Unsupervised Parsing via Constituency Tests
Steven Cao, Nikita Kitaev, Dan Klein
Syntax: Tagging, Chunking, and Parsing Long Paper
You can open the pre-recorded video in a separate window.
Abstract:
We propose a method for unsupervised parsing based on the linguistic notion of a constituency test. One type of constituency test involves modifying the sentence via some transformation (e.g. replacing the span with a pronoun) and then judging the result (e.g. checking if it is grammatical). Motivated by this idea, we design an unsupervised parser by specifying a set of transformations and using an unsupervised neural acceptability model to make grammaticality decisions. To produce a tree given a sentence, we score each span by aggregating its constituency test judgments, and we choose the binary tree with the highest total score. While this approach already achieves performance in the range of current methods, we further improve accuracy by fine-tuning the grammaticality model through a refinement procedure, where we alternate between improving the estimated trees and improving the grammaticality model. The refined model achieves 62.8 F1 on the Penn Treebank test set, an absolute improvement of 7.6 points over the previously best published result.
NOTE: Video may display a random order of authors.
Correct author list is at the top of this page.
Connected Papers in EMNLP2020
Similar Papers
On the Role of Supervision in Unsupervised Constituency Parsing
Haoyue Shi, Karen Livescu, Kevin Gimpel,

Investigating representations of verb bias in neural language models
Robert Hawkins, Takateru Yamakoshi, Thomas Griffiths, Adele Goldberg,

Automatic Extraction of Rules Governing Morphological Agreement
Aditi Chaudhary, Antonios Anastasopoulos, Adithya Pratapa, David R. Mortensen, Zaid Sheikh, Yulia Tsvetkov, Graham Neubig,
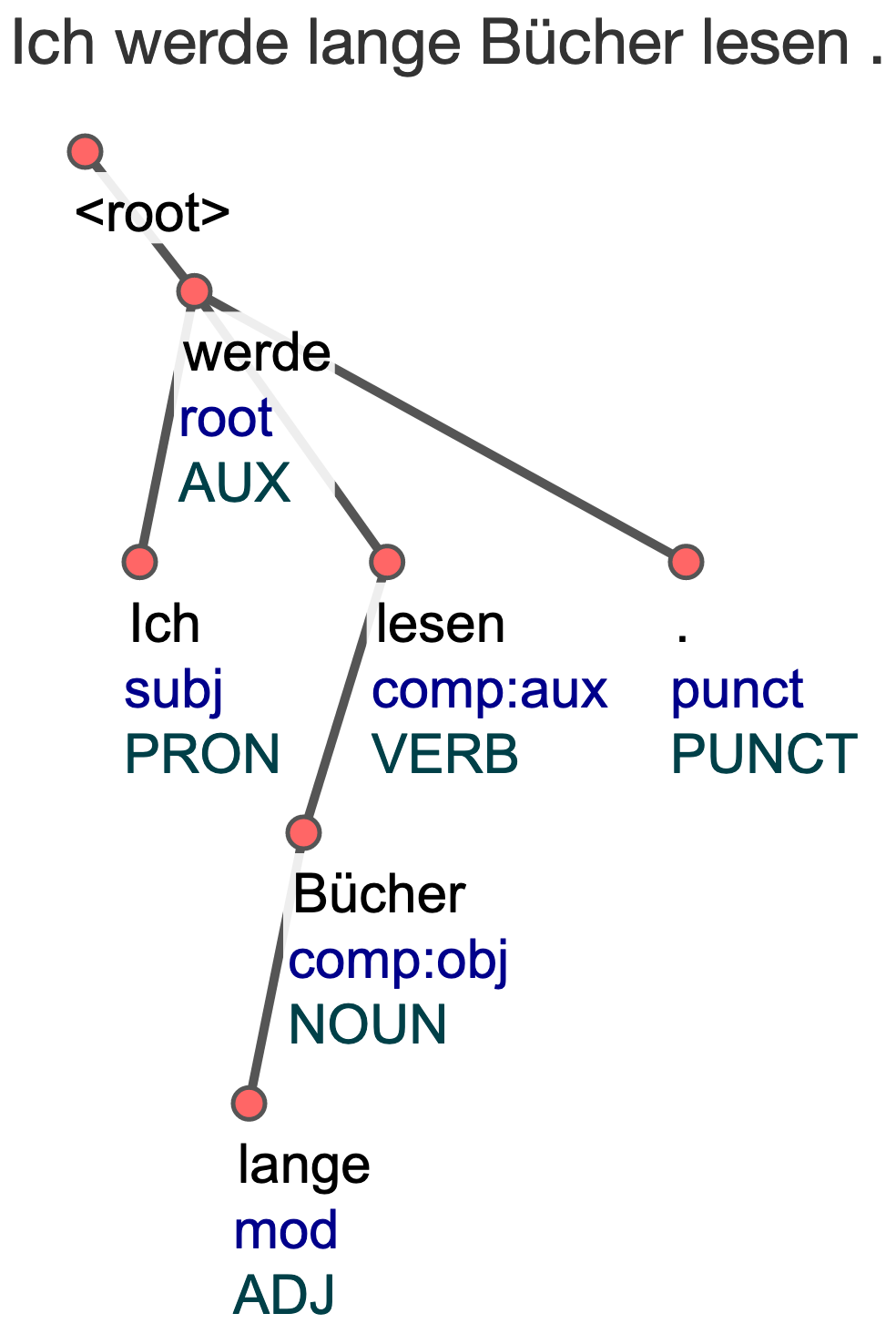