questions in NQ-open are ambiguous, with diverse sources of ambiguity such as event and entity references. We also present strong baseline models for AmbigQA which we show benefit from weakly supervised learning that incorporates NQ-open, strongly suggesting our new task and data will support significant future research effort. Our data and baselines are available at https://nlp.cs.washington.edu/ambigqa.
NOTE: Video may display a random order of authors.
Correct author list is at the top of this page.
Connected Papers in EMNLP2020
Similar Papers
Training Question Answering Models From Synthetic Data
Raul Puri, Ryan Spring, Mohammad Shoeybi, Mostofa Patwary, Bryan Catanzaro,
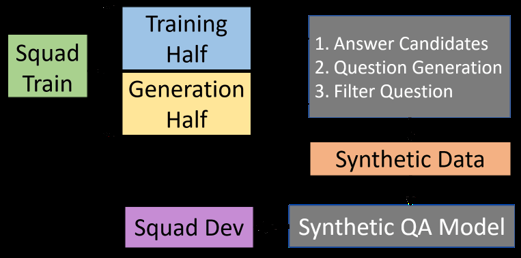
Inquisitive Question Generation for High Level Text Comprehension
Wei-Jen Ko, Te-yuan Chen, Yiyan Huang, Greg Durrett, Junyi Jessy Li,
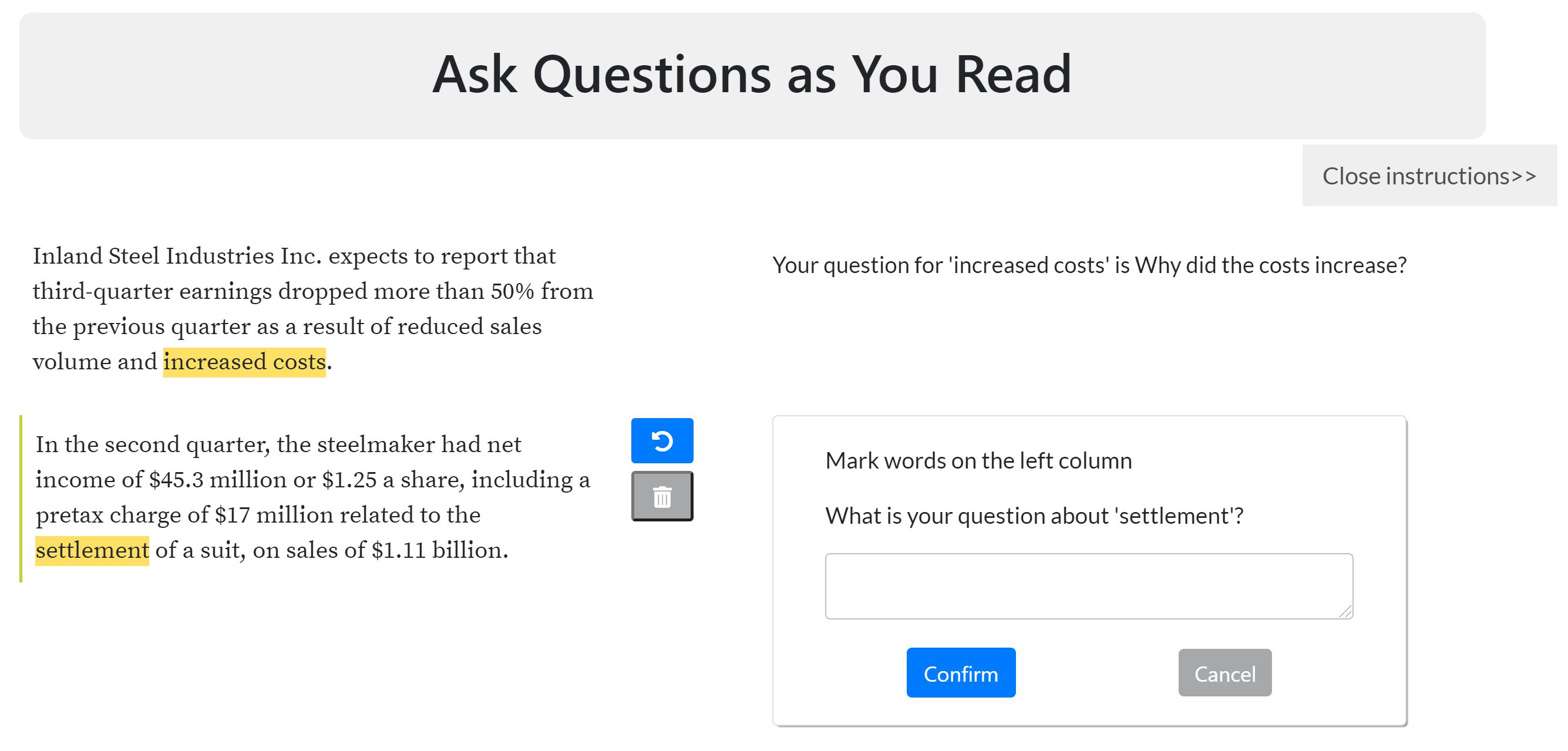
Dense Passage Retrieval for Open-Domain Question Answering
Vladimir Karpukhin, Barlas Oguz, Sewon Min, Patrick Lewis, Ledell Wu, Sergey Edunov, Danqi Chen, Wen-tau Yih,

What Does My QA Model Know? Devising Controlled Probes using Expert
Kyle Richardson, Ashish Sabharwal,
