Dense Passage Retrieval for Open-Domain Question Answering
Vladimir Karpukhin, Barlas Oguz, Sewon Min, Patrick Lewis, Ledell Wu, Sergey Edunov, Danqi Chen, Wen-tau Yih
Question Answering Long Paper
You can open the pre-recorded video in a separate window.
Abstract:
Open-domain question answering relies on efficient passage retrieval to select candidate contexts, where traditional sparse vector space models, such as TF-IDF or BM25, are the de facto method. In this work, we show that retrieval can be practically implemented using dense representations alone, where embeddings are learned from a small number of questions and passages by a simple dual-encoder framework. When evaluated on a wide range of open-domain QA datasets, our dense retriever outperforms a strong Lucene-BM25 system greatly by 9%-19% absolute in terms of top-20 passage retrieval accuracy, and helps our end-to-end QA system establish new state-of-the-art on multiple open-domain QA benchmarks.
NOTE: Video may display a random order of authors.
Correct author list is at the top of this page.
Connected Papers in EMNLP2020
Similar Papers
A Simple and Effective Model for Answering Multi-span Questions
Elad Segal, Avia Efrat, Mor Shoham, Amir Globerson, Jonathan Berant,

Context-Aware Answer Extraction in Question Answering
Yeon Seonwoo, Ji-Hoon Kim, Jung-Woo Ha, Alice Oh,
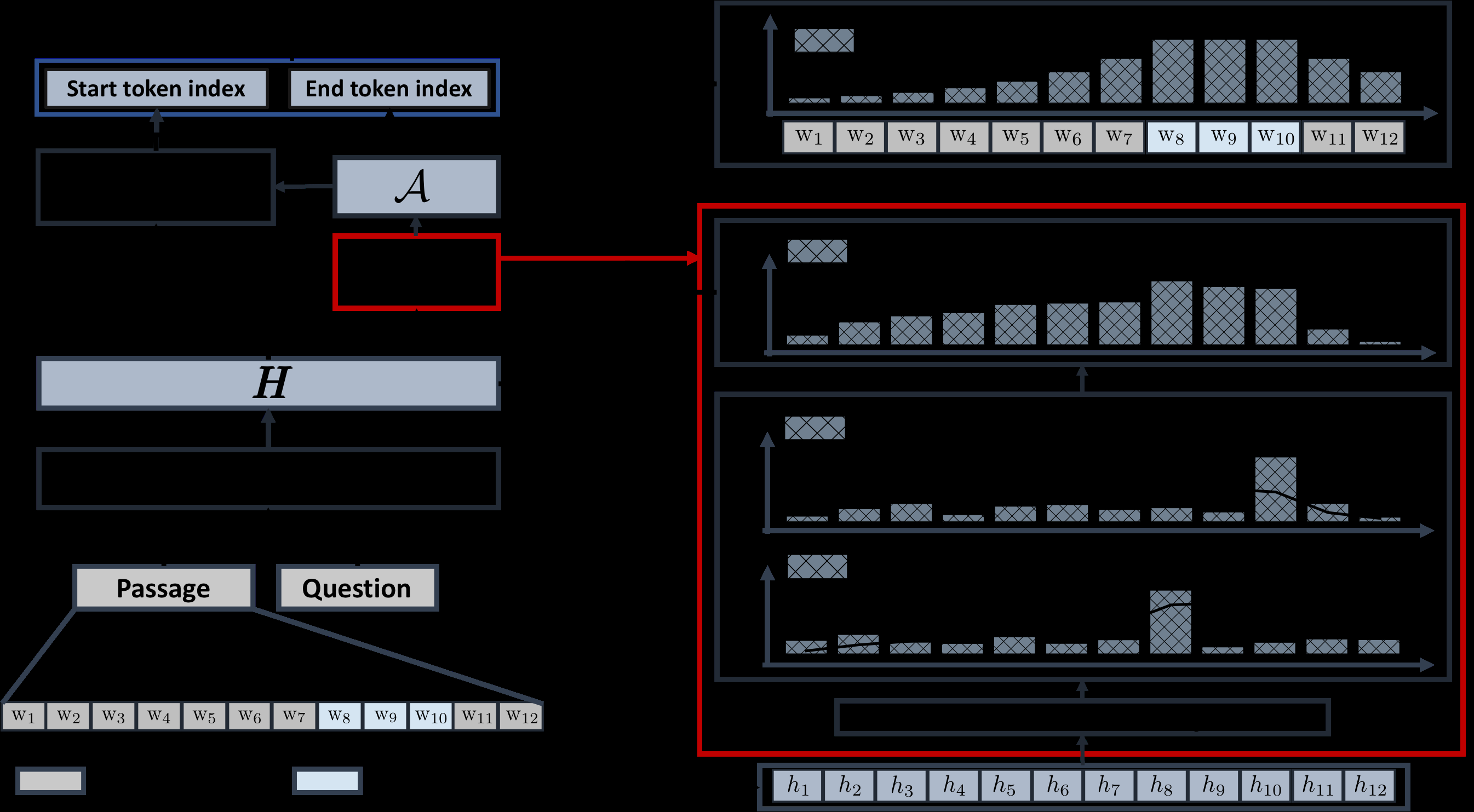
A Technical Question Answering System with Transfer Learning
Wenhao Yu, Lingfei Wu, Yu Deng, Ruchi Mahindru, Qingkai Zeng, Sinem Guven, Meng Jiang,
